How EnerKey Using InfluxDB Saves Customers Millions by Detecting Energy Usage Fluctuations Based on Weather and Geospatial Data
Session date: May 20, 2020 08:00am (Pacific Time)
EnerKey is a market leader in the energy data management industry. By collecting data from IoT sensors and meters, they help retailers, schools and local governments understand their energy consumption levels better through their analytics platform. Their clients have become data-driven by being able to correlate data across multiple locations.
In this webinar, Martti Kontula will dive into:
- EnerKey's strategy for reducing energy consumption
- How using a time series database enhances EnerKey's competitive advantage
- Their approach to using machine learning to help their customers forecast and optimize operations
Watch the Webinar
Watch the webinar “How EnerKey Using InfluxDB Saves Customers Millions by Detecting Energy Usage Fluctuations Based on Weather and Geospatial Data” by filling out the form and clicking on the Watch Webinar button on the right. This will open the recording.
[et_pb_toggle _builder_version=”3.17.6” title=”Transcript” title_font_size=”26” border_width_all=”0px” border_width_bottom=”1px” module_class=”transcript-toggle” closed_toggle_background_color=”rgba(255,255,255,0)”]
Here is an unedited transcript of the webinar “How EnerKey Using InfluxDB Saves Customers Millions by Detecting Energy Usage Fluctuations Based on Weather and Geospatial Data”. This is provided for those who prefer to read than watch the webinar. Please note that the transcript is raw. We apologize for any transcribing errors.
Speakers:
-
- Caitlin Croft: Customer Marketing Manager, InfluxData
- Martti Kontula: Chief Technology Officer, EnerKey
Caitlin Croft: 00:00:04.595 Hello, everyone. Welcome again to today’s webinar. My name is Caitlin Croft. I am part of the team here at InfluxData. And I’m super excited today, as we have Martti Kontula, who is the CTO at EnerKey, and he will be presenting on how EnerKey uses InfluxDB. So without further ado, I will hand it off to Martti.
Martti Kontula: 00:00:32.772 Thank you, Caitlin, for the invitation to present this webinar I’m super excited to do. So as a big fan of InfluxDB, our agenda here is that we are going to have a look at the company in general and have a look at the customer’s problem and how we are solving it, and then have a brief look at the product history and the timeline which ended up selecting the InfluxDB enterprise, and also how did we made the decision and comparisons. Then we look at our data acquisition architecture, as it differs quite a lot from a traditional thick stack. And after that, we can dive into actual interesting parts where we added machine learning to the mix and some studies to analyze this and made EnerKey super clever. And, of course, then afterwards the Q&A will follow.
Martti Kontula: 00:01:46.062 So where we are. We are located in Finland. And as you can see from the map, we are here at top of the world, far away in Northern Europe. Finland’s a small country of 5.5 million population. We use euro as a currency and speak Finnish and Swedish for historic reasons. It’s [inaudible] in that specific area in Canada, and so some people on the coast on Finland just can’t decide whether they want to be Swedish, Finnish, or both. And please bear with me. English is actually my third language that I speak, and I might use some words that you will find funny.
Martti Kontula: 00:02:30.487 Right. Our mission statement is sustainability and savings. Our product helps property owners measure their energy consumption in various quantities and discover possibilities for reducing those figures. Our product for support for fulfilling ISO 50001 and 40001 certificate requirements. And by reducing the [inaudible] and excess consumptions [inaudible] save money, and the environment and climate are happier, and therefore fighting the climate change in the front line.
Martti Kontula: 00:03:14.479 Here’s some information about EnerKey in numbers. We have roughly six million revenue. Back in 2019, our [inaudible] was working in software development and energy management [specialist?]. We are owned by a [inaudible] fund and active management. And in the system, current we have way over 100,000 metering points in - actually, this number is wrong. It’s closer to 20,000 properties at the moment. And we are the first software as a service company to combine sustainability in its management, at least here in Europe. And one of the key satisfactors is that they have over 80 different ready-made integrations to different building automation systems, IoT interfaces, and other metering device systems. So there’s a big change that when we come up with a new customer case, we already have the technical integration in place, that we can feed the data to our platform. And by using our product, the best customer cases have reached up to 30% annual savings in their energy bills, so that’s quite a lot.
