Discover How Allscripts Uses InfluxDB to Monitor Its Healthcare IT Platform
Session date: Nov 03, 2020 08:00am (Pacific Time)
Allscripts is an industry leader in electronic health record (EHR) system integration and healthcare information technology. Its platform is used to help healthcare organizations drive better patient care, improve financial and operational outcomes and advance clinical results. Its solution connects healthcare professionals with data across the open platform. Allscripts uses a time series database to become data-driven by gaining observability into its platform to help healthcare organizations maximize application availability.
Join this webinar to learn about:
- Allscripts effect on healthcare delivery
- Its DevOps approach that has improved service uptime
- How InfluxDB enables better data correlation and reporting
Watch the Webinar
Watch the webinar “Discover How Allscripts Uses InfluxDB to Monitor Its Healthcare IT Platform” by filling out the form and clicking on the Watch Webinar button on the right. This will open the recording.
[et_pb_toggle _builder_version=”3.17.6” title=”Transcript” title_font_size=”26” border_width_all=”0px” border_width_bottom=”1px” module_class=”transcript-toggle” closed_toggle_background_color=”rgba(255,255,255,0)”]
Here is an unedited transcript of the webinar “Discover How Allscripts Uses InfluxDB to Monitor Its Healthcare IT Platform”. This is provided for those who prefer to read than watch the webinar. Please note that the transcript is raw. We apologize for any transcribing errors.
Speakers:
-
-
- Caitlin Croft: Customer Marketing Manager, InfluxData
- Mike Montano: Sr. Manager, Allscripts
- Chris Ruscio: Solutions Architect, Allscripts
-
Caitlin Croft: 00:00:04.365 Hello, everyone. Once again, welcome to today’s webinar. My name is Caitlin Croft, and I’m super excited to be joined today by the Allscripts team. So they’re here to talk about how they’re using InfluxDB to monitor their healthcare platform. So without further ado, I’m going to hand it off to Mike and Chris.
Mike Montano: 00:00:31.345 Thank you, Caitlin. Appreciate that. All right. So as Caitlin mentioned, we are from Allscripts. We’re going to talk about the IT monitoring solution that we work together to design and how we’re using InfluxDB to monitor the health IT platform. The agenda for today is we’re going to do a quick intro. We’ll talk about the Allscripts company overview so you understand who we are and what we’re about. Kind of the main problem that we have, we’re going to explain why we came to the conclusion that we needed something like InfluxDB. And also we’ll talk about in-depth the solution requirements and overview of that solution. And then we’ll end on outcomes and future projects, lessons learned and then Q&A. So I am Mike Montano. I’m a senior technical support manager and IT manager for Allscripts. I manage an operations and development team here delivering application-monitoring solutions for hospitals and health systems across the US. We are an international company. We do have global accounts and things like that. But the majority of the accounts that we monitor are in the United States. I’ve been in IT for 25 years, 20 of those years in IT support and service delivery and 18 of those specifically with Allscripts’ commercial and custom-developed application-monitoring solutions and services. Chris?
Chris Ruscio: 00:02:02.423 I’m Chris. I’m a solutions architect here at Allscripts. I’ve been doing that for five years now. Also performed other roles here at the company for over a decade. I think I’m going on year 13 now. I happen to be a strong advocate for the adoption and contribution back to open source projects. And there are people much smarter than me that have solved the same problems that we’re facing today. And I’m glad for the opportunity to benefit from their experience and to pay that forward by contributing back what I can and build a community solution here just like the Influx open source projects have done.
Mike Montano: 00:02:36.521 All right. Thank you. All right. So before we talk about the problem and the solution and what we came up with, I wanted to discuss real quick about the global healthcare crisis experience. We are experiencing unprecedented challenges in many industries. Healthcare industry was highly impacted. Issues with not having enough personal protection equipment, the need to socially distance, and in some cases occupations that were meant to be in-person, on-location now become possible opportunities or virtually. All sorts of measures being taken to slow down the spread of COVID-19 and impacts. Even before the challenges brought by the global pandemic, keeping critical healthcare systems running and optimally performing was always a key focus for Allscripts so systems and applications are used to capture and track vital health information so care providers can deliver healthcare service, create and send electronic prescriptions, support business and financial operations. It’s never been more important now to keep critical healthcare systems available, optimized, and high-performing to avoid impacting patient care and business operations. A simple interface delay [laughter] in our area can mean patient care is impacted, medical treatment delayed, prescriptions delayed, or there’s negative consequences to providing patient care. So today, we’ll be talking about some of the operational challenges that we have with monitoring healthcare systems at Allscripts and also what we’ve done to meet and overcome these challenges using Influx data for a long-term strategy.
Mike Montano: 00:04:13.645 So who is Allscripts? Allscripts is a software solutions and services company for the healthcare industry. We provide innovative EHR, financial and operational management, population health management, patient engagement, precision medicine, and payer and life sciences solutions to our customers. We have industry-leading electronic health records, management systems that meet and exceed the needs of today’s healthcare environment from independent practices to community hospitals to large health systems and everything in between. Also helping you deliver smarter care, special [inaudible] workflows are built into our solutions that help care teams provide the greatest support for optimal care and outcomes. Actionable information at the point of care with solutions like our Baby Motion and FollowMyHealth applications.
