Introducing InfluxDB Cloud Dedicated
Session date: May 23, 2023 08:00am (Pacific Time)
InfluxData is excited to announce the general availability of InfluxDB Cloud Dedicated! It is a fully managed time series database service running on cloud infrastructure resources that are dedicated to a single tenant. With this new offering, we’re excited to provide our customers with additional security options, and more custom configuration options to best suit customers’ workload requirements. Join this webinar to learn more about InfluxDB Cloud, and the new dedicated database service offering!
In this webinar, Balaji Palani and Gary Fowler will dive into:
- Key features of the new InfluxDB Cloud Dedicated solution
- Use cases for using the newest version of the purpose-built time series database
- Live demo
During this 1-hour technical webinar, you’ll also get a chance to ask your questions live.
Watch the Webinar
Watch the webinar “Introducing InfluxDB Cloud Dedicated” by filling out the form and clicking on the Watch Webinar button on the right. This will open the recording.
[et_pb_toggle _builder_version=”3.17.6” title=”Transcript” title_font_size=”26” border_width_all=”0px” border_width_bottom=”1px” module_class=”transcript-toggle” closed_toggle_background_color=”rgba(255,255,255,0)”]
Here is an unedited transcript of the webinar “Introducing InfluxDB Cloud Dedicated”. This is provided for those who prefer to read than watch the webinar. Please note that the transcript is raw. We apologize for any transcribing errors.
Speakers:
- Caitlin Croft: Sr. Manager, Customer and Community Marketing, InfluxData
- Balaji Palani: VP Product Marketing, InfluxData
- Gary Fowler: Product Manager, InfluxData
Caitlin Croft: 00:00:01.496 Good morning, everyone. Good afternoon. And welcome to today’s webinar. My name is Caitlin Croft. And I am joined today by Balaji and Gary, who will be talking about InfluxDB Cloud Dedicated. Once again, this webinar is being recorded and will be available for replay, as well as the slides, by tomorrow morning. And beyond Balaji and Gary, we have Rick and others from the InfluxDB team here. So post any questions you may have in the Q&A, and we’re really excited to be here. So without any further ado, I’m going to hand things off to Balaji.
Balaji Palani: 00:00:41.099 All right. Thanks, Caitlin. Good morning. Good afternoon, everyone, wherever you’re joining from. I’m just going to go over this, I have a brief introduction here. So again, I’m Balaji. I lead Product Marketing at InfluxData. I’ve been here at InfluxData for about five years. I just joined Product Marketing recently. Prior to this, I was in Product Management. You might have seen me in the community, answering questions and so on. It was quite all right. I mean, basically I was very close to the product. But I felt like some of the technical knowledge that I have could also be useful for the product marketing. So I’m super excited about this new role and jumping in straight away. So I’ll hand it over to Gary for his quick intro.
Gary Fowler: 00:01:29.662 Hi, everyone. I think I’ve talked to quite a few of you on the participant list before. But those of you that I haven’t spoken to, I’m Gary Fowler. I’m a product manager here at InfluxData. Among the several things that I’ve been working on, one of them is helping with the cloud dedicated offering. So that’s what I’m here to talk to you about today.
Balaji Palani: 00:01:52.139 Awesome. All right. So let’s see a quick agenda. So I’m going to be kicking off first. I will talk about InfluxDB 3. You might have heard on the news, as well as last few weeks, blog posts and so on, on InfluxDB 3. So if you’re wondering what it is, I’ll be covering some of the highlights, and a bit of a detailed dive into the architecture. And then Gay is going to talk about cloud-dedicated, introduce it, and you’ll also get to see it in action, a quick demo on it. So without further ado, let me get started. So before I get into the meat of it, I want to start with a statement. Right? So successful businesses, and you can take any businesses, internet, SaaS, data enterprise, whatever, I believe that they are defined by the experiences that they create and deliver to the end users. However you interact with it, it goes from the UI to the back end to getting different things. So everything is created by that experience.
Balaji Palani: 00:03:03.327 And this is how we define some of these successful companies, such as Tesla. Right? Tesla here, in this case, we are showing Powerwall. Powerwall is not just an application. It has many components. It has solar panels, battery storage, mobile applications. It has a very rich, adaptable interface providing real-time data regarding power generation and consumption. Nest is another. I believe it’s an innovative company. They started with a very simple kind of learning thermostat. Right? But they do more now. Right? So they’re part of that [Nest home?]. And it’s just complete experience they provide. Disney Plus, a leader in video streaming. Rappi is also one of the largest online e-commerce marketplaces in South America. Each of these presences, I believe they are living, rich user experiences that are backed by data and real-time analytics that are powering those experiences. That’s what defines how different they are from some of their competitors. For example, the recommendation that you see when you browse with different devices and so on, or search for your favorite shows, based on that, what new movies you see there, and the way you click on them, and start from wherever you left it last time, and so on.
