Stream Processing with InfluxDB
Process continuous streams of large volumes of data to detect conditions and anomalies in an instant, inspect results, and analyze data from multiple streams simultaneously.
Get Started Now
What is stream processing?
Stream processing is the processing of data in motion. It’s big data technology used to query continuous streams of incoming data and detect conditions quickly.
Why InfluxDB for stream processing?
Stream processing unifies applications and analytics by processing data as it arrives in real time. Its key strength is that it can provide insights faster, often within milliseconds to seconds. That said, stream processing naturally fits with time series data, as most continuous data series are time series data. Time series data needs a purpose-built database to ingest, store, and process it. This is exactly what InfluxDB is. This is why, given its high-write throughput and the scalability it allows, InfluxDB suits stream processing.
Components of the InfluxData Platform
InfluxDB
InfluxDB is a time series database designed to handle high-write and query loads. It is an integral component of the TICK Stack. InfluxDB is meant to be used as a backing store for any use case involving large amounts of time-stamped data, including DevOps monitoring, application metrics, IoT sensor data, and real-time analytics.
Kapacitor
Kapacitor is an open source data processing framework that makes it easy to create alerts, run ETL jobs and detect anomalies. Kapacitor is the final piece of the TICK Stack.
Related resources for developers
InfluxDB Integrations
Free InfluxDB training
Get Blueprint
“Why InfluxDB? For Playtech, it was very important to have observability, to understand system behavior to predict possible outages and problems in the very early stages.”
Aleksandr TavgenPerformance and innovation with open standards
We built InfluxDB 3.0 in Rust using the FDAP stack
Parquet
Open column-oriented file format designed for efficient data storage and retrieval
Learn More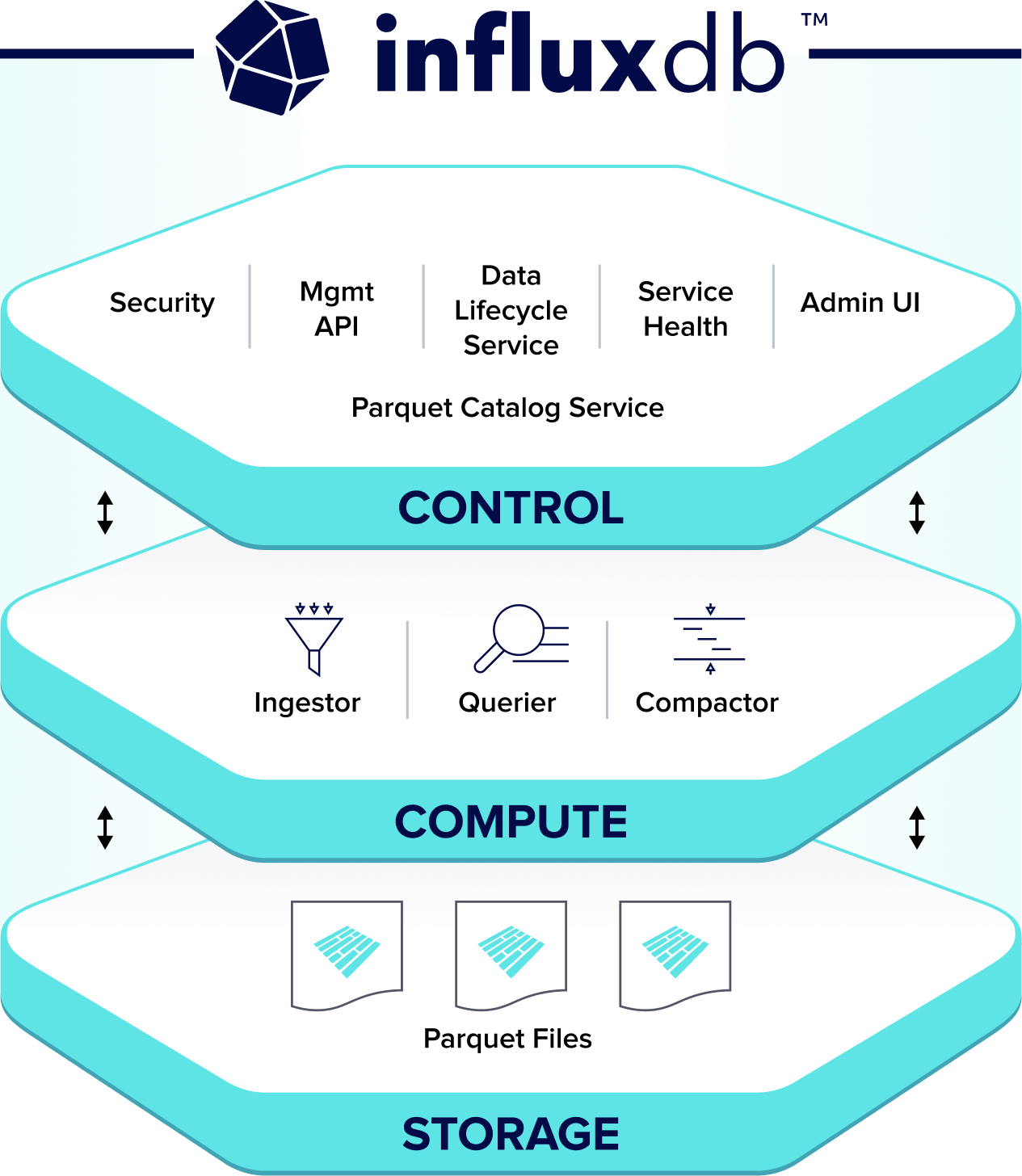
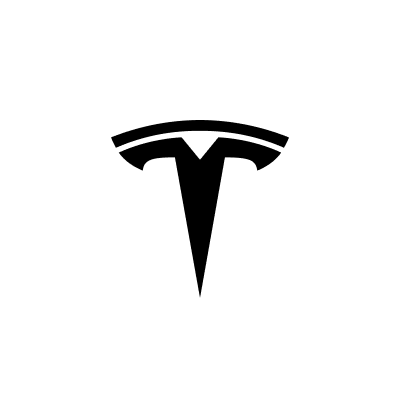
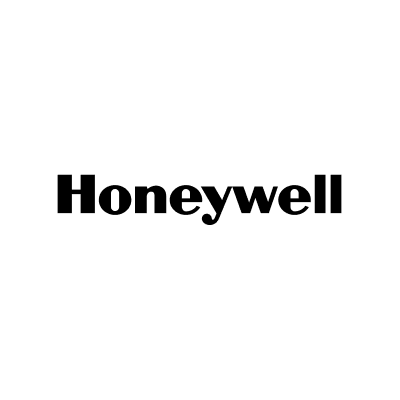
