Discover How IBM Uses InfluxDB and Grafana to Help Clients Monitor Large Production Servers and IBM Benchmark Centers
Session Date: Aug 13, 2020
Time: 8:00am (PT) | 3:00pm (GMT)
IBM has been innovating to create new products for its clients and the world for over a century. Customers look to IBM Power Systems to address their hybrid multicloud infrastructure needs. Larger POWER9 servers can have up to 192 CPU cores, 64 TB of memory, dozens of PB of SAN storage, and typically run a mixture of AIX (UNIX) and Enterprise Linux (RHEL or SLES) workloads. As part of its sales process, IBM is always benchmarking its new hardware and software which clients use to monitor their systems. Discover how IBM and its clients are using InfluxDB and Grafana to collect, store and visualize performance data, which is used to monitor and tune for peak performance in ever-changing workload environments.
Join this webinar featuring Nigel Griffiths from IBM, Ronald McCollam from Grafana Labs, and Russ Savage from InfluxData to learn how you can use InfluxDB and Grafana to improve large production workloads. Learn about the latest product updates from InfluxData and Grafana Labs.
Watch the Webinar
Watch the webinar “Discover How IBM Uses InfluxDB and Grafana to Help Clients Monitor Large Production Servers and IBM Benchmark Centers” by filling out the form and clicking on the Watch Webinar button on the right. This will open the recording.
[et_pb_toggle _builder_version=”3.17.6” title=”Transcript” title_font_size=”26” border_width_all=”0px” border_width_bottom=”1px” module_class=”transcript-toggle” closed_toggle_background_color=”rgba(255,255,255,0)”]
Here is an unedited transcript of the webinar “Discover How IBM Uses InfluxDB and Grafana to Help Clients Monitor Large Production Servers and IBM Benchmark Centers”. This is provided for those who prefer to read than watch the webinar. Please note that the transcript is raw. We apologize for any transcribing errors.
Speakers:
-
- Caitlin Croft: Customer Marketing Manager, InfluxData
- Nigel Griffiths: Advanced Technology Specialist, IBM Power Systems
- Ronald McCollam: Solutions Engineer, Grafana Labs
- Russ Savage: Director of Product Management, InfluxData
Caitlin Croft: 00:00:03.610 Welcome, everyone, once again. My name is Caitlin Croft. Welcome to today’s webinar. Super excited to have Nigel from IBM presenting on how he uses InfluxDB. Prior to Nigel’s talk, we actually are super excited to have Ronald from Grafana Labs and Russ from InfluxData to present on just a brief update from the two companies. Please feel free to post any questions in the Q&A box, and if you have any questions after the fact, please feel free to email me afterwards. I know questions come up after the fact. And without further ado, I am going to hand things off to Ronald.
Ronald McCollam: 00:00:50.834 Awesome. Thank you, Caitlin. And I’m Ronald McCollam. I am a solution engineer at Grafana Labs, so I spend a lot of time speaking with our customers and really understanding what people are using Grafana for out in the wild. And what I’d like to share with you today is a little bit of the work that we, at Grafana Labs, have been doing on Grafana 7 and a sneak preview of some of the things that will be coming soon in the Grafana 7 series. And I know Nigel’s the headliner here. I’m just the opening band, so I’ll keep things really brief, but I do want to hit some of the highlights here. If you could hit the next slide, please, Nigel. Thank you. So just to kind of recap Grafana’s philosophy. We are data source database neutral. Our goal in life as a product is to unite your data no matter where that lives, not make you fork that data off and lock down another copy somewhere else where you may not even have full control over it. And part of that philosophy is that we really want to be the best way that we can to visualize InfluxData particularly. So I’m pretty biased. I do work at Grafana Labs, but I think we do a good job of this. It’s something that we work very hard on, and we’re always trying to make that better. So I’ll share a little bit of that with you today.
Ronald McCollam: 00:02:07.830 Next slide, please. If anybody’s not already familiar with Grafana, just to recap what it is, it’s kind of the gold standard for open source visualization and dashboarding. It’s a way of connecting out to data sources wherever that data lives, whether that’s in Influx or Prometheus or other similar data sources, and then gives you the ability to apply really beautiful visualizations and dashboarding and alerting capabilities on top of that data. Next slide, please. So just a little bit about Grafana’s background. Grafana itself is, as I mentioned, an open source project, and it’s been around for about seven years now. Grafana Labs, as a company, was found slightly after the Grafana project, and we are really the kind of supporting organization behind Grafana. And we’ve definitely focused mostly on Grafana as a tool, but we’ve also worked to improve other types of open source observability and other tools in that space in general. And we always want to be able to work with open metric and logging tools wherever possible. And I’ll say my one and only commercial plug here is that Grafana Labs does provide an Enterprise version of their product, so if you need additional support or features that are aimed at managing very large-scale Grafana deployments, we’d be happy to help you with that. And I’ll not plug anymore from here.