Balaji Palani: 00:04:24.297 All of these things, they have one common element behind the scenes. In all of these applications, time series data, we believe, is a foundational component. There could be other data. There could be things that you need different databases for. But we also believe that time series data is a foundational component of these applications and services, and that is what powers those rich experiences that you see, from investigating incidents [through Kubernetes?] application architectures, to analyzing real-time events, all of these data have common [invests?] on time, and they allow you to gain critical insights and observe trends and detect anomalies, so even predict the future events. So what are the kind of time series data we’re talking about here, right? So some of these time series data are metric events and tracers. Metrics are definitely sort of a type of time series data, but they are collected regularly over time. Right? And some examples would be from [capture?] from a center, or available free memory from your laptop, or disk space, and so on. They are very useful in visualization analytics, such as correlation forecasting and so on. Events, they are things that happen irregularly, and usually are indicative of a state change or a trigger. They could be an event — a simple example of an event could be a log file entry, an error from a log file, they could be an alert. They are very useful for summarization, to convert regular time series and for the analytics. Or just simply alert on them, right? So you just notify people to act on them, and so on.
Balaji Palani: 00:06:07.789 Traces are, actually, another time series type. They are actually a log capture of the request, and how it propagates to a distributed network or a cloud infrastructure. They are also useful in correlating finding root cause analyses, causal analytics, and even visualizing your execution pathways, and so on. So what about the sources, right? So time series data — they are usually time stamped, and they could be generated hours, minutes, seconds, or nanoseconds. They could be from different sources. It could be the factory floor, or from networks and infrastructure. They could also be coming from the physical world. Solar panels, windmill farms. We have so many of your physical entities, physical devices, sensors available, that this data could be captured and sent to do some central analytics and so on. They could also be from a virtual world, from a Kubernetes infrastructure, or cloud services, or even by a [inaudible] script that just checks API response times. So all of these are time series data.
Balaji Palani: 00:07:29.554 There is one unique characteristic about time series data. There are a couple, or a few, but basically, time series data are coming in at massive scale. They’re just collected very, very, fast, sometimes in milliseconds. You have many, many data points created per second. They arrive quickly. They could be batch. They could be sent in batches because you may not have connectivity, or intermittent connectivity. And then, they could also be streaming. You can just have a lot of cases where just the real-time data is just coming in. They are coming in at massive scale as well. Okay? So billions of data points, or billions of data series. Series could be many different types, or many different devices, and different combination of them. And these services and the applications, they have to take some action on real time based on the data that’s incoming so quickly and at massive scale. You look at that data, and with the context that they provide, you may have to do anomaly detection, or do triggering alert, or automation, something else. So all these are parts of what you could do with the time series data.
Balaji Palani: 00:08:48.425 And what we have seen, again, this is by experience, this is by working with customers, most general purpose databases simply cannot handle these time series data scale, simply because they’re not built for it. They’re not purpose-built for that. Some of the examples are time series relational data, for example, they are optimized for processing transactions, online transaction processing. They’re not built for those time series, for instance, with heavy reads and writes, for example. They’re built for consistency. Consistency is, hey, data comes in, I want to make sure that the same data point exists in different replicas distributed across the network. So when you optimize for consistency, you miss out on the scaling. And then complete data life cycle management. Because when data comes in, again, it’s coming in time. So you might want to keep the recent data more current. You might want to keep the queries really fast for the recent data. And then, you might want to retire some of the older data. In a year, maybe, you archive it some place. You kind of put it in a warm location, so that you don’t exactly need it immediately, but then maybe after some time, if you’re trying to do some trend analysis, you may want to query that data again. The entire life cycle management, if you try to build it with a relational data source such as Postgres, it’s going to take you a long time. You’re going to spend a lot of time doing things which are built into some of these purpose-built solutions.
Balaji Palani: 00:10:31.303 So what does purpose-built really mean, right? So for example, InfluxDB, we are designed for scale. We, basically, the way we have architected is we don’t block writes and reads. So you can do hundreds of thousands of writes coming in, and at the same time, you can a lot of queries just querying the data at the same time. The architectural differences make them service those high-volume writes and reads together without any issues, without any blockages that’s happening. Availability. We really prioritize data availability, kind of write and read availability, over consistency. So you will find that in InfluxDB, it’s eventually consistent. But we’ve taken all of those writes. That’s why we’re able to even support massive time series data that come in at very, very high speed. And as I mentioned earlier, the data life cycle management is built on data retention, or [shorting?], and all of those concepts are built into the database without having you to worry about those things.
Balaji Palani: 00:11:39.774 So next couple of slides I’m going to talk about specifically InfluxDB 3.0. We’ve been building InfluxDB 3.0 for the past year and a half. And we have delivered in two flavors in cloud, Cloud Serverless and Cloud Dedicated. And it’s a differentiator. It’s so much better in so many different ways from what we have done in the past with TSM, [inaudible], and so on. InfluxDB 3 provides single data store for metrics, events, and traces. This means your time to learning and insights are much, much lower. It is designed to deliver sub-second query responses. The way we do it is the data, especially the recent data, live data that’s coming in, it’s available in memory. And all of the query responses are so fast. You can really, really focus on building awesome end user experiences with that. With InfluxDB 3.0, you don’t have to choose, “Should I store this data wherever? How do I keep my costs low?” So you can do both. Right? So you can store your data. Typically, you would promptly archive your data. But here, you store your data. And you can also expect to pay lowest cost because we are storing it on cloud object store, and it’s storing at high compression. So you’re getting the benefits of really, really storing a lot of data at the lowest cost possible.