Mike Montano: 00:05:10.887 Our solutions also have intuitive workflows for ease of use. Allscripts has actually won awards for its usability, top ranking, and user-centered design based on framework developed by the AMA and MedStar’s Health National Center for Human Factors in Healthcare. Also, from care coordination standpoint, we have solutions, complete single-patient records to help providers make care decisions based on that information, enable communication and collaboration in ways never before available and also reducing gaps in care and enable better management of individuals and populations, especially important during COVID. These are some of the words to kind of illustrate: Allscripts has become known for providing top-performing and highly usable solutions in hospital and health system space, in ambulatory setting, in organizations large and small, and also in the clinical care, financial management, and operational support. We are an industry leader and an innovator.
Mike Montano: 00:06:17.869 So this brings us to the part where we start talking about the operational challenges we face with having so many of the solutions out there. This picture depicts the scope of an Allscripts solution or service being used. This is generally showing the United States. But as I mentioned, we are a global - we have a global presence. This is to show the magnitude of the problem, specifically with ensuring that Allscripts solutions are monitored, available, high-performing, and we do this through IT and application-monitoring tools. However, as we’ll share with you, what the scope brings about all the different challenges that we have. So this really sums up the problem. Through various means, acquisition of companies where we have solutions that we’re trying to include into our portfolio, over the years, additional systems and solutions in place, and those companies have been acquired also have their monitoring solutions, their data centers, and things like that. So as we become one Allscripts, we have these different challenges, different IT systems across the entire organization and in the world, essentially. So as you can see from this slide, the tremendous variety of challenges, multiple data centers, self-hosted environments, third-party data centers, cloud solutions, third-party solutions, actual solutions that work with our applications and our solutions, and just a plethora of commercial enterprise-monitoring tools and in-house developed IT application performance-monitoring tools.
Mike Montano: 00:07:57.138 Some of the tools were bought over through the acquisition, as I mentioned, gaps in healthcare solutions, and were filling to meet customer needs and expand our solution portfolio. That also in turn brings about a lot of overlap in the capability, so that’s creating scope issues, tremendous cost issues when we’re having things - monitoring the same solutions or systems and the overlapping capabilities is just not very cost-effective. So needless to say, that creates all sorts of operational challenges to manage or maintain these various monitoring solutions, not to mention different alerting methods for each tool. Sometimes, we have different tools. They have different capabilities and they work differently. They have different APIs and that sort of thing. And also, the same thing happens with the visualization of those capabilities in that area. So all of these challenges affect the support and service delivery for our customers. That brought to us the question: how do we create a unified monitoring platform that gives us the base IT system and application-monitoring capability that we need but also while having the ability to only use specific commercial monitoring tools when necessary? So there might be situations where we want a platform across the board, but there might be in some places where we actually need a commercial monitoring tool to do X, Y, or Z. And so that is also becoming a challenge to kind of integrate these things together and have them all working in conjunction with one another. That would drastically reduce our monitoring software footprint by doing that scope and cost and allow us to leverage a more powerful platform like that to consolidate a learning and visualization capability.
Mike Montano: 00:09:44.383 So what we did was we put our heads together. I remember Chris and I and some other key personnel in this area met years ago to try to come up with a solution, and a lot of things are thrown on the board, other commercial solutions to take over everything. Open source came up. Chris is an advocate for that. And so we came across all these different ideas and things like that. And what we came to are the kind of key solution requirements. So the first is being flexible. We have to have a unified monitoring system that looks at any IT system, Allscripts solutions and allow for customer development. That’s a key piece because we are a development team. We have situations where we need to do something custom. We have to be able to do that without having to go back to the vendor and have them build it for us.
Mike Montano: 00:10:37.128 Secondly, economical. To replace all the costly commercial tools that we have that are overlapping and leverage the solution to monetize for new service offerings. As I mentioned before, kind of in the situation in the place to provide service offerings to clients, we want to be able to generate new service revenue opportunities and using the tools to do that. Third was it needed to be adaptable. So we have to have the ability to monitor any environment as I showed you in that problem, hosted, self-hosted third-party cloud hybrid. We do have situations where we have a customer who’s one of our customers but also a hybrid. They have another solution from another vendor, and they are also managed in another data center. So there are situations like that. And also, we needed it so that it was adaptable to the rapid changing technologies.
Mike Montano: 00:11:32.059 Another one was being scalable. We have to be able - so as a monitoring operations team, we have to be able to deploy a solution out quickly. We can’t have weeks down. We can’t have months of planning and projects and things like that. It needs to be very quick, extreme scalability, and be able to implement quickly through orchestration. Our customers are hospitals and health systems. They do not have hours in time and resources to spend working with you on a project for weeks or months. And some additional, these are not least of the key requirements, but these are additional solution [inaudible]. Very, very important that these are in here. The first was on these additional as a zero downtime, as I mentioned, these are health systems. They have to be at 24/7. So we can’t have any kind of solution in there that we have to do an installation and it causes the system to go down. It’s not possible. So we have to meet that demand for our customers.