Ronald McCollam: 00:03:30.742 So next slide, please. So what I really want to focus on today in the few minutes that I have is Grafana 7. So we recently launched this. This happened in May. And this is probably the most exciting release of Grafana ever. There’s just a wealth of new features in here, and I’ll hit some of the highlights of those. I definitely don’t have time to go through all of them, so I’d encourage you that if you haven’t played with Grafana 7 yet, go download it. Go grab a copy. Start kicking the tires. There’s a lot of really, really cool stuff in there. It’s a great release with a ton of updates. Next slide, please. I think the thing that’s probably most interesting to this audience is that we have full Flux support. So Grafana has had InfluxQL support for quite a long time, for a number of years, but as of Grafana 7.1, which is out now, you can actually use Flux queries directly in Grafana. So this will give you a really powerful tool to access and query your metric data from Influx and then visualize that in multiple different ways alongside of other data sources that you have in your environment.
Ronald McCollam: 00:04:37.041 Next slide, please. And I’ll hit on a couple of the other high-level features that we’ve got here beyond just improved Flux support. Broadly speaking, this release is about - Grafana 7 is about improving the underpinnings of Grafana itself. So a lot of these features are really interesting on their own, but they’re really mostly about building the framework for the next iterations of Grafana. So as an example, we’ve completely rebuilt the underlying data representation in Grafana itself. So this means that you can ingest a wider variety of data from way more sources than before. But since that data model is shared, you can combine all of that data together and then do predictive analytics or combined analytics on top of that data. And this also means, because we have this new data structure, we can bring in data from non-metric sources as well. So you can ingest things like log data and even trace data and visualize those inside of Grafana, no matter where that data is actually sitting.
Ronald McCollam: 00:05:40.079 So what that means is that you might have, say, logs sitting in Elastic and you can relate those to the metric data that you have sitting in Influx and bring those together in one dashboard to provide a full view of what’s going on in your environment and get those things to talk to each other in a meaningful way. We’re kind of extending this beyond just the data model into the user experience and user interface as well. So we’ve done things like normalized how visualizations can handle configuration and options and things like that and just unified those into a single view. So this will make it much faster for you to configure dashboards, to build out visualizations, and even build new plugins. And then finally, there’s a ton of work going on, on the plugin side itself. And we’ll talk about this in a moment, but this is going to dramatically improve how quickly you can build plugins and should improve the variety of both data sources and visualizations that are available in Grafana.
Ronald McCollam: 00:06:41.712 Next slide, please. So I’m just going to hit a couple of these from a very high level, and I’m going to start with that unified data model idea. So previously, every data source that was connected in Grafana had to build out its own way of managing data, connecting to the data source, manipulating that data, presenting it to the user, which really meant that you had a lot of inconsistency in the user experience. You had different sets of options for different types of visualizations, and plugin developers kind of had to reinvent the wheel to produce a lot of these things. But now that we’ve got this unified data model, that means that we know what data’s going to be represented as in Grafana, and we can kind of extract these things out and give you a common source for working with filters, thresholds, styling data, things like that so that they can be consistent across all the different visualization types. And if you’ll hit the next slide, please, I’ve just got a quick screenshot of what that looks like. If you’re used to Grafana 6 and before, you’re probably familiar with things jumping around and changing down at the bottom of the screen every time you select a different visualization. So it’s a little bit of a different experience now. You’ll see that everything has been moved to the right side of the screen, and when you select different visualizations, most of those options are actually going to remain consistent. So it’ll take a little bit of getting used to, but I think once you experience it, you’ll realize you can build out dashboards and modify existing dashboards much, much faster and easier than you could previously.
Ronald McCollam: 00:08:14.121 Next slide, please. So I mentioned that there are more changes than just the data model changes. So we’ve extended this out to the plugin system in Grafana itself as well. And if you’re not aware, basically, everything in Grafana is a plugin. So connecting to InfluxData, there’s an Influx plugin, or visualizing that data as a graph, there’s a graph plugin. So it’s sort of a plugin on either side. Previously, this was kind of ad hoc. Again, we didn’t have a unified data model for things to talk to one another. Everybody was sort of reinventing the wheel and building their own thing whenever they built a plugin. What we’ve done with Grafana 7 is start to extract some of the commonalities between plugins into reusable libraries. So what this means is if you want to do, say, a manipulation of data in your custom visualization, you can just inherit the same kind of data manipulation libraries that are being used in the default Grafana plugins. So this ought to make the level of effort required to produce both new data source plugins as well as new visualizations much, much easier. If you’ve ever looked at this and said, “Man, I really wish I had this kind of custom graph that combined line graphs and bar graphs or something like that, but it’s just too hard for me to write all the code to put that together,” I definitely encourage you to take a look at some of the new tutorials around Grafana 7 and building plugins. You should be able to build new visualizations much easier than before.