Balaji Palani: 00:13:16.709 There are other benefits as well. With InfluxDB 3, we are very developer focused. We support InfluxQL, as well as SQL native support. Both of these make it really — you don’t have to have a big learning curve. So it just works much better working with time series data. It’s also designed to be interoperable with other machine learning tools, or data lake, with examples like do your copy sharing, and features that would allow you to optimize on your data sharing, and work with other tools such as Jupiter Notebooks, which we’ll see a demo today. Or perhaps you want to do Spark machine learning tasks on your data, and so on.
Balaji Palani: 00:14:08.375 So let’s look into some architectural details on why InfluxDB 3 is so much better. For example, we said, hey, we will store metrics, events, and traces together in a single data store. How is that possible? Cardinality. The reason when, you have all of these three data types, and you’re trying to store them in a single data store, you end up with really, really high cardinalities. Think billions of series. Because tracing, for example, it has, depending on how many spans you have with an [inaudible], you could end up with a lot of different combinations of tags and columns. And those combinations typically explode your cardinality, which in any time series database is going to be a problem. Your writes and [inaudible] slow down with an extremely high cardinality. But with InfluxDB 3, we have solved that problem. Typically, we will see that in that time series, for example, in TSM, we used inverted indexes to store the different tags and [inaudible] where the data is located and so on. The inverted indexes, in InfluxDB 3, we don’t do any of those. We use a catalog to track the schema changes and [inaudible]. We use time base partition. We use optimization. All of these combinations allow you to really get to that data pretty quickly, find that data and that [inaudible] really quickly without really doing all of those other things. This is where other databases fail.
Balaji Palani: 00:15:47.081 And what this helps you in doing, it now has, with InfluxDB 3, you don’t have limits on cardinality. You can store any kind of wide tables, or high dimensional data, into InfluxDB 3, which means metrics, traces, and events. All of them together just simply makes things faster when you want to look at insights and so on. Sorry about that. One second. The other thing that we do in InfluxDB 3 is we have tiered storage. Kind of we store hot and cold. We use hot/cold storage tiers for performance and low-cost. What does hot data mean, right? So as data comes in, data is loaded into memory as Apache Arrow format. And everything, all of the core of InfluxDB 3, is Apache Arrow. It works on the data in memory. It’s optimized for really, really fast sub-second query responses. So any data that’s recently queried, or just arriving pretty recently, is in memory and can be read.
Balaji Palani: 00:17:02.489 And that’s the concept for hot data. And anything else, right, so all of the data is eventually persisted in an object store as Parquet. Parquet is a very well-known, open source, popular format. Has the highest compression. And not storing it in a cloud object store like S3 means you’re optimizing for really, really low cost. And you don’t need, as I mentioned earlier, you don’t need to decide whether to store this data for a year or forever. You can store it forever. And when you run another query which does a trend analysis, we just kind of collect everything. So InfluxDB is optimized from the query engine side, but it can load data from memory, and load from Parquet, combine them together, and present the results. So all of these are very groundbreaking, and it just makes it a really better performance, as well as storage.
Balaji Palani: 00:18:02.412 Open architecture for interoperability. Right? So we talked about, I mentioned briefly earlier, how does this happen? So all of these Parquet, when data is persisted as Parquet, it also means that when we store them in S3, we have other options, such as leveraging [inaudible] copy, data sharing. With that, you can actually do your machine learning tasks or other data science activities without really topping that data. So much better in terms of data efficiency. And you can work with your data science teams. You don’t have to have multiple copies of them floating around, and then trying to manually kind of bring them together, and do all of those data [inaudible] that you could do with InfluxDB 3. This is, again, one of the exciting things that we are super excited about.
Balaji Palani: 00:19:04.463 So how can you use? So that’s something with InfluxDB3. How can you use these new features that way? So some of the ways you can go interactively between your environment is, if you’re doing some building internal data lake for monitoring — we have several customers who are actually doing this. They collect metrics data all over the place from monitoring the environment. And they use InfluxDB as their kind of data lake. Again, just for time series data. Right? So you can do that, that coexists with other data lake technologies, or other data types, and so on. You can also build your SaaS applications or even an IoT. Perhaps you have sensors collecting data from your factory floor. Or a manufacturing company is doing predictive maintenance. All of those cases, you can use InfluxDB, especially with the scale and performance that we support. Some of the examples that we have is predictive analytics and maintenance. And on the SaaS application side, we have several customers who are using our solution for building their racing analytics, or log platform that they offer to their customers. So those are also some use cases that you can do, think about.
Balaji Palani: 00:20:31.773 Some of the examples, further examples of customers adopting InfluxDB by industry, we have many different examples here. Some they’re when crypto was really popular. Yeah. That would be this. So some of our customers, network telemetry, some of the largest network providers or mobile operators. Media companies. All of those are examples who are using InfluxDB 3. So this is another — if you are a developer, you are thinking about, “Hey, how do I ingest data? Or what kind of visualization analytics tools?” Imagine your data, all the time-stamped data, is coming in. You can use Telegraf. Again, we have 300-plus plugins. Or we offer client libraries in different languages that allow you to write and read data. You can use it in code. And then you’ll [inaudible]. And then also, with our APIs, you can connect any one of these visualization tools, such as Grafana, Superset, or even use, maybe, other technologies like [inaudible] to build your learning and so on and so forth. So all of this is possible [inaudible].