Mike Montano: 00:12:33.408 The next thing, also very important piece of it, especially now with ransomware and things like that going on, and recently there was some news come out that hospital systems are very susceptible and being attacked. Right as we’re probably talking about this right now, they’re being attacked constantly. So we have to meet the customers’ Allscripts HIT and in some cases government regional security requirements. We can’t have a solution out there that is susceptible to ransomware attacks and things like that, so we’ve got to be very careful with that. It has to be modular, swap out modules without affecting entire monitoring solutions. Very key. If we have something that, say, part of the solution or vendor we’re using no longer exists, we have to be able to be able to plug something in there that’s modular with it that’ll work. And in an open source area, that seems like a very doable solution, so. It needs to be modular and then probably one of the most in terms of from a management perspective, I’ve really used reports and dashboards and that data that’s coming from these solutions. And we have to be able to do that from a centralized, accessible, has to be available for everybody, all the managers, all the VPs and everything. And then it needs to be very robust, reporting and dashboards that we can get to easily and be able to see what’s going on out in our environments. So that was the solution requirements. And now we’re going to get into the solution overview which I’m going to hand over to Chris Ruscio to explain.
Chris Ruscio: 00:14:09.186 Thanks, Mike. So as we looked at the requirements in the current tools, we decided to focus on monitoring of operating systems and application service health specifically. Infrastructure monitoring and APM distributed tracing were out of scope. And as Mike mentioned, we still look at some commercial tools for special areas and those are those areas. We use LogicMonitor for some of our infrastructure monitoring and AppDynamics for some of our APM. So as we were looking at the application service health, the problem began to shape into three key areas: configuration management, log analytics, and telemetry. And our first choice was to expand one end-use tool or find a single replacement to meet all of those requirements. And we looked at a long list of things. Among others, there was LogicMonitor, Splunk, Datadog, eG Innovations, Nagios, Sensu, ServiceNow, Operations Intelligence, and I can’t tell you how many different Azure are offering.
Chris Ruscio: 00:15:05.787 We even looked at a few [inaudible], their feature sets, especially as we were in our early days of alert management. Their feature sets very closely align with some of our requirements. But none of that really fit our needs. There were either technical limitations managing them at scale or limited data fidelity and retention issues for some of the hosted services or just prohibitive costs as we started to scale up to the scope that we were looking at. And so to achieve our goals, we started focusing on a combination of a collection of open source services. And it was important that each one of those, as Mike mentioned, be modular, scalable - and also important that they had a very strong community backing and a commercial service offering where we found ourselves needing some help. If you could get hit the next slide. So starting off here, we started off with telemetry. And I mean, that’s why we’re all here. So let’s feature that one first. Why did we choose InfluxDB? We knew that we needed a purpose-built time series database to be able to handle the volume of data we were looking to collect and process. And so many of the solutions that have metrics as a bolt-on, so for example, Elasticsearch, just would not scale to the size that we needed. We narrowed it down to InfluxDB, TimescaleDB, and Prometheus.
Chris Ruscio: 00:16:26.737 And Timescale looked promising. It’s built on PostgreSQL. It has an unshakable foundation there. But at the time, it was still pre-1.0 and its future was much less than certain. It also still to this day lacks an alerting story, and it chooses to recommend Grafana for alerting instead. And the other issue we had with it was that its relational schema data model is just much more rigid than the InfluxDB tag-set model. And there’s value there. It keeps you from running away on your cardinality. But at the same time, it slows down your ability to push out new data collection. So Prometheus was easily at the time the foremost InfluxDB competitor, I think. However, its scaling model just wasn’t well suited to our deployment and security requirements. And it didn’t scale well. CentOS and other add-ons were beginning to appear back then, but they were just that. They were add-ons, not maintained as a core feature of Prometheus itself. And so at the end of the day InfluxDB provided a horizontally-scalable, time series-optimized database with a lightweight agent that could be deployed anywhere we needed to push data to a central location. And we can rapidly modify tag fields or measures without needing to juggle table schemas or any other changes first. Okay, the next slide, please.
Chris Ruscio: 00:17:56.392 So the other two primary components to our platform are configuration management and log analytics. SaltStack with our first choice to centrally manage configuration of deployed monitoring agents, and as the platform matures, we intend to grow that into managing configuration of installed business services as well, not just our monitoring components. For a single data center, we would’ve probably chosen Ansible. We just couldn’t operate it efficiently across multiple data centers, many of which are Windows-only with no easy way to deploy a local Ansible cluster. Chef and Puppet were the more popular CM tools at the time. But there were concerns about conflicting with existing installs, and we didn’t have the Ruby experience in-house to extend them the way we could solve through Ansible and Python. And we also looked at SCOM/OMS/Azure Automation/Lighthouse/whatever Microsoft is calling it today, which could multihome agents very well. But it’s much more of a black box, and it’s great when things worked. But we’ve been burned by it more than a few times.