Martti Kontula: 00:04:47.701 Here’s our current spread across Europe. We mostly operate in Finland, Sweden, Norway, and Denmark; Russia; and we have some single industrial sites monitored in the Baltic countries, Poland, and Slovenia. And currently our target is to highly extend our presence in Sweden and Norway and then make a turn south and enter the German and Dutch markets. And the reason for this is in the maturity of digitalization and analysis [inaudible] infrastructure. In the Nordic countries, in addition to Germany and the Netherlands, clearly had [inaudible] of the Europe in this race. And of course we do support, like I say, manually read devices for electricity or water, but our aim is to get the data automatically from different sources to the platform. And these kind of manual readings are quite slow and require lots of manual work.
Martti Kontula: 00:06:06.771 The product currently supports over 90 different types of energy and other quantities, including [inaudible] types of dissipating or [distant?] cooling - just letting it cool down. Electricity of course, with many interesting soft-metering types. We’re also able to mix and match products and commodities side-by-side with other consumptions, to provide insights on how changes in production quantities alter the consumptions in energy. And lately we’ve also added environmental measurements such as inside air quality, humidity, etc. to the mix. And on the sustainability side, you’ll see accurately calculated conversion factors, that are able to present used amounts of different energies as carbon dioxide emissions or costs. And when you add transport fuels and different waste types as also added value in sustainability reporting and development towards reduced environmental impact on our cost on this side.
Martti Kontula: 00:07:20.459 And as said, the connectivity is our competitive edge. And I’m quite proud to say that if we don’t have it, we’ll build it. So this very agile and forward-looking attitude has a resulted in this last amount of ready-made integrations. And we have lots of data, and we expose it back to our customer by an open API. And some customers have a big - information screens, so housing buildings or in a little base of office buildings showing what’s the trend of energy consumption, and also some [inaudible] within the customer’s own systems.
Martti Kontula: 00:08:19.081 We originally built this EnerKey product for [inaudible] sales. But this Powered by EnerKey was added last year. And it’s a white-label version of EnerKey, so we can give it for the energy companies so that they can offer it to their customers with their own brand look and feel, and logos of course. And the new tools, which include the machine learning and artificial intelligence, are also part of the offering in this Powered by EnerKey version. So basically it’s, of course, just the same software as a service product running in a cloud with such different brand images and a multi [inaudible] divided data in the lower layers.
Martti Kontula: 00:09:18.523 And these logos are from the Nordic countries and most likely don’t sell a lot of story for you guys abroad, but basically our customer segments include the retail, large property owners, industry, and the public sector, with lots of building and housing and office complexes. And here is a good reference slide. Kesko is the largest retailer in Finland, and during an eight-year partnership with EnerKey they’ve reached a level of 500 euros in annual savings in energy consumption, and that’s a quite big number. Somebody would even say beautiful. And Kesko was the most sustainable retail company status, from a group of 100 most sustainable corporations worldwide, last year. So it’s a quite nice reference. And HELEN is a major energy company operating in the capital city area of Helsinki, and they were the first Powered by EnerKey case we had in production.
Martti Kontula: 00:10:42.837 And in moving on, what we are actually doing - the problem we are solving is our customers’ data. And for example, property portfolio owners - their buildings are distributed across Finland, across Sweden, Norway. And the energy business is an old business area, and traditionally divide into small local companies. So when you have a thousand different properties spread out through the country, it’s very likely that you have your energy consumption data in highly distributed way. And this leads to a problem, which is called energy management by Xcel. And believe me, I have nothing against Excel when it’s correctly used, but for property portfolio sustainability and energy management, it’s certainly not a correct place for Excel.
Martti Kontula: 00:11:46.006 So we are doing the dirty work. We are integrating all their [energy?] consumption quantities on a single platform, in a clean and harmless presentation. And we also take care of many additional calculations and analysis, based on the time series on behalf of the customers. And InfluxDB is massively used to store billions and billions of data points because we keep our one-hour results and consumption data in the system, for six years and one-month aggregations, up to 12 years. And I’m quite aware that some people operate InfluxDB in a millisecond or even a nanosecond of resolution, for a short period of time. But we basically have the same use case, the same amount of data, but it’s so spread out in a much, much longer timeline. And InfluxDB doesn’t care. It’s about time series data, and that’s where it shines.