Balaji Palani: 00:21:58.951 This is another kind of view onto you can run InfluxDB 3 on either cloud, using Cloud Serverless, or Dedicated. The difference is Cloud Serverless is a multi-tenant solution, so you get started with your workload really small, and you can just pay as you go pricing. You can just pay for what you use. Or you can choose to say, “ Hey, I’m big enough. I want to have a cluster just for my workload, and choose Cloud Dedicated.” In which case a cluster is spun up, and it’s exclusive just for you to run your workload in. We plan to deliver InfluxDB Clustered, which is an evolution to the InfluxDB Enterprise, if you have used it before. And Edge is another offering that we would support, which is nothing but a single-load InfluxDB running on the edge, giving you the power of InfluxDB 3 on the edge. So with that said, I know I spoke a lot, but I hope that gives you a quick overview of InfluxDB 3.0, and all of the different offering. I am not going to hand it over to Gary to talk us through the cloud dedicated pieces.
Gary Fowler: 00:23:19.127 All right, Balaji. Thank you very much. That was a great overview of InfluxDB 3, which is important because InfluxDB Cloud Dedicated is our vehicle for delivering you a dedicated, hosted, managed version of InfluxDB 3. So what is InfluxDB Cloud Dedicated? It’s called dedicated because it’s a cloud instance of InfluxDB specifically for an individual customer. A single-tenant solution for a single customer, tailored to fit their workload. So we see customers with a lot of different types of workloads. Almost every customer is at least a little bit different. Some customers have much heavier ingestion workloads and lighter query workloads. Some have less ingestion, but have a ton of query, and a ton of very large queries. So with InfluxDB Cloud Dedicated, we can size the number of ingest nodes that we use. We can size the number of query nodes that’s used to match that workload. We can even tune to the type of queries you perform, in some cases. So it really makes it where we are managing an instance of InfluxDB 3 specifically for you and for your needs. And then with capacity-based pricing, you really only pay for what you’re using. And then with the instance that is dedicated to you, brings more security options, private link type security options, and you get that enterprise-grade security.
Gary Fowler: 00:24:51.353 So why should you use InfluxDB Cloud Dedicated? As I just mentioned, it can be tuned to your workloads to ensure you’re getting the best performance experience. And as your data needs grow, it can continue to scale and grow with you as well. So I mentioned that we have separated the processing from ingestion and querying. We’ve done that so we can easily scale up. Right? So say you’ve introduced a new application into your environment. Now you have a lot more data. We can scale up ingest for you. Let’s say you bring on a whole new set of users, or you’ve deployed a new application to your customers, and now you’re doing a whole bunch more querying than you used to. We can dynamically scale that up for you, and grow with your needs. And then our first offering of InfluxDB Cloud that’s available right now, we have AWS support, really, in the AWS region, if you want. And soon we’ll be following with Azure and GCP as well, so that you can have the data in the location that makes the most sense to you.
Gary Fowler: 00:26:03.843 All right. So you’ve heard enough talking. So why don’t we go ahead and see this in action. So I’m excited to show you a demo today. I’m going to share my visual studio screen here, if I can find it. And I’m going to show you a demo. Now, I want to set the stage for this demo. Actually, Balaji, do you want to show the slides again? I missed a couple of slides I want to do before the demo. Yeah. Sorry. Nope, my bad. [silence]
Gary Fowler: 00:26:57.893 Okay. So what I’m going to do with the demo is I’m going to show a real-time analytics use case. I’m going to show you how you can do real-time queries with both SQL, something that we introduced with InfluxDB 3.0, something our customers have been waiting for a long time, and InfluxQL for existing customers that have been using InfluxQL and like its ease of use. We’re going to show how easy it is for a data scientist or a business analyst to use Apache Arrow to get a large dataset from InfluxDB 3 for analysis. I’ll show how easy it is for them to use some of the tools they already use and are comfortable with, like pandas, which is a very commonly used, popular data analysis tool. So I mentioned Apache Arrow. For those of you not familiar already, it’s a framework for defining in-memory columnar data. Apache Arrow provides a cross-language, in-memory data format designed to improve the performance and efficiency of big data processing. It enables efficient data sharing across different systems and languages without the need for expensive data serialization and de-serialization. Arrow provides a standardized was to represent complex data structures in a flat memory format, allowing data to be easily passed between different data processing frameworks and languages. It supports a broad range of programming language. It’s increasingly becoming a standard way of representing and processing data in the big data ecosystem. With this demo, I’m going to show you how easy it is to get data from InfluxDB, from Cloud Dedicated, down to an Arrow table within your application. Next slide, please.
Gary Fowler: 00:28:46.679 We’re also going to look at Apache Parquet. So Apache Parquet, for those of you who don’t know, is an open source file format designed for efficient and high-performance data storage. And it’s really used by a wide range of frameworks, like Apache Spark [inaudible]. It’s really becoming kind of the new CSV, the new and improved CSV. It’s just a format that a lot of different tools use. Parquet is designed to store structured and semi-structure data in a way that allows for efficient compression and coding of data, which allows for big, significant improvements in data storage and processing and performance. You can see here from this table, you can see how much data gets compressed with the Parquet format. Next slide, please.