Ronald McCollam: 00:09:42.712 Next slide, please. And kind of the final bit around plugins and functionality here is the inclusion of tracing. So again, because we have this new data model, we can ingest things that are necessarily metrics, like logs and like traces, and visualize those in Grafana. So this is a huge change. This is something that is brand new for the 7X series, but it gives you a lot of power. So what you can do now is you can have a dashboard that has metric data coming in from Influx, and maybe you get a page for something high latency. Some issue is going on in your environment. You can follow the metrics from that InfluxData linked directly into logs right there in a Grafana dashboard, see what is actually causing that problem that got you alerted at 3 o’clock in the morning, and then even follow that log data straight into a trace that was captured when that problem occurred. So without ever leaving this one user interface, you’re accessing all sorts of different underlying tools and pulling that data back to get you to the root cause of your problem so that you can resolve it and go back to bed. In a previous life, I was an IT operations manager. I got plenty of pages at 3 o’clock in the morning, and I know that even a 10- or 15-minute improvement in how quickly I can find out what’s going on means I get to go to bed 10 or 15 minutes earlier, so this hits homes for me. This is a really cool feature.
Ronald McCollam: 00:11:09.931 Next slide, please. And so the final bit on the existing functionality I want to talk about in Grafana 7 is transformations, and this is probably the biggest feature, even bigger than tracing, probably the biggest change to Grafana as a whole since the project has begun. So again, previously, we didn’t have a standard data model. We didn’t have any consistent way of representing data when it was visualized in Grafana. So that meant that if you had, say, some data in Influx and some data over here in Graphite, you can visualize those side by side or even on the same panel, but you couldn’t relate them meaningfully to each other. They had different internal representations in memory of how the data was stored and handled. So they could really only be visualized together. Now that we’ve added this ability to standardize a data model, that gives us the freedom to take data, no matter the source, and apply transformations on top of that. So I can do things like filter data, reformat, change column names, change column orders. I can even do combinations of data. So I could do, effectively, a SQL join against InfluxData and Graphite data and derive a brand new metric from those two different data sources and visualize that just as a single line inside of Grafana. So in my time at Grafana Labs, this is probably the most requested feature that I’ve ever heard, people saying, “Why can’t I do analytics on this data? Why can’t I do cool combinations of data?” Now you can, and this is just such a cool powerful feature. I could talk for an hour about that. I won’t do that to Nigel or Russ because they have - yeah, Nigel’s shaking his head, so I won’t do that, but definitely, check it out. It’s a super, super cool and super powerful feature.
Ronald McCollam: 00:13:00.745 Next slide, please. And so I’m looking at the time. I only have a couple of minutes, so I’ll wrap up here quickly, but I do want to share now a few things beyond just what we’ve done in Grafana 7.0 and 7.1 and what we’re looking at through kind of the rest of the Grafana 7 series. And I think my formatting got a little broken. That should say Grafana H2 2020, if anybody’s wondering what that weird symbol is. So I’ve kind of harped on it a lot as I’ve talked about this underlying data model change and this underlying plugin model change. And the reason for that is that those things, while they’re cool and while they’ve added some very cool features to Grafana 7 already, what they’re really about is laying the framework for the next several generations of Grafana. So what we’ve seen so far is just scratching the surface of the power that these things are going to give. So we’ve got a lot of things planned to use these new features and use these new models, and I’ll hit a couple of the highlights here. So first of all, we want to use some of these to just improve the ease of use of Grafana as a platform. We’ve always been really good at kind of giving you a big box of Legos and saying, “You can build whatever you want out of this,” but you still have to take that first step and get the framework built out and get your data in and visualized in Grafana. So what we’re going to do now that we have this unified framework is start to be able to say, “Okay. Why don’t you just start with the castle model or the race car model? And then you can take that and then customize on top of it. So instead of having to go from 0 to 100 by yourself, maybe we’ll get you from 0 to 50, and you can get from 50 to 100 from there.”