Martti Kontula: 00:12:52.848 And let’s take a brief look back in the history, at this point. The actual EnerKey product was start already back in 1995, when Finland won the first ice hockey world championships. That was a great year in many ways. And this SQL server was used to store the data. And, well, we all know how well an SQL server handles time-service data, and that resulted in impeded capability in a single database, and that the old EnerKey used quite, quite interesting manual process to add a next sequential database with an increasing number. And that’s not quite of an architecture of miracle; it’s a mess as [inaudible] system. And this old EnerKey reached its end of production in 2014, when the new EnerKey development was started. But also, this new EnerKey development started slightly on the wrong foot, and that’s because SQL servers were still used for the time-service data storage. And as we know, it’s not no good in that purpose.
Martti Kontula: 00:14:27.845 So here’s how it basically went. In the first half of 2016, EnerKey development faced serious performance problems with SQL storage. And I joined the company in October of the same year, and my first task was to figure out a better solution for time series data storage and handling. In December, the same year, I had the first talks with InfluxData salespeople, and we used most of the 2017 to test-drive the open-source solution for functional [inaudible]. And of course we did lots of product development in the same time, and of course, not all of the time was used for database comparison.
Martti Kontula: 00:15:22.481 But anyway, the results were pretty good with InfluxDB, and we upgraded to buy the enterprise version at the end of 2017. And the next year, we used to run a hybrid solution with all data storage side-by-side with the new InfluxDB. It was a continuous migration, the [inaudible] today that we all face. And it was a handful of work. And of course, in the software development business, everything doesn’t always go like you planned it to go. We accepted a major migration during the new year of 2019, and our system uses Azure functions and Azure service bus now for the data communication. And the functions responsible for writing the data to InfluxDB were using a consumption-based plan. And that plan comes with an automatic scale, and the scale did not have an upper limit.
Martti Kontula: 00:16:31.942 So what happened - we ended up performing a DDoS attack on our own system, with over 100 rapid-firing function instances, which brought the DB down. And a quite heavy rollback fallout during [inaudible] of mid-2019. That was like quite a busy days and nights. But anyway, in the Q3 of 2019 we finally managed to move over to the latest InfluxDB and everything has been like happily ever after since then. So it’s not more so like a short decision to, “Hey, let’s go to the InfluxData,” but instead it was spread over a few years in development and testing and integration and migrating to old data. But now everything is up and running really nicely and smoothly.
Martti Kontula: 00:17:39.474 And here’s some thoughts of what we were thinking about when we decided to go with the InfluxDB. Of course, the high data ingestion rate is a fantastic feature, and in the meantime was - it’s [inaudible] and it’s also able to output the data for the queries. And group write time is the fantastic feature when you are working with time series data. And I’d say that our group [inaudible], which are based in the time, have been invaluable for us in terms of saved development time for other important things. Of course, it’s possible to write the same kind of functionality yourself by a code, but all of that time was saved for other product development.
Martti Kontula: 00:18:41.346 And for example, some IoT devices, when they send the data in, it’s not coming on the exact hour, minute, or second boundary. Instead it’s off by a fraction of a unit. And with the group write time, we’re able to get rid of this abnormalities with ease; just a group write time one hour, and it’s always sharp. And we also use a lot of linear interpolation to fill the gaps in the occasional missing data points. And when the data is cumulative, it doesn’t matter if you’re missing a few points here and there; you can just linear interpolate it, so consistent time series.
Martti Kontula: 00:19:27.347 Also the natural upsert, which I mean, that - when [inaudible] an exact time, once the data set doesn’t have it, it performs an insert. And when exactly the same [inaudible] and exactly the same time it send again, it performs an update, hence the term “upsert”. I know, of course, in one occasion this feature backfired a bit, where one of the new guys in the development team was not 100% aware of this nature of this functionality, and we ended up having some duplicated data, which also of course was later on cleaned up.