Gary Fowler: 00:29:37.910 So I mentioned all of this because Apache Arrow, Parquet, and Flight are all part of the Apache software foundation, and they’re used by InfluxDB 3. That means the benefits of those things are available to InfluxDB 3 customers. And likewise then, available to InfluxDB 3 Cloud Dedicated customers. It is something that we’re really excited about, and a lot of the folks that I have talked to in the data science and machine learning world are really excited about too, because these are tools that they use. All right. So you can stop the share now. I am going to go ahead and share my screen again. [silence]
Gary Fowler: 00:30:30.231 Okay. So first, I want to warn everyone, I am not a software developer. I’m a product manager. But I do play one on Zoom, and I’m going to play one here today. For this demo, I’m going to use Python and a tool called Jupiter Notebooks, common tools used by data scientists and business analysts out there. So this first section here is just defining our dependencies. And what I want to show here is how easy it is to set up Flight for getting data from InfluxDB. They’re just the resource dependencies, and just a few lines of codes. And there are samples on our website. The data scientists can go, and basically, these are just boilerplate. They can just copy these things. The next section here is I’m setting up a couple of functions for setting up InfluxDB queries. So I’m going to do it two ways. I mentioned that we support SQL now, so we’ll do it via SQL. But we’ll also do it via InfluxQL. So defining a couple of functions here for that. The next function is I’m actually going to do an InfluxQL query. I’m going to get 100,000 rows of high cardinality data. I’m going to do it via SQL. And at the end, I’m going to write that to a Parquet file. So this is easy to run in Jupiter Notebooks. I can run it all at once, or I can go section by section. The dependencies take no time at all. So run them, defining these functions takes no time at all.
Gary Fowler: 00:32:04.776 Now we’ll get right to the query. So when I run this query, what’s going to happen is it’s going to communicate directly with InfluxDB 3, and it’s going to get real-time data. What I mean by real-time data, in this case, is we’ve eliminated TVR in InfluxDB 3. There’s no queueing that’s involved. When you ingest data, it’s immediately queryable. You have a zero TBR, time to be ready. So when we go and do this query, it’s getting the live data as it is right at that moment. I’m specifying here to do a SQL query and get back 100,000 rows. So it’s going to go out and do the query, which the query itself will be sub-second. And then it’s going to transfer all of that data over the wire, over my slow hotel internet connection here. It’s going to load it into an Arrow table. So then I will have a table. Once I have that table, I can query that table individually. I have all of that data that’s already in a columnar table format that I can do so much with Arrow. Then I’m going to convert that table to a pandas data frame because a lot of our data scientists use pandas. It’s a great tool for working with data. And so I’ll show how fast all of that happens, and how they are ready to start working with the data. So I’ll do the query here. Again, the query itself sub-second. The rest of the time here is downloading all that, and having it available in an Arrow table. So that is really fast to take 100,000 lines of high-cardinality data, transfer it over a slow internet connection, competing with Zoom right now at this moment, and making it available. You can see I’m just showing a summary of the results here. It shows the first five and the last five lines of that data.
Gary Fowler: 00:34:03.624 So I did that, the first one, was via InfluxQL. I’m going to do the same thing SQL. You can see that you can use the language of your choice to do this. The difference, really, when using the Arrow library, on whether you’re using InfluxQL or whether you’re doing query, is this one parameter. We’re saying language equal InfluxQL here. We’re saying language equals SQL here. So easy to pick the one that you want to use. Mix and match, if you want to use InfluxQL for some of your queries. A lot of time for time series, InfluxQL is a little bit easier. For time series data, you can do it. But if you’re more familiar with SQL, easy to do that. And then you can see, you just put in the query in your parameters here. Very easy to do. And then, once you’re done with all that, it’s very easy to just write this to a Parquet file, if you want to. So some data scientists will take that data and they will work with it right in the pandas data frame. Others may say, “Hey, I want to use a third-party tool, maybe a Tableau to work with it.” They can write it to a Parquet file or anything that reads the Parquet format, which is a growing list of applications. But guess what? If you want to use a tool like Tableau, or Grafana, or Apache Superset to work with it, you don’t even need to write any code to get this. InfluxDB 3.0 and InfluxDB Cloud Dedicated, we have direct access to those tools. So you can use Grafana. You can use Tableau. You can use Apache Superset to access that directly from those tools and do that data analysis you’re looking for in real time.
Gary Fowler: 00:35:55.672 We’re adding support for more third-party tools all the time. We’re in the process of testing and certifying PowerBI. So really, the objective is pretty much any tool that can support a JDBC driver, we hope that you’ll be able to use with InfluxDB Cloud Dedicated. And we’re well on our way there with Grafana, Apache Superset, and Tableau. All right. So that is my demo. Thank you. I’ll stop my share. And I’ll hand it back to Balaji.
Balaji Palani: 00:36:30.890 Thank you. Thank you, Gary. That was cool to see the Cloud Dedicated in live action. I’m going to share one final slide, something as a takeaway for you. If you’re wondering, hey, how fast we are, how better we are compared to the older InfluxDB 1.x, or 2, or something, this is what we have observed in our kind of benchmarking labs, which by the way, is becoming available to provide some benchmark tests compared to the older versions, as well as some of the competitors. But you can expect faster time to insights, that is reduce your time by at least 50%. Store more data. This is, if you are storing terabytes of data, paying for that, know that for the same cost, you can store 10X more. Or reduce your cost by at least 70% because now you are using the cloud object store. And then ingest performance — and we have seen this in low cardinality situations, as well as high cardinality solutions. Really, if you have a billion of series, know that we are at least 10X better than what we were before. And we have seen upwards of hundreds of millions of datapoints per second, and sometimes billions. But of course, it depends on the size of your [inaudible], and so on.