Chris Ruscio: 00:19:01.592 On the log analytics side, I don’t have quite as much to say other than, “Keep it simple.” Elasticsearch, the ubiquitous answer, we did take a little bit of a look at Grayscale Solar and a few others. Elastic was well-established. Running Grayscale, we would’ve been running Elastic anyway, so that would’ve been more complexity there. And Elastic could scale easily and came with Beats agents that we needed to facilitate our push model, so it just seemed to check all the boxes. Next slide, please. So for visualization and alerting, we needed to figure out what to do with all of the data that we collected and we needed to see it in action. And we went in two different directions here. For visualization, we chose Grafana. We wanted a single interface that could merge multiple data sources into one UI, one holistic view, and one design language for any user to build their own views across all available data. We manage a mix of curated lockdown dashboards and an instance of open access dashboards which enable teams to modify and design to suit their bespoke needs.
Chris Ruscio: 00:20:11.903 For alerting, we went the other direction. We chose to implement the native services in Influx and Elastic ecosystems. Our alert developers are a smaller group. The cost of learning to work with multiple interfaces is worth the features and performance optimization we get from using those tools. The streaming success there, we can process data far more efficiently in TICKscripts into Influx than with Grafana alerting. On the flip side, we’ve done little with Elasticsearch alerting, and we’re still deciding between Elasticsearch and Open Distro, but we are going to stick with one of those two and we are going to use the native alerting in one of those two platforms. Today, we primarily use Elastic for ad hoc querying across aggregated data sources, less so for actioning alerts. Hit the next slide, please. So what does that all look like? We have an Azure tenant running an 8-node InfluxDB cluster with three metanodes, a 10 data node Elasticsearch cluster with, I think, 8 other nodes between the ingest [inaudible] and master nodes and a seven-broker Kafka cluster.
Chris Ruscio: 00:21:26.267 All of our Azure resources were created via Terraform and configured via SaltStack. Data are ingested via the internet-facing Kafka cluster through an F5 BIG-IP appliance, maintained by our network perimeter security team. Kafka provides us a single Ingress surface and a message buffer in the event of either planned or [laughter], more than I care to admit, unplanned cluster maintenance. And on the client side, we bootstrap a SaltStack minion agent on every server, and those agents provide a central control of our entire deployed footprint in any data center. They then deploy Telegraf and Elastic beats agents with configurations programmatically generated from roles and services defined in our ServiceNow configuration management database. So all of that happens without any user having to point and click on any interface or manage any configuration themselves. All communication is mutual TLS authenticated. And we currently have about like 10,000 agents across three major data centers and close to 100 agents in a half a dozen other smaller data centers. We’re just a bit shy of half a million points per minute in Influx as of yesterday afternoon. And our footprint, we’re hoping to actually scale north of 50,000 Windows servers across a dozen major data centers and hundreds of on-prem client sites. Hit the next slide, please.
Chris Ruscio: 00:23:01.879 So what do we do with all that data? First, I do have to apologize. This diagram is a bit dated. Today, we do stream data directly from Kafka into Capacitor. Capacitor alerts are then sent to our ServiceNow event management. They tie back to the same configuration items and service maps that drove the Telegraf configurations on our previous slide. We also had an interesting challenge to overcome where our internet-facing services and our end users exist on two separate networks. So again, we brought in our F5 and our perimeter security team, and they provide us security and also Grafana hosted on our user network to access our Influx and Elastic clusters in our internet-facing network. One other thing is you’ll notice a bidirectional connection between Kafka and the Telegraf relays on the left side there. We use tag-pass and tag-drop filters in Telegraf to feedback a subset of data to a new topic which is then ingested into a much smaller dev environment on our user network. And that allows for rapid prototyping of new dashboards and alerts. The dev environment is a free-for-all. Production configuration is much more restricted. Nothing is deployed to production without being committed to source control and run through Terraform and SaltStack, and that dev environment and the functionality of Telegraf allows us to have a sandbox where our users can prototype and create anything that they need. Next slide, please.
Chris Ruscio: 00:24:32.344 So what are the outcomes of this and some of our learning experiences? The much faster delivery than our legacy tools. We used to have to RDP into a server, point and click on an install.exe, open up a dialog, maintain a configuration, sometimes file with it, sometimes registry keys. Sometimes, a GUI dialog used to take minutes or hours to deploy an agent. Today, we can deploy thousands of agents in minutes instead of a three-month project. We can reconfigure them in seconds. We manage half a dozen overlapping monitoring services today. And reducing that to a smaller footprint to manage, maintain, and secure will definitely reduce our operational costs and security risk. And being able to deploy monitoring in areas not feasible in the past due to costs, scalability issues opens us up to some new revenue opportunities. And then centralized information leads us to a reduced mean time to identification and mean time to resolution which improves support, service delivery, and most importantly, improves our client satisfaction.
Chris Ruscio: 00:25:39.921 Along the way we’ve definitely learned a few things. Our very first issue as we were rolling out Influx, we ran into some disk I/O issues in our InfluxDB cluster. We opened up a support ticket with Influx. They helped us tune some things. I think the main culprit there was Anti-Entropy if I remember. But we were just simply over capacity. So one of the things that they helped us come up with was a plan to switch from a single P30 Azure-managed disk for cluster node up to three disks, and that separated out one disk for a WAL drive, and then the other two disks and a RAID 0 for data, and so that gave us much better I/O performance when we had a lot of users querying that data and much more efficient ingest when we separated WAL from our data drives.