Martti Kontula: 00:20:14.273 And we looked at some other alternatives as well. One solution was based on the PostgreSQL, but that use - quite bizarre, table [inaudible] place in a program code and ran some new data for [inaudible]. The system created new tables here and there and somehow we are closely keeping track of the process. And to be honest, I was mostly impressed that that thing actually worked. [inaudible] cheap, and it can perform quite high I/O with correct time series oriented schema. And the same story goes for Cassandra as well.
Martti Kontula: 00:20:59.515 And the common denominator for both of these alternatives was the lack of native time-handling, and I would say that this native time-handling was the biggest single decisive factor for us to choose InfluxDB as a time series database. And of course the coin always has the other side as well. The lack of natural months is and was a bit annoying, since this is a time series database, and one would think that the database that handles time would know about a calendar. But as we all know, with InfluxDB, this was not the case with the 1.x series. But as annoying as it was, it was quite easily solved by using the calculation functions outside the InfluxDB, and aggregation was performed by our own program code.
Martti Kontula: 00:21:57.802 And the other thing is that at the time of the selection, in 2017, the cloud offering was quite limited. And going for the on-prem, or basically the virtual machines, not Azure - the on-premises was the only way to go when you wanted to have a cluster. So why did we go for Enterprise? Well, the answer is, of course, the cluster, because our data is - not some time in metrics about the CPU and memory level or a water level in Santa Monica, but this is real business data with a long-lasting value. And in some cases, the additional services that they have built on top of this [inaudible] product, they include invoicing and invoiced additions - new additions by [inaudible] the business rules. Like in a shopping mall, the energy consumption may be divided by areas that the smaller retail shop is consuming less energy based on its area, or something like that.
Martti Kontula: 00:23:24.637 And we need it. For this business data, we need reliable storage, and we really want to go for some kind of clever replicating process with the open-source database. But we chose the enterprise for its cluster-like behavior. The other thing was the support. We realized that we are entering a new area of technology, which definitely will bring along some surprises. And although we did quite a lot of testing and learning during 2017, the back of the end of the Enterprise support was a mandatory thing to have, and it has also proven to be highly valuable - I’d almost say invaluable - for us, because in this curious [inaudible] case from the early 2019, we spotted that there was an increasing load in the cluster CPU, which was unexplainable. And we called Enterprise support for help, and they went through our logs and spotted a rogue query that was eating up a lot of CPU.
Martti Kontula: 00:24:48.009 And as I said, we are performing quality assurance for the data as well. And when new data are in this platform, we need to check that the time series is consistent. And the query which was looking for the last existing data point - [inaudible] query without a lower limit. So when the data accumulated, the poor database was doing a lot of work, because it was [inaudible] way too long timeline for the last existing data point. And we replaced this query by this kind of exponential widening sequential search. So it takes the first 24 hours, then the next 48 hours, then one week, one month, three months, a year. And the most likely hit for the last existing data points was always found, but in most cases in the most recent 24 or 48 hours. And this flattened the CPU load all the way back to the 20% area that it’s supposed to be with the data load that we have in the system. So big high five to the Enterprise support. Those guys did a fantastic job to help us mitigate this issue.
Martti Kontula: 00:26:19.722 Okay. I know this looks like a - from some kind of science fiction movie, but it’s an actual thing. And we are using a Hangfire Scheduler to pull the data from various data sources from public internet or through VPN tunnels. The Hangfire is instructing its Azure functions. You go ahead and pull the data in any format in one of the 80 different integration formats. It’s pulling the data from the data sources, making an in-place conversion to our common data format. So we are thinking like a thick wall where the reader functions, input comes to the exterior side, and it’s [inaudible] conversion and providing our service bus in the [inaudible] side with the common data format. And then the data is easy to handle because it’s harmonized to a single data format. So it’s a time series with a time stamp, a measuring code, ID, and a value. And the value can, of course, be an hourly consumption or a cumulative data that accumulates indefinitely to the future.
