Consumer IoT
The consumer IoT solution using InfluxDB provides customers with insights and analytics into devices—collecting, storing, and visualizing sensor data.
Get Started Now
Why a purpose-built time series database?
Sensor data is all time-stamped to help you understand how your processes and equipment are performing over time to help further innovation and improvement.
Why InfluxDB for consumer IoT?
Organizations are keen on providing their end customers with real-time access to the data being collected through their websites and mobile apps. People are using the time-stamped data to reduce energy consumption, improve sustainability practices, and save money.
Monitoring and tracking
Tracking battery performance, turbine production, package delivery status, and generally visually monitoring sensor data provides incredible capabilities to businesses and their customers. This is usually the first step in any successful IoT project.
Analytics
Using historical data from sensors to gain insights that can be applied to the current situation creates a major competitive advantage. Predictive maintenance, optimized traffic routing, reduced churn management, and enhanced water conservation are all possible with IoT analytics.
Action and control
With sensors generating the speed and velocity of events, businesses want to act on this data in real time without human interaction. For example, automatically shutting down a pump if a leak is detected or changing wind turbine direction based on forecasted wind speed all create immediate business advantages.
Related resources for developers
InfluxDB Integrations
Free InfluxDB training
“You can design your database in several different ways. It’s just a matter of trying and seeing which one works best for you.”
Dr. Angelo FaustiSoftware Engineer, Vera C. Rubin Observatory
Performance and innovation with open standards
We built InfluxDB 3.0 in Rust using the FDAP stack
Parquet
Open column-oriented file format designed for efficient data storage and retrieval
Learn More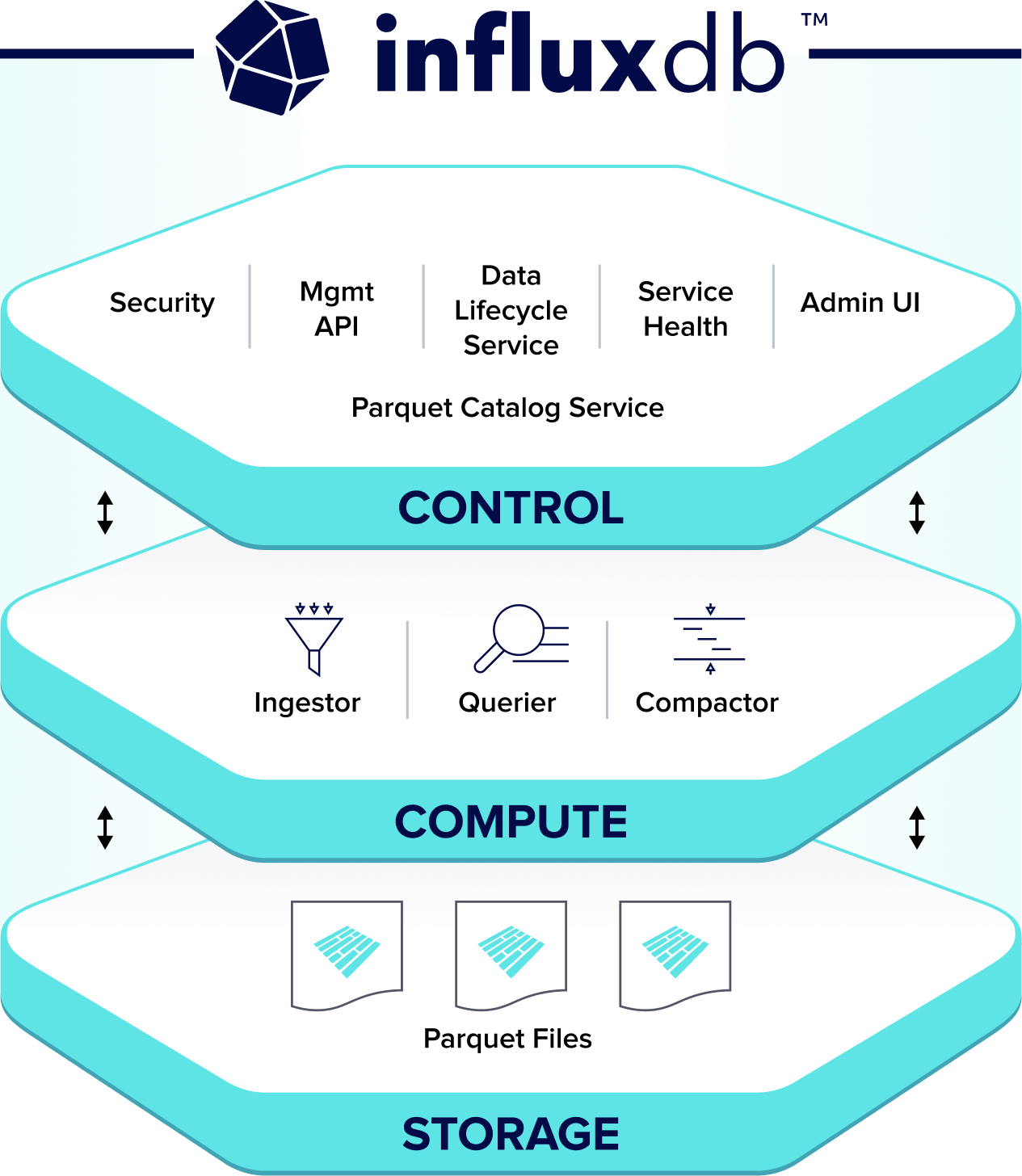
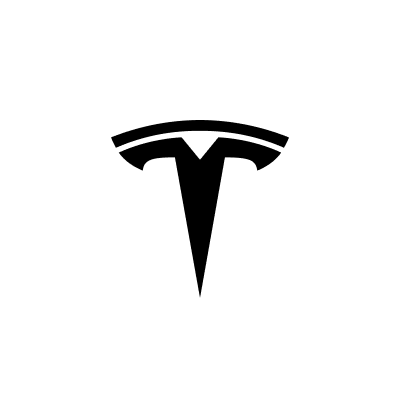
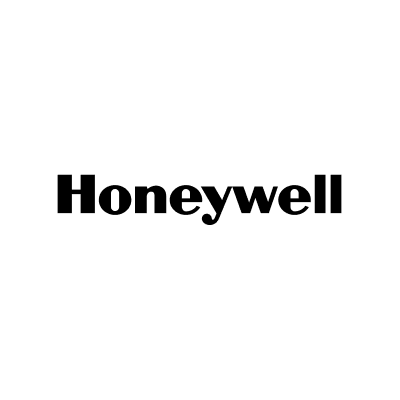