Chris Ruscio: 00:26:29.257 So at that point, things started to run very smoothly and the cluster started to ingest data at quite a fast rate quite happily which led us into our next problem which was some patent bandwidth saturation issues. In a couple of our DTCs, we ended up with so many Telegraf agents exhausting path tables on a firewall and consuming the available network bandwidth. About a year ago, we built and deployed what we called consolidation servers. And it’s funny, a few months later, Influx published, I forget, it was either a white paper or a case study but a very similar design that, I think, called them aggregation servers. But either way, it was nice to see we were on the right track and that others had come up with a similar solution. Deploying those servers though allowed us to vastly reduce the connections on our firewalls. And it also gave us one other benefit. We could then manage data center-wide all of the bandwidth which we could’ve done at the network layer beforehand but would have had to manage different ways in each data center with different teams. This gave us a way at the application layer to manage bandwidth across a wide deployment of agents in a single location.
Chris Ruscio: 00:27:41.669 And another side benefit there is because we were batching all of that traffic through a couple OF Telegraf relays, we saw a significant drop in bandwidth. And so that brought us to our next problem. Now that we had these Telegraf relays out in our data centers that were very happily consolidating and forwarding traffic, we ran into this issue where when we had a network outage, the Telegraf collection agents would basically [inaudible] the Telegraf relays when they came back online. So they were all trying to flush their buffers in RapidFire. It turned out we needed to restart thousands of agents to reduce their metric buffers and restore normal operations. We had just enough capacity to handle traffic under normal conditions. But when everybody was trying to catch up, there was just too much. And we actually have a feature request open with Influx support right now. We’re hoping that they’ll implement an exponential backup algorithm in the InfluxDB output plug-in. Otherwise, we’re probably going to have to switch to Kafka on our consolidation or aggregation servers to address that problem.
Chris Ruscio: 00:28:51.235 One other issue that was, I think, a couple months ago was released in Telegraf, we collect primarily PerfMon counters on a 1-minute interval, but every 15 minutes, we also collect Windows Service status. And that input is a very small amount of data, but it has a very large number of tags and metadata, and it creates a massive spike in the bandwidth and points written that we were seeing. Being able to jitter that collection one heavy infrequent input across a wider timespan significantly improved our bandwidth measures and our bandwidth over the internet. Another issue that I don’t know that this is a learning experience so much as a continuing education experience is that we are always, always tuning our batch sizes, metric buffers, and flush intervals between our Telegraf collectors, the relays in our data centers, and the relays between Kafka and Influx and Capacitor. I don’t think that that will ever have a final solution to that. That’s just one of those things that as each individual area grows, we find we are constantly tweaking and adjusting and optimizing to balance the load on the network and across those individual servers, services rather, with our desire to get the data from its origination point to eyes in front of a service console as fast as possible.
Chris Ruscio: 00:30:27.432 And one other issue I just wanted, a learning experience rather, I wanted to throw out here is that we had opened a GitHub issue. We, as I mentioned, used mutual TLS for all of our communication. We wanted to use Telegraf to monitor client certificate status for that, but the plugin did not work on certs unless they had a server [inaudible] usage key. And I opened a bug on GitHub. And Daniel literally, I think it was less than 48 hours, had it in a release. And so it was so wonderful to see something turn around so quickly there. If you hit the next slide. And so where does leave us and where are we going in the future? So on our roadmap for 2021, today we compile Telegraf agents with custom plug-ins for our business-specific services. And one of our developers right now is working to port that to the new Shim plugin. We hope to redeploy the official Telegraf agent that’s released by Influx and maintain a much smaller code base on our own of just our own in-house business logic alongside of the official Telegraf plugin.
Chris Ruscio: 00:31:41.392 Two of our other major goals for next year are to start to decommission those other more costly services and tools that we’re now providing a feature-complete replacement for and start to recoup some of those costs. We also need to invest more in [inaudible]. We already run Telegraf and Journalbeat agents on all of our servers. We need to do more to work to visualize and action that data though in the same way that we’re driving for our business services. And of course, as it’s getting closer to GA, we’re looking to upgrade to InfluxDB 2.0 and TICKscript to Flux. And I have one other story I want to share with you that is not on any of these slides because it literally happened last night. One of our VPs who’s a data junkie, he sent out an email last night saying that he was tired of trying to wrangle time series data in Power BI. And that’s in use as part of one of our other monitoring solutions. And thanks to the work that we’ve done to build configuration of code in Terraform and Salt and the work that Influx has done to provide a very well-documented container image with clearer configuration planes, we had a fully provisioned and secured Influx Chronograf and Grafana environment for him running by the end of the day. I think he sent an email, somewhere around 5:30, I was eating some dinner, and by 6:30, he had his environment. And all it took was just the definition of a few environment-specific variables and a Salt highstate. I mean, I cannot overstate the flexibility and efficiency of these platforms. And if you could hit the next slide.
Mike Montano: 00:33:34.694 All right. We are at that point. Thank you, Chris. Questions. I think there is one that came in to [crosstalk].
Caitlin Croft: 00:33:42.888 Yes. What sort of redundancy is built around Kafka and Grafana?