Martti Kontula: 00:27:50.580 So we push the data to our service bus. And once it’s on the bus it’s safe. It’s a quite large system and performs a lot of operations on the data. And sometimes we receive some data which just fails to calculate - there’s some other glitches; maybe somebody even brought a bug. It happens in software development, I’ve heard. And in these cases some kind of - a calculation fails - the data is not lost. It’s placed in a data [inaudible] queue, [DA?] queue. And we have an ongoing process which from time to time pushes the [DA?] queue back to the service bus. And when we have this possible box fixed, the calculations and data operations succeed, and the data is fit the system again. So it was sort of mandatory thing to do. We just cannot lose the data. And the service bus is a very scalable, robust, and reliable - like a mid-term storage.
Martti Kontula: 00:29:10.061 And we perform the automatic quality assurance functions. We see if there is faults, for example, gaps. There might be positive or negative spikes. And if - and we have this heuristics in place which try to detect the spikes and flatten them out, because we know that if there [is a?] normal consumption of 1 kilowatt-hour, 2 kilowatt-hours, and then suddenly there’s a 100K spike - but it’s not really consumption; it must be flattened. And we have different tolerances to decide which is a spike and what is not.
Martti Kontula: 00:29:50.538 And we also store the original raw data in different storage. So, because we perform this automatic quality assurance, we sometimes might be requested to, “What was the original data like? And what did you do with it?” So first storage is an InfluxDB. And after the quality assurance teams are done, the data is forwarded to our calculation functions which perform normalization of the temperatures so that you can compare facilities and properties with different temperature areas. For example, Finland’s a long country, about 1,350,000-something kilometers. And of course up in the north it’s colder, and in the south it’s warmer. And [inaudible] perform this normalization, which is based on how much heating energy is required. So the heating energy part is [laid?] out and then actual consumption in electricity is comparable. And we also built a natural month aggregation in some other business aggregations as well.
Martti Kontula: 00:31:12.448 So I mentioned that it’s quite different from traditional tech stack. It is. And we all know that the Telegraf is a fine tool with an extensible working architecture. But since we pull out the data from the business systems, such as building automations, we have quite little control or no control whether we can install a Telegraf plugin in those systems. And in most cases there’s already an REST or SOAP interface that we can use to put the data in our system. And since it’s not metrics - it’s business data - the Telegraf is sort of ruled out.
Martti Kontula: 00:31:57.885 We use InfluxDB for the business takeoff course, and we use it in conjunction with Grafana for monitoring production environment. And we have lots of others for various parts of the production environment. And we have quite nice production [super?] view called Dashboard. And on the next slides I will show you a few screens of that. Initially we planned to use Capacitor for the Q&A functions, the quality assurance. But the idea was then replaced by the Service Bus solution. And the Azure functions written in C# and the Microsoft languages and technologies fitted our general architecture and technology set better. So basically it was possible, but for us our tech stack was better off with the functions and the Service Bus solution.
Martti Kontula: 00:33:04.165 Okay. Here we are seeing - we are monitoring the Azure [up?] service plans, where the CPU and memory are going, and we have [consequent orders?] that the CPU and memory should not go away to 5%, or if it does it’ll send us a message to our teams that, “Hey. I’m behaving badly here. Somebody please take a look.” And there’s some stuff in the [DA queue?] that must be emptied at some point. But the trend is quite stable. It’s not increasing, so. Probably it’s got some old junk.
Martti Kontula: 00:33:59.346 Also, we are monitoring our API performance. There are some spikes yesterday. It’s flattening, maybe unreadable. Also they’re monitoring how many actual errors that happens in terms of API, HTTP 500s. [That’d sort of be?] too many. And I’ve got an alert level for that as well. How many total requests are going through different APIs. And it’s 25,000. [inaudible] are quite normal. That’s looking good. And of course there’s the active [inaudible] count in our service bus. And the spike areas showed [those?] busy hours and a lot of data is flowing in from the [reading?] functions.
Martti Kontula: 00:34:54.819 The Influx cluster itself is monitorable, of course. Memory use and CPU use. And alert and alarms for that as well. And then some other database figures. This is showing our metadata, reporting services, DT levels. So it’s an Azure SQL. And when it’s running below its 85% [inaudible], we are happy. And some figures from the [release?] cache, as well. It should be somewhere under 1-gigabyte level, and that looks good.