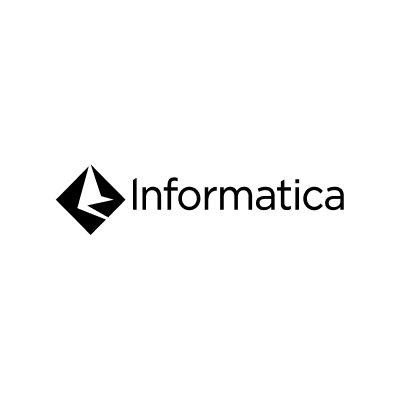
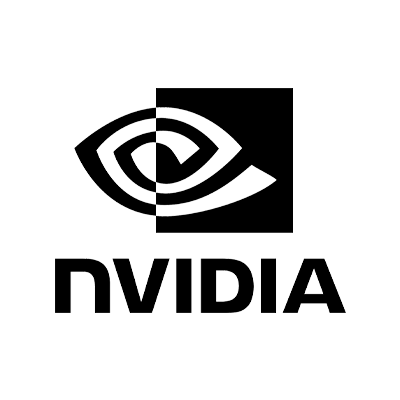
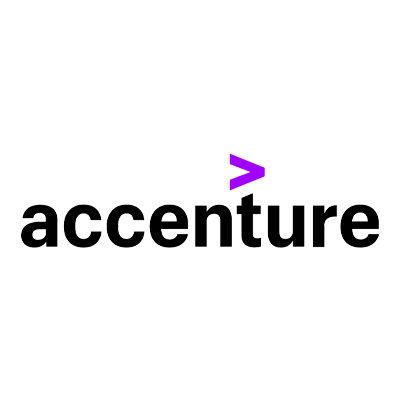
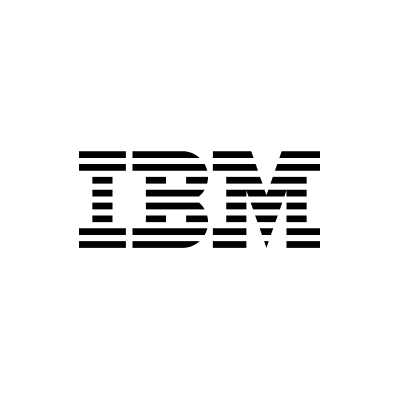
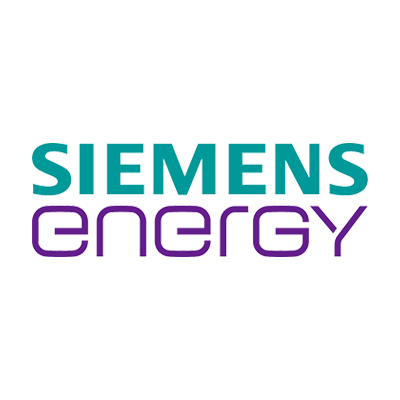
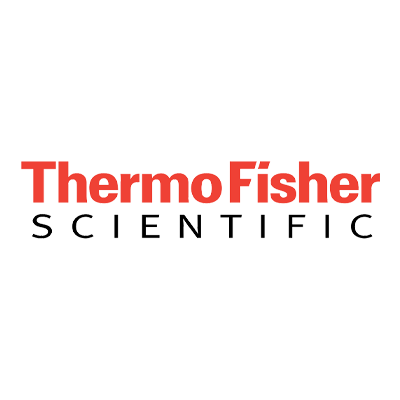
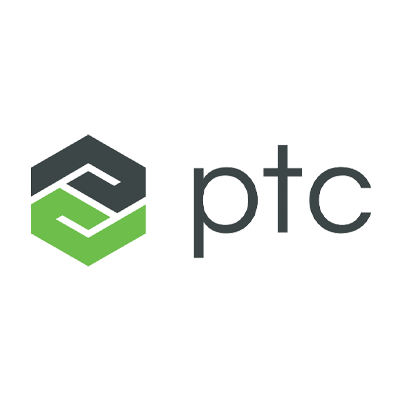
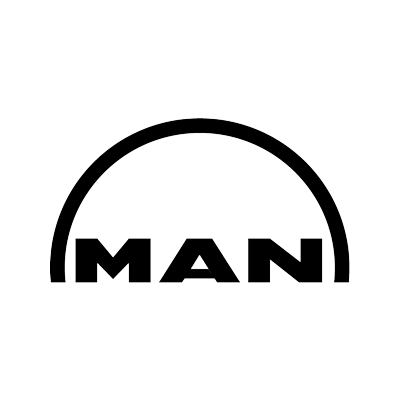
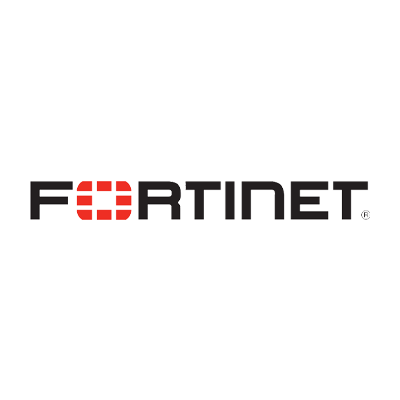
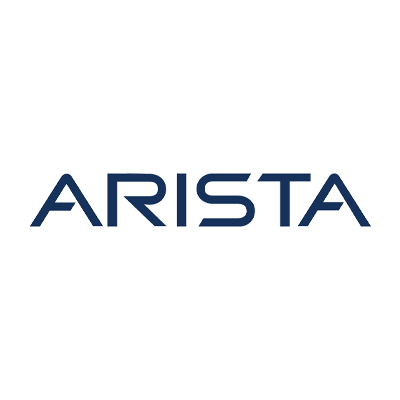
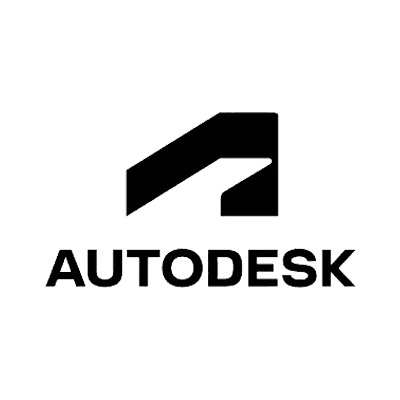
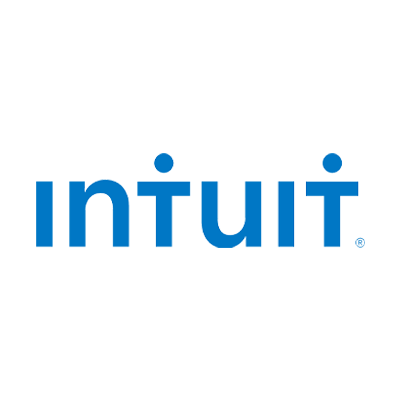
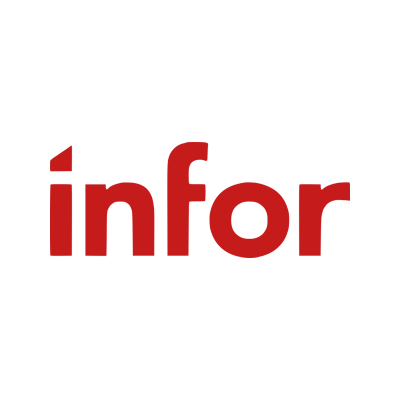
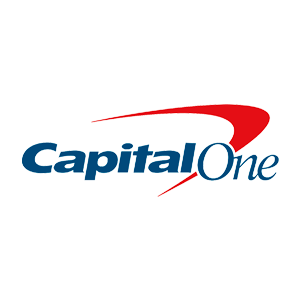
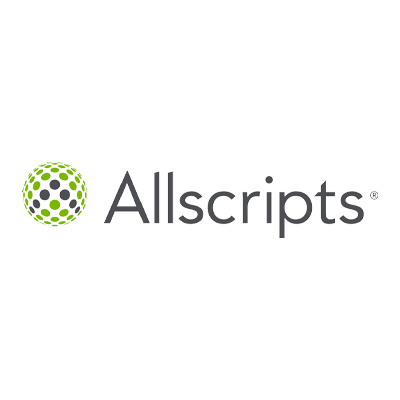
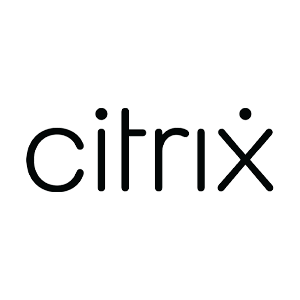
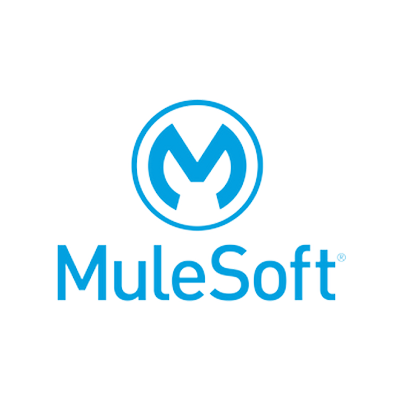