Chris Ruscio: 00:33:54.841 Let’s see. Kafka. So Kafka, we have a replication factor of three for all of our topics, I believe, so we could lose up to two nodes and still have data. That data lives for a very short time. So we feel that if we were to lose, say, the entire Kafka cluster or we were to lose, say, well, the entire Influx cluster, we would be able to build up. So let’s say the entire Influx cluster got destroyed. We would reprovision it with Terraform, restore backups. And given the volume of data that we’re collecting right now, that might take a day or so to process to rerun those backups. At that same time, we have, I think, capacity for seven days of retention right now in Kafka. So during that time, we’re still receiving data. And as soon as Influx is back online, we would feed all that downtime back into Influx and have no gaps in our data.
Chris Ruscio: 00:35:01.754 On the flip side, everything is consumed from Kafka almost in real time. So if a Kafka cluster went down, if we lost a couple of nodes, no problem because there’s sufficient replication. If the whole cluster went down, we would have a break in our flow of data while we got it back up. But again, it would be a very quick action. We would just redeploy it with Terraform and we wouldn’t care about any restoration of data because all of that up until maybe we might have a few minutes between - not even. We might miss a few seconds that didn’t get consumed before it crashed. But there’d be nothing in a back-up that would be worth restoring because it’s already been consumed and in Influx. And on the Grafana side, as I mentioned, we have two instances. One is fully provisioned, so no concern about loss there. We just reprovision it. And then the other is sort of the more open environment. And we take, I think, hourly back-ups of the database and so, just again, reprovision, restore the very small SQLite database, and we’re up and running again. Does that answer the question?
Caitlin Croft: 00:36:16.356 We will find out [laughter]. Let’s see. There’s a few other questions. Yes. Okay. So you did answer his question. Is it scalable to add a new streaming technology that might come up in the future? What changes are expected?
Chris Ruscio: 00:36:37.897 Scalable to add. So I’m not quite sure what that question is there. I will say, so there’s been a couple of things we’ve looked at. So as I mentioned, right now, we use Telegraf relays to stream data or consolidate data and stream it across the internet from our data centers. And we’re looking at replacing that with Kafka because of the performance issues there. I’ve been keeping an eye on replacing Kafka with Pulsar. One of the things that has crossed my mind that I don’t think we’re going to approach, but in the same way that we have a unified visualization platform, there’s some appeal to having a unified alerting platform and the only place I can think to efficiently do that would be Kafka stream processing. The only way to alert on both the telemetry and the log analytics at the same time would be in Kafka. So certainly, switching from some of the stream processing in Capacitor or in Influx 2.0 over to Kafka stream processing is one of the options that we like having available but not one that I think we’re going to go down. I’m not exactly sure what the intent of that question was. Hopefully, I’m coming close to answering it.
Caitlin Croft: 00:37:53.576 I think you’re coming close. They had it on to replace Kafka as an example. So I think you kind of covered that.
Chris Ruscio: 00:38:01.004 Yes. Yes. So Kafka specifically. We’ve looked at Pulsar and are quite curious to see how that develops.
Caitlin Croft: 00:38:10.309 Cool. How did you guys hear about InfluxDB?
Chris Ruscio: 00:38:16.740 I’d like to say Google [laughter]. I mean, this project started almost five years ago. As Mike mentioned, he and I and a couple of folks just chatting about, “Wouldn’t it be nice if? Wouldn’t it be so much better if?” And it ruminated for a little while until we started seriously building a proof of concept, I think three years ago now, and then working on getting approval through our compliance and security teams to deploy into production two years ago. And when we came across Influx, at what point it was, “Yeah. No. This is the tool,” I don’t remember exactly. But there was a lot of research involved in a year or so period to make sure that we found something that truly fit what we were looking to do, and Influx fit that.
Caitlin Croft: 00:39:15.177 Awesome. What do your retention policies look like?
Chris Ruscio: 00:39:20.897 Would you like to know what they look like or what they should look like? So one of the advantages of only being deployed to about 7,000 servers when we want to build a system that’s going to scale to 50,000 servers is I haven’t had to worry about that much yet. So today, our retention policy is just raw data collected for six months. There’s no down sampling or rolling up, and that is a problem that we recognize we’re going to need to face as we scale up. We certainly don’t want to - I mean, in a perfect world, if storage and compute were free, we would want to keep all that, but that’s not going to scale for us. We are definitely looking at - I think our plan is to try and keep raw data for a whole month and then scale that back down into a day for a couple of months and then maybe even a little bit longer than that. Or I’m sorry, not a day. Down to hour intervals for a couple of months, and then after that out to six months at day intervals.
Chris Ruscio: 00:40:29.527 And to put that in context, we have a couple of our tools that downsample the very next day. So you can’t see more than a 15-minute average or a one-hour average for what was happening yesterday or the day before which becomes incredibly problematic when somebody says, “Hey. This problem has been happening for a couple days or a couple weeks. And we don’t know what’s going on. Can you look at what was different two months ago before our upgrade, four weeks ago before the problem started, and then the last three weeks when it’s been going on?” If you don’t maintain that data, you can’t answer that question if you aren’t aware of the problem beforehand. So we have a - one of the reasons that we needed a time series-specific database was to be able to handle so much data and keep it for long enough to start to answer those historical questions.