Martti Kontula: 00:35:48.664 Right. So we [now get a?] very good [software and?] [inaudible] management solution. And it is a really good platform for that. The data is secure; it’s fast; it’s robust. We have the integrations for the data flowing in and going out. We are an open API. And so basically it’s good. But the challenges that we have [confronting?] us are mostly facility, property, and real estate management systems, which comes with some basic sustainability energy management features. So we need something that the others couldn’t do. And the solution was to add some machine learning models to pinpoint energy consumption profiles that are misbehaving in some way.
Martti Kontula: 00:36:46.308 And how we did this - first, what we had first, for starters. We had a good quality data consumption data for main metering points and some sub-metering points with interesting energy quantities. For example, the main energy consumption and then sub-metered heating or cooling energy consumption. And also we pulled out good-quality [inaudible] winter data, basically weather, temperature, wind, and sun radiation. And we mixed that with good-quality meter data from buildings like cross-areas volumes, [allocation?] building, [year of?] building, type, opening hours, when the building’s occupied, when it’s not. And we would feed all of these things into machine learning models. And [instead?] we are running [DASH?] here. And we are mostly a Microsoft house. But in this case our analysts suggested that we would be better off by using [inaudible] Notebooks and running them in a Linux [inaudible] end-docker. And so machine learning models that this Linux [inaudible] ran - it’s fetching the data from the InfluxDB and it’s fetching the metadata from the Azure as well. And the result is a cloud-deployed, reusable product with can be used for analysis in ad hoc or continuous work [stack?] mode.
Martti Kontula: 00:38:30.042 And on the next slide are some results from one initial machine learning model that our analysts built. And this analysis checks if the time program for building or installation is active and functioning properly or not. And in this case the result was that the time program was not active when it could have been. And in short it was wasting energy. And in this case the savings opportunity was up to 300 euros per month during the cold months, and it’s also 1500 euros per year, for just one single building. And if you imagine how a - 100, 200 buildings in a portfolio and 25% of them are misbehaving, there’s a quite huge opportunity for savings. And of course, well, you convert that consumed energy, for example, the CO2, we are seeing the environmental impact.
Martti Kontula: 00:39:47.377 This model was being thought how the time program should function in terms of energy consumption when it’s active, it’s reducing to installation levels, and it’s using less energy. And in this case this time program is misbehaving badly. The red color shows that it’s pretty much [inaudible] active at all. And these gray areas are part of the model, because this part of our building is not used during the weekend. So those gray areas are not inspected. And the report shows that the facility has 0 days of time program active out of the 107 inspected days, which brings the data up to a level of statistical significance.
Martti Kontula: 00:40:48.229 Here’s some further analysis of the case. The blue dots and the blue recreation line shows how the building reacts to changes in outside temperature, when it changes and the building is occupied. Most likely this is some kind of an office building. The red dots and the recreation line shows the same when the building is unoccupied. So the people are the building. And basically if the time program is correctly configured the energy consumption should be something which is presented here, with the orange dots and the recreation line. And those unoccupied and occupied lines should not cross, or otherwise based on this model the time program is misbehaving.
Martti Kontula: 00:41:43.451 And the result is that the light green area over here shows the hours when the building is occupied. The gray areas are the unoccupied hours. And with two additional ventilation windows before the occupation and after the occupation, [inaudible] their savings are presented here with the orange line where the ventilation could be tuned down. And [inaudible] to their savings comes from the darker green areas. And this resulted in a 300-euro savings per month during the colder months in Finland.
Martti Kontula: 00:42:33.029 And it’s one other example. Here we did some analysis for the cooling energy with the sub-metered consumption in a certain size grocery stores of a retail chain. And this analysis was based on a slope of energy consumption increasement when the temperature outside rises by one degree. And the blue bars over here shows the slope of the store, measured from the sub-metered cooling energy, and the yellow bars on the right side show the same for measurement done from the main electricity meter. And the results are surprisingly similar. So the cooling energy affects a lot of the actual total energy consumption. And the correlation coefficient tells how good the estimate is. And these red dots over here shows the facility where the slope is over 0.7. And, as we can see over here, there’s lots of facilities in the comparison. And we are looking at the high end, when the slope is the highest, which means that the store’s cooling energy consumption highly correlates with the changes in the outside temperature.