Balaji Palani: 00:38:03.123 If you want to see how InfluxDB 3, you want to get a taste of it, sign up for free using our Cloud Serverless. Here’s a link for that. Or you can contact Sales if you are interested in Dedicated. We will set up a cluster for you. We can run a proof of concept. Call our sales team for that. That is about it. And we’re happy to take any questions now.
Caitlin Croft: 00:38:31.169 Awesome. Thank you, guys. All right. So first question is: Is InfluxDB 3.0 automatically updated to users of InfluxDB Cloud with an older version?
Caitlin Croft: 00:38:46.881 The answer to that is no — not right now. We’re not automatically updating users. There are some differences in how the data is handled between the two storage engines. So right now, our thinking is we don’t want to surprise people with that change. And it will be more of an opt-in. We’re working on some tooling to make that upgrade migration easier, easy to do. But right now, the plan is not to automatically update users.
Caitlin Croft: 00:39:18.975 All right. So the next question is, how would you upgrade from 2.0 to 3.0?
Gary Fowler: 00:39:28.324 Yeah. So we do have some instructions on our website, some documentation for how that you can do this. How you can extract your data from 2.0 and ingest it in 3.0. So you can follow that. But also, I’d like to tell you, we do plan on building some additional tooling to help customers do that as well.
Caitlin Croft: 00:39:48.499 What is the approximate time line for InfluxDB 3.0 open source?
Gary Fowler: 00:39:57.798 So we definitely are starting with InfluxDB 3.0 on our commercial offerings. And we started with our Cloud Serverless product that was released at the end of January. And now, our Cloud Dedicated product. And then we will move to our on-premises products in the second half of this year. And then after that, we will look into what we are going to provide for OSS.
Caitlin Croft: 00:40:27.186 A couple people are asking about Fluxlang support with InfluxDB 3.0.
Gary Fowler: 00:40:35.311 Yeah. So InfluxDB 3 is certainly optimized for SQL and InfluxQL. That is going to be your best offerings on InfluxDB Cloud 3. We spent a lot of time with Flux over the last couple of years, and Flux has been a great language for us, but we’re really excited about InfluxQL and SQL in the 3.0 world.
Caitlin Croft: 00:41:08.272 What is —
Balaji Palani: 00:41:08.967 Just to rephrase. Just to add to that. Flux, if you’re using Flux [inaudible], it’s not going away. So it is still supported. We still have InfluxDB Cloud Serverless, where it will continue to work. But yeah, I think if you’re trying to move to Cloud Dedicated, then your best options are InfluxQL and SQL. As Gary mentioned, they are both natively supported, natively optimized, and we know they will perform very well. Flux is, yeah, not optimized to work with the cloud object store.
Caitlin Croft: 00:41:47.580 What is the performance difference between InfluxDB Cloud Serverless and Dedicated?
Balaji Palani: 00:41:53.495 I can take that. So Cloud Serverless, you can think about it as — hey, you want to get started with it quickly. And you don’t know what your workload size is. It’s not large enough. It’s a small size. You’re trying to evaluate. Your best bet would be Cloud Serverless because it allows you to get started. I mean, there is a free tier, but you can quickly convert by, not providing a credit card, and then just convert to pay as you go, meaning pay for what you use, pay [inaudible] pricing and so on. Cloud Dedicated, it’s all of the entire cluster. I mean, there are different sizes, but they are just dedicated to running your workload. So we definitely think that if you have a large enough workload, large in the sense of millions of datapoints per second or something, you definitely should look for Cloud Dedicated. The performance would be much better. And the entire workload, all of the resources for Cloud Dedicated, are just optimized to running your workload. So you don’t have noisy neighbor issues, and so on. That’s how I would like to think about the difference between Serverless and Dedicated.
Caitlin Croft: 00:43:14.006 Okay. What are the plans for InfluxDB 3.0 for Enterprise on-premises?
Gary Fowler: 00:43:21.126 Yeah. So we’re super excited about getting 3.0 into our Enterprise offering. We are frantically working on that right now. So the dedicated offering was our big push in this last quarter, and we were able to get it out and available. And our next big push is to get it for enterprise. So we have a lot of customers that are really excited about it for on-prem, as well. So that’s our focus right now for the second half of the year.
Caitlin Croft: 00:43:55.179 It sounds like the products and engineering team are really busy. All right. So what are the security differences between InfluxDB Cloud Serverless and Dedicated?
Balaji Palani: 00:44:12.806 I can take that as well. So Cloud Serverless and Dedicated, from a data access perspective — it is all their data is encrypted on disks, as well as to the wire. The security difference, the biggest differences come in when you’re looking for, let’s say, a private link, or private connectivity options. We do not have plans to offer private connectivity in Cloud Serverless because it’s a multi-tenant solution. You can’t really connect using private connectivity. But Cloud Dedicated, definitely, we support private link. You can just talk to our sales team, and then once that is set up, we’ll be able to set up private connectivity to AWS PrivateLink, or Azure Private Link. And Google, I forget what is that, but we will be able to support those. Or even Enterprise SSO. All of those are options for Cloud Dedicated.