Mike Montano: 00:41:22.039 Yeah. That’s a really good point that Chris brought up there too, I mean, from a management perspective. Some of the commercial tools that we use, they don’t go back very far in retention. I mean, one of the tools we have is between 45 and 60 days. That’s a max. And once we get the alerting data over to ServiceNow and things like that and we do reports that way, not necessarily going back six months or something like that. But however, there are definitely use case, like Chris mentioned. During upgrades, going from this version of our solution to this version of our solution, things were different. How were they good or bad? And having that information stored for long periods of time is critical. But the use cases are smaller in terms of retention, I think, more of kind of those situations that Chris brought up.
Caitlin Croft: 00:42:13.380 Great. And you guys are obviously in the healthcare industry. Are there any HIPAA rules or any data privacy issues or rules that you have to be concerned of with your operations?
Mike Montano: 00:42:28.283 Absolutely. Absolutely [laughter]. We are definitely under that regulation. But the other thing too is that’s just for the United States. I mean, globally, there are other situations that we come across where certain parts, certain international areas of working internationally, they don’t allow data to come out of their province or their country and that sort of thing. So yeah. Absolutely, we have to deal with that. And Chris can probably go into more detail, but most of the information that we collect and send is data. It’s metrics. It’s percentages. It’s things like that. It’s not patient information or anything like that [inaudible] tracking that kind of information or a user making patient names, usernames, things like that. So I don’t know, Chris, if you had any [crosstalk].
Chris Ruscio: 00:43:17.427 Yeah. So I’ll add two things to that. One of them is that our design absolutely planned for the need to say - I’m drawing a blank on which one of the countries it was that had the data sovereignty laws, but the ability to take and create a mini cluster probably with open source Influx instead of enterprise Influx, so a single node instead of a cluster but be able to drop a small appliance that is sort of a little mini version of our overarching environment so that we can manage in the same way that we do our main environment and we can maintain data separation and data sovereignty. So we did absolutely choose the components that we chose with that in mind of needing to be able to scale down, scale up in those specific needs.
Chris Ruscio: 00:44:07.227 And then the other thing I’ll add is that, as part of our security and compliance, one of the questions that’s asked is, “Will there ever be any personally identifying information [inaudible] a whole checklist.” So that’s the main question in this context is, “Will there ever be any personally identifying information being stored in this solution?” And we do have a number of our monitoring solutions where the answer to that was no. But my take on it is I have no intent to ever consume that data, but I have every expectation that it will somehow, someway get introduced to some log. And so a very key part of our solution is ensuring that we have the ability to meet all those requirements that we can secure the communication channels in the way that we need to, that we can audit logs and provide answers to the questions that we need to provide answers to and that we can encrypt data at rest and track who it is that’s looking at that. So that absolutely comes into every design choice that we’ve made is what are the security implications and data vulnerability of those decisions.
Caitlin Croft: 00:45:17.315 Yeah, absolutely. We definitely have seen other community members do just as you described for data separation where they have a different instance for a specific region and then they send the aggregations to the main instance and then downsample the local data at the time interval that keeps them in compliance. So it’s just interesting, and healthcare data’s always, there’s [laughter] a lot of security issues around that. So we have a few more questions here. How do you use authentication in your InfluxDB cluster? And yes, how does your Telegraf relay deal with that?
Chris Ruscio: 00:46:00.639 So the Telegraf relay isn’t - so the InfluxDB cluster authentication is just basic AuthZ with a couple of user accounts. We have a user account that has permission to read, a user account that has permission to write, and then we have an admin user account. And there’s a few other things that go on there and a few other database-specific silos. But that’s really a very simple layout on Influx. Where we manage that is in two places. The first is that Grafana is LDAP authentication. So to get into Grafana to view dashboards, you need LDAP access, and no user has direct access to Influx, so you need to go through Grafana to get there. The dev environment that I mentioned has filtered data for two reasons. The first is to make sure that only data we’re fine with a wide number of eyes seeing end up there for that sort of sandbox space and the second reason is just because we don’t want to have a full copy of production. But part of the reason that we do that filtering is to restrict what data people have access to, and that sandbox environment’s kind of free for all.
Chris Ruscio: 00:47:06.774 So production, you have to authenticate with Grafana through LDAP and we’re moving to sample for that. The relays within our data center just use that one shared-right account to consume from Kafka and then write into Influx. And then our collection agents, we have a peak AI infrastructure where every single agent has its own client certificate, and those certificates are basically similar to tokens in that they have an expiration of, I think, it’s either eight or nine days, and we cycle them weekly. So we have a very short-lived certificate per agent that provides access to send data to our Kafka brokers.
Caitlin Croft: 00:47:49.862 How are the query results in terms of time benchmarked in your environment? How did you arrive at that? Can you share any thoughts on it?