Ronald McCollam: 00:14:40.539 We’re also doing a lot of work in surfacing more information from the underlying data sources themselves. So this is starting with Prometheus and Loki because these are projects that we, as Grafana Labs, are deeply involved in ourselves and contributors to, but it’s definitely not limited to those projects. I really hope to see Influx as part of this in the very, very near future. And what this will do is give you the ability to surface not just the actual data, not just, say, metrics from Influx or from Prometheus, but also metadata about things like how long that query took to run even down to things like query plans if those are available. So right there from a Grafana dashboard, you can troubleshoot errors in your data or why something is taking longer to render than you would expect it to do. So this should, again, just really improve the kind of feel and experience of using Grafana from a development and dashboard-building perspective.
Ronald McCollam: 00:15:39.655 I mentioned earlier we’re also bringing in some data sources that aren’t metric central, and this is including things like logs. And here, we’re starting again with Loki because that’s a project we’re deeply involved in, but we do have an open and democratic philosophy. This is also already extending to things like Elastic and Splunk, and we’ll see it go even farther out from there. What this is really doing is giving you the ability to not only visualize log metrics - or excuse me, log data in a dashboard, so seeing just raw logs right there next to your metrics, but start to derive metrics from the logs themselves, so being able to do things like counts of error messages in a log and render that count as a graph just as if it were a metric inside of Grafana. And of course, what that lets you then do is not only visualize it but alert on that data. So without having to necessarily create a custom metric collector to know when your 500 errors on your web server are going too high, maybe you can just pull that directly from the log data itself as if it were a metric data source
Ronald McCollam: 00:16:48.225 And that brings me to the last point on here which is around alerting itself directly. There’s a whole raft of improvements being worked on under the hood with alerting right now just the same as there were with the unified data model and the plugin model. Alerting is kind of the third tier, the third leg of what we’re focusing on improving in Grafana right now. So you’ll start to see some of this pretty soon, actually. You’ve started to see some of this in the 7X series already just in terms of more data sources supporting alerting directly natively in Grafana. But what you’re going to see is that we’ll be able to combine this and normalize the alerting capabilities across multiple different data sources, so you can do things like compound alerts from multiple different data sources. Maybe I’ve got some data, again, in Influx and some in Graphite, but I want to be able to alert on kind of derived metrics from the two of those. And we’re also going to give you more control over how those alerts are handled, how they’re forwarded, how they are muted, things like that. So really, this is just sort of the underpinnings of the next generation of the alerting system for Grafana. And next slide, please. I think that is all I had to say. I think I managed to get through it in about 10 minutes which is what I was asked. So thank you so much for your patience. And if anybody has any questions, please feel free to reach out to me on [email protected], or you can find me on Twitter as @RonaldMcCollam. Thanks, folks.
Russ Savage: 00:18:16.916 That was awesome. Super excited for all the new capabilities and enhancements coming in Grafana. And I’ve played around with it. It’s really amazing. I encourage everybody to jump in as well. My name is Russ Savage. I’m a product manager here at InfluxData, and I’m going to take a few minutes to talk to you a little bit about the InfluxData platform as a whole and then specifically about how we integrate with Grafana and some of the awesome work the Grafana team’s been doing to enable our powerful new Flux language in their platform. So if we jump to the next side. Really, at the core of InfluxData, we’re focused on providing a real-time visibility into stacks, apps, and systems. Right? And that’s a vision statement. It’s a broad message. But really, what that means is we want to enable developers and builders to create the next amazing application. And so if we jump to the next slide. Really, at the core of everything we do, we want to focus on those developers and builders. And so the tools and the products and the things that we create, we focus on developer happiness. We focus on time to awesome, which is our way of saying you’re able to hit the ground running and get started quickly, and really, starting from small, maybe a handful, hundreds of thousands of metrics, and scale that out into millions or tens of millions or even more.
Russ Savage: 00:19:49.878 So if we jump to the next slide. How do we do all this? What is actually involved in a platform? Really, we break this down into three big categories. First, we want to accumulate all of the information and all of the data around, and so we are insanely focused on time series data. It’s our belief that time series data is going to take over the world. And so we’re really focused on events, metrics, logs, tracing, basically any data with a timestamp. And I bet if you think about some of the data that you’re looking at, even if it doesn’t have a timestamp today, if you started collecting timestamps with that data, you’d be able to see interesting trends. And so our general philosophy is that almost all of your metrics are more interesting when you add a time component to them. Once you accumulate all of those individual data points, right, you need a way to analyze. Right? And so at the core of InfluxData is a powerful new language that I’ll talk about in a moment called Flux and really focused on easy to build and analyze your data. We really want to make sure that you, as a developer, do not hit the edges, do not hit the boundaries of what you can do with your data, and that’s really the core focus of Flux as a language. And then once you run that analysis, once you do that, you need to take action on it. Right? At the beginning, you might just be looking at trends. You might just be looking at dashboards and visualizations. But you want to create alerts. You want to create triggers. You want to create automation off of that analysis. Right? And so our InfluxData platform and our tools kind of encompass these three broad categories.