Martti Kontula: 00:44:24.728 So one medium-sized [process?] was spotted. It stood out from the results. And slope increase was substantially steeper when the temperatures exceeded 24 Celsius. And please note that 24 Celsius is [culturally warmer there?] in Finland. It’s quite cold here in general. And after investigation by professionals on site, they found that the condensing increment at the roof for the cooling devices was undersized. And it’s also replaced by adequately sized ones, and excess cooling and its consumption did not occur anymore. So of course we didn’t know this. We are only able to point out that this building is behaving differently than the other buildings in similar portfolio. And then the actual professionals who understand something about buildings and cooling and equipment went in for investigations.
Martti Kontula: 00:45:36.156 And in that bombshell, it’s time to say thank you all for listening, and for InfluxData and Caitlin for advising to present this webinar. And before I’ll hand it over to Caitlin for the questions and answers, Caitlin asked me to present this advertisement for the InfluxDays London virtual meeting. And I’m going to be present on both of these venues. Right, Caitlin. That’s all from me. Over to you.
Caitlin Croft: 00:46:12.592 Okay. Thank you, Martti. This is fantastic. So before we got into the Q&A section, just want to remind everyone again about InfluxDays London, which is coming up. So we have our hands-on Flux training on June 8th and 9th. And then later on in the month we have the InfluxDays, which will of course be virtual. There’s some really fantastic presentations all lined up from other customers, as well as internal folks as well. So be sure to RSVP. It’ll be a great event. We’re super excited to have everyone there.
Caitlin Croft: 00:46:50.734 So we got a few questions here, the first one being, “How do you show energy consumption per day in Grafana or per week, weekly - sorry, per day, weekly, or even monthly?” The energy value is the total consumption from the meter, correct?
Martti Kontula: 00:47:14.371 Okay. As I said, we support both the main metering points - for example, a building, traditionally it has one single in-point from the energy company. Then we fetch the sub-metering data usually from the building automation systems, and we build a metering device tree which shows that, “Okay, this is the total consumption and it’s divided to smaller totals.” For example, in shopping malls we might have a sub-metering for lights, the inside and outside. Maybe there’s some electric vehicle charging points. They are usually also sub-metered. And as I told you about the cooling and heating energy, those devices are usually also sub-metered. And we have a metering point tree which our users can drill in to a more precise information about the consumption. And we don’t use Grafana for our end customers. Instead we have our own modern single [database?] application, which is showing all the resourcations and [inaudible]. So I hope this answers the question.
Caitlin Croft: 00:48:59.772 Great. The next question is, “What do you use to perform calculations and store your results in a database?”
Martti Kontula: 00:49:10.582 Yes. The calculations are some [inaudible] programming written in C#. And we use different measurements for the aggregated values. So our late-data model in the data business is quite simple. It’s conscious of time stamp, the value, and the metering. And unique identifier for the metering device or the metering point. Then all of the data, whether it was electricity, water consumption, [inaudible] heating, it’s just time series. And the metadata storage in Azure SQL then figures out which metering points means what. So the calculations for the aggregates, for example, natural month, are performed outside InfluxDB and then stored in separate measurements, which sound like 1 times 10 per month in the beginning of the month, and then it has a value which is the aggregate for that month. So this is how we solve the natural month problem.
Caitlin Croft: 00:50:36.479 Great. Why did you choose Azure SQL instead of an on-prem SQL?
Martti Kontula: 00:50:45.895 That’s a great question. Azure SQL at some point, back in 2017 perhaps - [we know they studied?] - they’re actually exceeding the performance of an on-prem SQL server running on a legacy hardware. And since everything in our platform is in Azure, with the Azure SQL we had lower latencies in the data transfers from our back-end APIs and the database. So we are aiming to be cloud-native in all aspects. And that’s the reason for the Azure SQL instead of an on-prem one. And another thing is, of course, to enable to scale up while just pulling the slider with your mouse. That’s your control portal. So it’s modern nowadays solution.