Chris Ruscio: 00:48:01.357 How are the query - well, I don’t know that we’ve done very much to benchmark queries other than just simply tuning slow-performing Grafana dashboards. So for example, we had one Grafana dashboard that had a series of variables which were supposed to be selected to reduce to the point where we would be filtering on a reasonable amount of data. I think it was - we collect windows PerfMon process. So every single process running on the machine, data about every single process. And so we had some, I think, Citrix servers that ended up having where some servers would have a dozen of this process. These Citrix servers with hundreds of user sessions on them had thousands of processes, and that just created a - Grafana generated this Influx query that - I think the query itself was - I forget how many tens of kilobytes or megabytes it was trying to put, first in a get request, and then we enabled the configuration to put that in post request which helped a little bit.
Chris Ruscio: 00:49:12.733 But then we had to take another look at that and implement Grafana’s ability to use wildcards in its query filters, but that also meant that we had to redesign our query to make sure that logically instead of just if we said this and this and also a wildcard for this field, we would end up - we ended up broking this sort of staggered filtering. So I guess all that is to say that we’ve definitely had some performance issues and some lessons learned there on how to manage variables and how to manage the construction of Influx queries in Grafana. But we haven’t, say, for example, had the need yet to go in and perform log analytics on the Influx cluster to identify long-running queries and specifically target them. It’s just been, “Oh, this dashboard is running slow. Let’s dig into that.”
Caitlin Croft: 00:50:08.391 Right. Do you have any plans to put more security events into InfluxDB?
Chris Ruscio: 00:50:19.468 So we continue to look at - at the time that we made these original decisions, Elastic had a very weak metric story, and Influx did not have any log story whatsoever, and that’s definitely changed. And so we continue to look at consolidating on one of the two tools. And I think that Elastic still to this day does not have nearly the storage efficiency to handle the metric collection that we’re looking at doing. On the flipside, I’m cautiously optimistic that Influx has the capacity to handle the log ingestion that we’re doing. I’m not entirely sure about that yet. I think that today, it still makes the most sense to keep the two separate. So we don’t today do any log ingestion, security or otherwise into Influx, but it is something that we continue to look at as a possibility.
Caitlin Croft: 00:51:16.106 Can your developers add their own metrics to the InfluxDB instance?
Chris Ruscio: 00:51:24.289 So we have a number of developers that prototype on their own instances, and then we build that into the provisioning that builds Telegraf scripts. So we have a basic, sort of general - so just a little context. I don’t know how well I covered this in the presentation, but 99.9% of what we monitor is Windows Server. So we have a Telegraf conf file that has some agent config, some global tags, and some output config. And then all of our role-specific inputs are broken up into different configuration files that go in the confd directory or telegrafd directory. And so we have developers who will for different products and services identify the information that they need. For example, right now, we’re working with our VMware team to start to collect some, yeah, more specific metrics that they need. And next, I think, coming up, we’re going
to be doing some improvement to our Citrix agents or provisioning agent collection. So these teams will come to us and say, “These are the things that either we do today [inaudible] or the things that we want to be able to do.” And so we’ll work with them. We’ll build a configuration. We’ll work on building the provisioning that determines from our configuration management database, “Oh, this server should get this config. Let’s dump it on the agent, so it starts collecting that data.” But they don’t directly manage modifying any Telegraf config on a deployed agent.
Caitlin Croft: 00:52:56.667 Perfect. All right. Well, thank you both. It looks like we’ve answered everyone’s questions. Thank you so much. It was a great presentation. I just want to remind everyone once again that we have InfluxDays North America coming up next week on Tuesday and Wednesday, so it’s November 10th and 11th. As I mentioned before, it’s completely virtual and it’s completely free this year. So we’re really excited to see everyone online chit-chatting with each other and just learning from each other. I know it’s obviously a little bit [laughter] different this year with everything being virtual, but we’re still really excited to have everyone there. So please, feel free to go and register. We have tons of live sessions as well as on-demand sessions that will be available next week. So lots of presentations from InfluxDB engineers as well as community members. You can learn about how other InfluxDB users are using the platform as well as hear the latest and greatest from our team. So please be sure to check it out. Hope to see you there. Thank you everyone for joining today’s webinar. Once again, it has been recorded and the slides and the recording will be made available later today. Thank you, Mike and Chris, for presenting. And I hope everyone has a good day.
Mike Montano: 00:54:29.583 Thank you.
Chris Ruscio: 00:54:30.710 All right. Thank you.
[/et_pb_toggle]
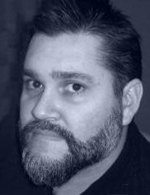
Mike Montano
Sr. Manager, Allscripts
Mike Montano is a senior technical support manager and IT operations manager at Allscripts. He manages an operations and development team delivering application monitoring solutions used for Hospitals and Health systems across the United States and abroad. Mike has spent over 20 years in IT support and service delivery in the area of commercial and custom-developed application monitoring solutions and services.
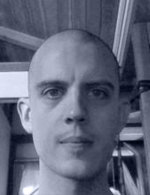
Chris Ruscio
Solutions Architect, Allscripts
Chris Ruscio is a solutions architect at Allscripts. For over ten years he has driven modernization and consolidation of legacy monitoring software across a diverse landscape of Hospitals and Health Systems. He has a passion for open source adoption and contribution. He strives to enable more efficient/improved customer interactions, accelerate innovation, and help better the experiences of colleagues and clients.