Caitlin Croft: 00:45:15.913 What is the difference between creating an account directly through the InfluxData website, versus using a cloud platform directly, such as the AWS Marketplace?
Gary Fowler: 00:45:30.360 The main difference there is how you’re billed, whether you’re billed directly from us, or whether you’re billed from the Marketplace. Outside of the billing, the solutions are largely the same. However, we have not rolled out InfluxDB 3.0 to the Marketplace as yet. So if you want InfluxDB 3 on Cloud Serverless, you will want to come directly through the InfluxData website for that.
Caitlin Croft: 00:45:58.650 Right. And some just asked me — oh, go ahead.
Gary Fowler: 00:46:01.306 Let me clarify. With AWS, it is rolled out. So you can get it from the AWS website. But for GCP and Azure, we don’t have it rolled out there yet.
Caitlin Croft: 00:46:13.548 I think Rick answered this question, but someone was asking: Is InfluxDB Cloud Dedicated also a serverless service? It’s still cloud. Right?
Gary Fowler: 00:46:27.596 Yeah. So both Serverless and Dedicated are cloud offerings. So it’s Cloud Serverless, Cloud Dedicated. It’s in the name. And then we’ll have our on-premises products coming out later this year.
Caitlin Croft: 00:46:40.777 Will you be able to migrate InfluxQL Grafana dashboards across to Dedicated? Will this work with the current InfluxQL data source via a Version 1 compatibility API?
Gary Fowler: 00:46:57.070 Yeah. We’re really excited for our InfluxQL customers that have been doing InfluxQL for a long time, and have built up a lot of stuff with Grafana and other tools. And they’ll be able to do those with Cloud Dedicated, as well.
Caitlin Croft: 00:47:13.804 Perfect. So I have a question for all three of you, Balaji, Gary, and Rick. You guys have talked to a lot of customers over the years, and especially with the new product launches that we’ve been working on the last six months or so — what are some tips and tricks that you guys would like to share with the community that you’ve learned along the way, even yourselves, working at the organization?
Rick Spencer 00:47:45.236 I have a bazillion, since I’ve been writing a lot of programs with it. I’ve written a website that collects and stores time series data in InfluxDB. There is a new community Python library, I think it’s called pyinflux3. It’s very clean API. It’s very nice to use. So that would be my first tip is, if you’re writing Python, to go participate in that community. It’s not an official, supported thing, but kind of actually makes it more fun to me because you can actually make pull requests and etc. And the other thing is that there is a tool called Plotly that does any kind of visualization that you could possibly imagine, and it works natively with Arrow. So the combination of the Python client library, and Plotly, and Jupyter Notebooks means that you can do all kinds of really interesting dashboarding and analysis. And because it’s in Python, you can do whatever you want. You can bring in data from anywhere, send data anywhere. So that’s my main tip and trick. Jupyter plus the new Python, the community Python library plus Plotly, you will be able to do anything you want.
Caitlin Croft: 00:49:21.676 And Gary, what about you? I know you talk to a lot of customers in your role. Any tips and tricks that kind of stand out that you wish customers knew?
Gary Fowler: 00:49:31.907 Yeah. I think that, for some data scientists and developers, they can think a little bit differently now. I was talking to a friend of mine that is a data scientist, and I was asking about the process that he goes through to get data and do his analysis on it. And he talked about how he’s going to wait for a batch job to copy data into his data lake. And so it might be the next day before the previous day’s data is available. Then he goes through and does the query for it. It downloads it and puts it in a local [inaudible] database. Then he queries from it then, and maybe exports it to CSV or something to start working with in Excel or something like that. And the process that he has to go through just to do some simple analysis, for data that is already a data old, was incredible. So I was telling him about what you could do with InfluxDB 3, and he was really impressed. That was partly what drove this demo, because the data scientists get really excited when, within a few seconds, they can have live data within a panda’s data frame that they can start working with. And so I think that’s the thing is, hey, you don’t have to think of things like you used to where you’re gathering old, stale data and working with them. With this, wow, you can have an Arrow table or a data frame live in your application within seconds of the data arriving.
Caitlin Croft: 00:51:07.945 And Balaji, any tips and tricks that you’d like to share?
Balaji Palani: 00:51:15.064 I’ve switched my allegiance to marketing. So my tips and tricks would be to look at our blogs. We are posting a lot of blogs recently from Charles, Jason, even our wonderful DevRel community on how to use InfluxDB. We have a wonderful InfluxDB U. We have updated those courses recently, just recently, with SQL and InfluxQL. So check it out. Those would be my tips and tricks to get started with InfluxDB 3.
Caitlin Croft: 00:51:42.632 I love it. Yeah, it’s awesome having Balaji on the marketing side because he really knows the product. All right. Another question here. Does cloud provide better performance than non-cloud InfluxDB?
Balaji Palani: 00:52:00.430 Go ahead, Gary. I think you want to answer that.
Caitlin Croft: 00:52:05.395 Oh, I think you’re on mute, Gary.
Gary Fowler: 00:52:07.690 Yeah. Does cloud provide better performance than non-cloud InfluxDB? Actually, I’ll let Rick take that one.