Caitlin Croft: 00:51:55.262 Perfect. And is Grafana in the cloud as well?
Martti Kontula: 00:52:00.217 Yeah. Grafana is running on a virtual Linux machine in the cloud as well.
Caitlin Croft: 00:52:09.786 And which version of InfluxDB are you using? And are you using Flux query?
Martti Kontula: 00:52:18.467 Good question. We are running currently - and we are still in the 1.x series and running the 1.x.0 at the moment. And as I told you about the selection process, I’d have loved to go with the cloud offering of InfluxData since day one. But since it was quite limited back in 2017, we had to use more calculation power from the virtual machines and build the cluster ourselves. And we are aiming of entering the cloud 2.0 solution when it comes available next year. I’ve heard a rumor that it’s coming. And Flux is something that we definitely look at, because it has ability to mix different data sources. And, for example, the [accuracy of the?] calculations could be performed with Flux, as it can fetch the data from the Azure SQL as well as from the InfluxDB. So that’s definitely something that we are looking forward in the near future.
Caitlin Croft: 00:53:30.015 Great. And just to confirm, everything that you’re doing, is it in Azure cloud?
Martti Kontula: 00:53:36.711 Yes. Well, 99%, because some customers are a bit sensitive about their data transfers, and they want to transfer the data for us inside a VPN tunnel. And those tunnels are in some cases terminated now on-prem data center. But all in all that’s minor thing. So 99% we are running in Azure cloud.
Caitlin Croft: 00:54:09.186 Thank you so much, Martti. There were some fantastic questions in there. So just to let everyone know, since I know a couple people were asking, this webinar has been recorded, and it will be available for replay later today. So just be sure to check back tomorrow. The registration link will take you to the recording tomorrow. So obviously since you registered for the webinar you’ll be able to watch the replay as well. If anyone has any more questions, feel free to post them in the Q&A box or even the chat box. We are monitoring both.
Caitlin Croft: 00:54:50.718 Martti, this was great. This is basically the third time that I’ve heard you tell EnerKey’s story. And every time you tell it I learn something new. So it was a fantastic webinar.
Martti Kontula: 00:55:04.981 I’m super excited and glad that you enjoyed it. And as I told you in the introduction, I’m a huge fan of the InfluxData and InfluxDB. It has been a key success factor for us, with all its abilities in time-service data handling. So if anyone out there is considering using InfluxDB for time-service, believe me, it’s worth it.
Caitlin Croft: 00:55:36.314 I’m so glad to hear it. We did get another question. Someone’s asking if we can share the Q&A log. Unfortunately we don’t share that. But the recording will include all of the questions, so you will be able to go back and find the questions as well as Martti’s answers. Well, that concludes today’s webinar. Thank you, everyone, for joining. I hope you have a good rest of your day. I hope to see you at InfluxDays, the Flux training, and even maybe the community office hours which start in about an hour. Thank you very much, everyone. I hope you have a good day. Bye.
Martti Kontula: 00:56:17.432 Bye.
[/et_pb_toggle]
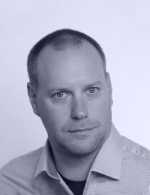
Martti Kontula
Chief Technology Officer, EnerKey
Martti Kontula is the Chief Technology Officer at EnerKey. He joined the company then known as Enegia three and half years ago as an Enterprise Architect. Before joining Enegia/EnerKey he worked at software/project delivery companies for nearly 20 years as a developer, a technical lead, an architect or as a team leader. This experience has been invaluable, especially now that he's more likely on the buyer's side of the table, EnerKey uses some subcontracting to help build their products. Before studying information technology at Jyväskylä University, he started his early career back in the 90's when the internet was all new and full of opportunities. He remembers a curious technology stack where an online store was selling gospel CD's O'Reilly books for CGI programming in C. They were sold out at the local shop and he had to adapt them for the PERL version. He's been reading and writing regexps in his dreams ever since. He lives in a small community called Muurame next to the city of Jyväskylä with his wife. He's a cycling enthusiastic, passionate golfer and ski instructor when he has some time off work.