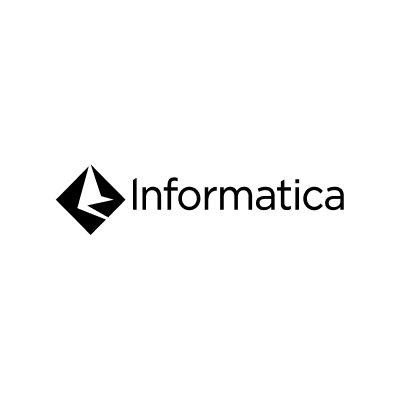
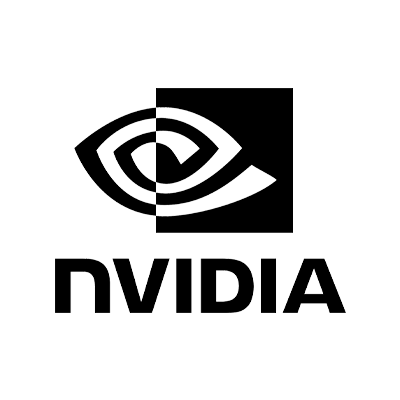
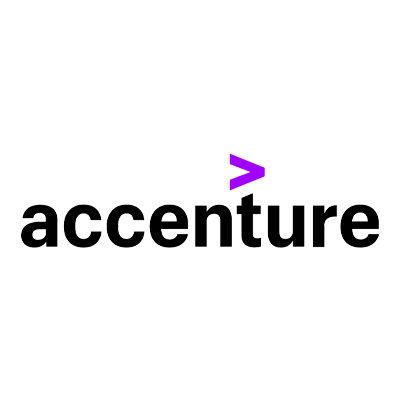
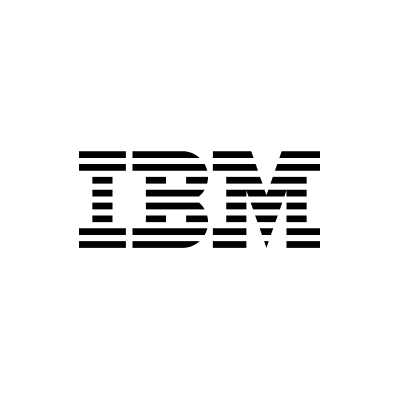
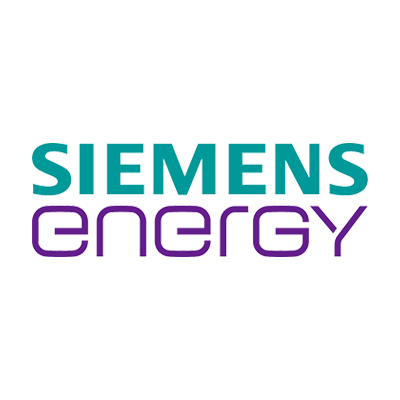
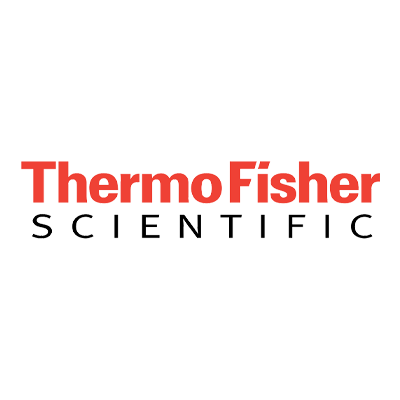
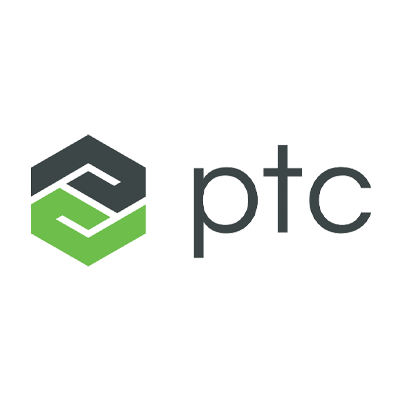
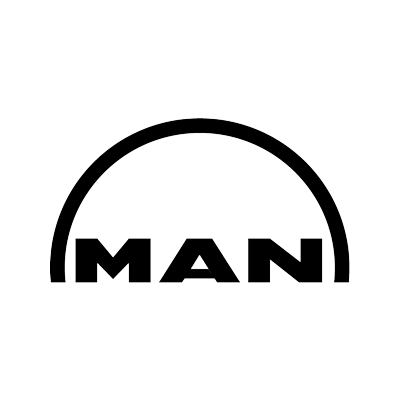
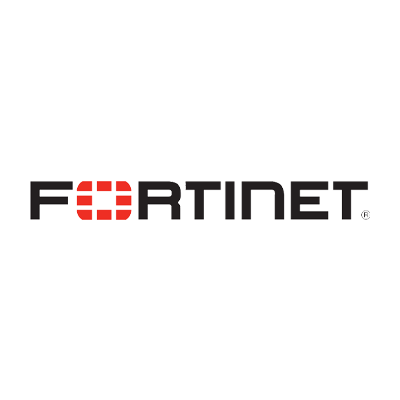
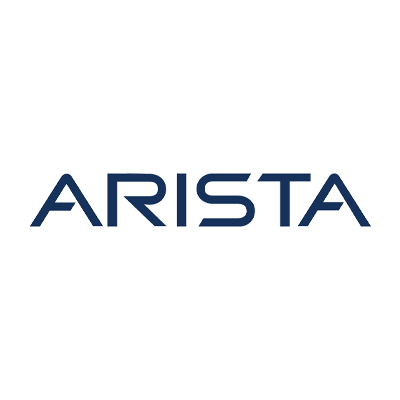
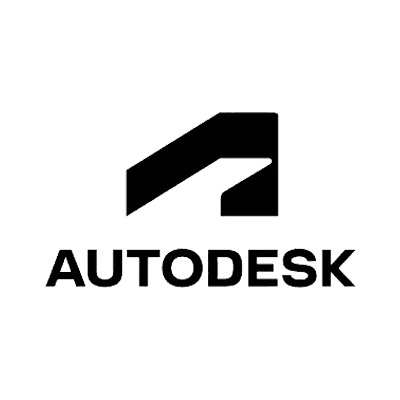
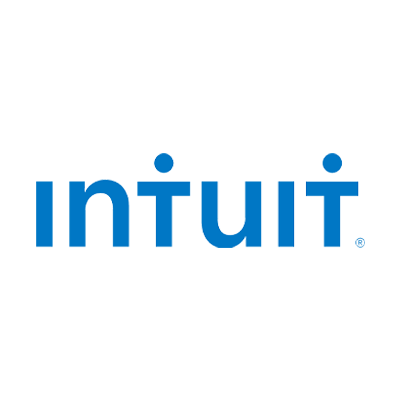
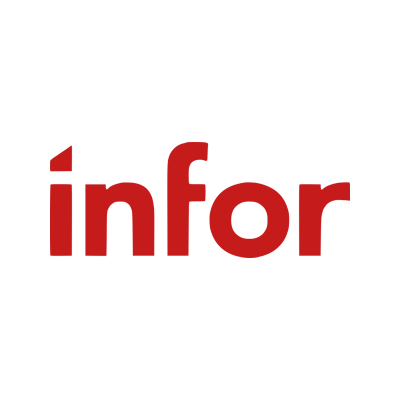
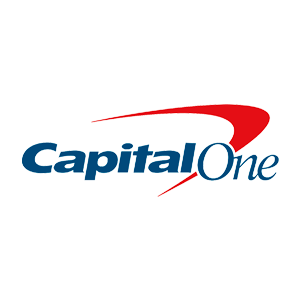
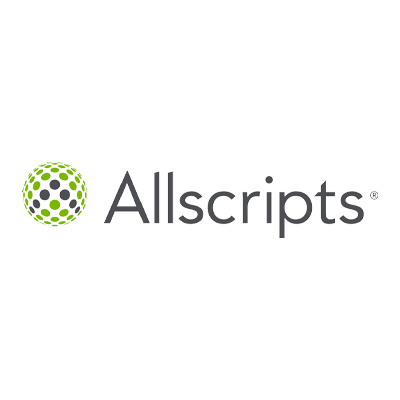
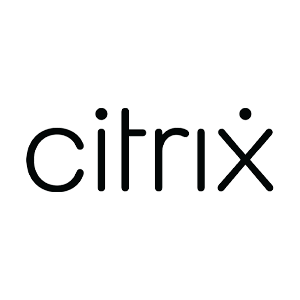
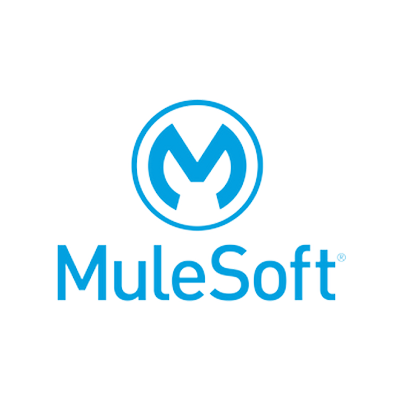