Rick Spencer: 00:52:19.736 Well, it’s a little hard to answer because we do have a few versions out there. So I would say if you’re interested in performance, today your best options are InfluxDB Serverless or Dedicated because those are built on the 3.0 base. You may recall we called that project IOx when it was in development. You’re going to get — your InfluxQL queries will go much faster than anywhere else that you might run it. Your ingest will be way more performant. Your best performance would be getting a Dedicated instance because then you can really tune it to your workload. So if performance is important to you, query performance, ingest performance, space on disk, you really do need to go to Serverless or Dedicated. I hope that answers the question. And you can always reach out to me in Slack.
Caitlin Croft: 00:53:23.852 All right. I meant to ask specifically around timestamp performance.
Rick Spencer: 00:53:29.551 Not 100% certain what he means by that. But like I said, I’d be happy to follow up outside of the webinar, to understand his needs.
Caitlin Croft: 00:53:44.584 And I’m just going to toggle the Allow to Talk. So if you do feel comfortable speaking up, you should be able to unmute yourself and expand on your question, if you would like.
Attendee: 00:54:01.523 Hi. No, I think we can talk offline. I just need to get this Slack. I don’t think I’m on the Slack for Rick. That’d be nice. We’re having some issues having all these timestamps configured, and we’re taking data from a Siemens server. Then we have InfluxDB. Then we’re pulling into Grafana. And for the most part, it’s pretty straightforward. It’s pretty good. But we’re missing a couple of data points. We’ve been working with a couple of people from Influx and from Siemens, and Ignition, from Ignition Servers, also. But yeah, it’d be nice to have Rick’s contact.
Rick Spencer: 00:54:51.462 Sure. Yeah. I put it in the webinar chat. My name is Rick Spencer 3 on the Community Slack. And I will answer any questions when he reaches out to me there, so.
Caitlin Croft: 00:55:04.608 Also, we’re in the Slack link. So if anyone who is on this webinar who’s not part of the Community Slack workspace, you can click on that link, sign up for a free account, and find all of us in there. So yeah. It would be awesome to chat with you guys some more. All right. We’ll keep the lines open a little bit more. See if anyone has any more questions. In the meantime, while we see if there’s anymore, if someone wants to sign up for InfluxDB Cloud Dedicated, what’s the best process? Should they reach out to us on the website through the Contact Us, or what’s the best format?
Balaji Palani: 00:55:50.757 Yes. I think they should reach out to us. They can contact us. We’ll just work with the to understand the size of the workload, how big the cluster needs to be, and then we can run a proof of concept with the customer. We in the sense of the sales team. Our wonderful sales team will work with them.
Caitlin Croft: 00:56:09.052 Cool. Yes, the presentation is being recorded. Someone’s asking where it will be upload. So we make it really easy for you guys. You just have to go back in 24 hours and check out the registration page, and it will automatically time over to the recording, as well as the slides. So really easy to find it. Just go back to where you registered for it. And also, everyone here should have my email address, as I think some of the automated emails from Zoom appear as if they come from my email address. So you’re always welcome to email me, and I’m happy to share that link out with you. And I also just want to let you know, with the new version of InfluxDB, it was completely rewritten in Rust. The previous versions were written in Golang. And the team took a huge undertaking and actually rewrote the entire database in Rust. And I’m really excited that we have a webinar in a couple weeks, about a month actually, with Paul Dix and Mrinal from Ockam, which is another open-source security company who also rewrote their tool in Rust. So they went from, I believe, C-sharp to Rust, and we went from Go to Rust. And so it’ll be a really fun, panel discussion between the two of them, discussing why make that change and all that. So I think that will be a really fun presentation, and I think you’ll be able to learn a lot, and understand the theory behind all of the updates that we’ve been doing in the last couple years here at InfluxData.
Caitlin Croft: 00:57:54.653 Thank you, everyone, for joining today’s webinar. Lastly, I just want to do a little plug. If any of you are in the Seattle area and are going to Microsoft Build, Balaji is actually there. And I believe Rick is actually there, too, as well. So if you’re going to Microsoft Build, go find the InfluxDB booth. Everyone there is super friendly. Go ask some questions. I know Balaji and Rick would love to chat with you guys. Anything else that you guys would like to add, or any last-minute words of wisdom that any of you would like to chime in on?
Balaji Palani: 00:58:35.255 No. Thank you for having us. Thanks for listening.
Caitlin Croft: 00:58:38.789 Awesome. Thank you all.
Gary Fowler: 00:58:40.292 Thank you very much.
Caitlin Croft: 00:58:41.877 Thank you all. And once again, this has been recorded and will be made available by tomorrow morning. Thank you.
[/et_pb_toggle]
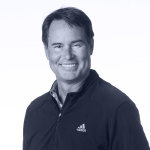
Gary Fowler
VP of Products, InfluxData
Gary Fowler is VP of Product at InfluxData. Gary has nearly three decades of experience in product management, program management, software engineering and sales engineering. He previously held Vice President roles in Product and Engineering at iPass, Airborne Interactive, and Lilee Systems. Gary resides in Holualoa, Hawaii.

Balaji Palani
VP, Product Marketing, InfluxData
Balaji Palani is InfluxData’s Vice President of Product Marketing, overseeing the company’s product messaging and technical marketing. Balaji has an extensive background in monitoring, observability and developer technologies and bringing them to market. He previously served as InfluxData’s Senior Director of Product Management, and before that, held Product Management and Engineering positions at BMC, HP, and Mercury.