Russ Savage: 00:21:46.221 So I mentioned this a little bit in part of the analyze topic, but if we jump to the next slide. Really, when you think about a powerful data platform, it demands a powerful query language. Right? I’m sure a ton of people on the call have been using and loving InfluxQL, and we use it and love it as well, but it’s pretty easy to run into limitations. Right? It’s SQL-like. It’s not a fully capable SQL language. And so some of the amazing capabilities you have in more advanced SQL languages you just don’t have in InfluxQL. Right? And that’s okay because it sacrifices some of that advanced capability to make it super easy to get started and use. But what that means is, as an application developer, what you end up doing is pulling your data out of Influx and then actually writing custom logic and custom code to analyze and manipulate that data. And while that works, one, back to our original goal, developer time to awesome, the more code that you have to write, the less time you have to focus on some of the core business capabilities that you want to build out. And so our vision is with our data platform and our query language. We want you to take some of that logic that historically has been coupled with your application, move that closer to the database, move that into the platform so that it can run faster and be more powerful.
Russ Savage: 00:23:23.924 And so if we jump to the next slide. Did you skip a slide? Sorry. No, I guess you didn’t. That’s okay. So that’s really what we focus on as a platform. So what are the different pieces? Obviously, you guys are probably familiar with our open source InfluxDB: really key, everything you need to get started in a singular binary, really quick to deploy, really easy to use. We now have an InfluxDB Cloud which is everything you need, but we run it, and we manage it for you. We have a couple of different tiers there. One of them is a free-forever tier, so if you’re a hobbyist that wants to monitor and leverage our services for some of your personal projects - we love that - jump in there. And then obviously when you scale out and when you grow, there’s a paid offering. And then we also have the Enterprise as well which is if you want to run this stuff on your own hardware.
Russ Savage: 00:24:32.819 Really, at the core of - the big capability of this platform is, across all of these different products, we have a common API which means that applications that you build or you start building in the open source can easily scale out into Cloud or into Enterprise and without having to change your actual code and your application logic. So if you look across that common API, that enables really powerful integrations with data collection services. Right? So Telegraf, if you’re not familiar, a really awesome open source data collection agent. I like to say if data exists out there, Telegraf can capture it, so really, really powerful and really flexible data collection agent. We also have a full suite of client libraries and SDKs and, obviously, third-party integrations which is where this connection into Grafana comes in and really enables you to build those custom applications that you’re interested in.
Russ Savage: 00:25:31.319 So if we jump to the - if we jump to the next slide. So let’s talk a little bit more about how InfluxDB and Grafana 7 work together. So really, the awesome part about the new and improved InfluxDB data source and Grafana 7 is that it supports all of the InfluxDB versions. So if we jump to the next slide. When you’re releasing new capabilities or releasing new APIs, we really wanted to make sure that the experience of moving between versions of InfluxDB was as seamless as possible, and awesome engineers at Grafana made it happen. And so in Grafana 7, there’s a new and improved InfluxDB data source, and that data source can be configured to connect to any instance of InfluxDB wherever it’s running, so Cloud, OSS, Enterprise, 1.8, anything out there. And it can also leverage - as Ronald mentioned, you can leverage InfluxQL that you know and love, that you build all of your dashboards for, and you can also leverage Flux which, again, if you’ve ever hit the limits of the InfluxQL query language and what you can do, math across measurements or maybe some more advanced analysis, jump into Flux. Try it out. Take it for a spin. A really powerful language. So again, so you get the best of both worlds when you’re leveraging Grafana.
Russ Savage: 00:27:02.485 So if we jump to the next slide. InfluxQL is a SQL-like language. It’s familiar. It has limits. Flux, it’s a functional programming language. It’s a full programming language. Right? We always take about it as a query language, but it actually has the semantics and capabilities for running scripts outside of queries. So our vision with Flux is being able to write Flux applications that pull data from any data source, InfluxDB or anything else, analyze that data and write that to wherever you need it to write. And so it’s a really powerful language, and it’s really extensible. It has a libraries capability that means it’s really easy for use to build your custom functions and your custom analytics into the language. So you can import your own custom library for your company or your application and make it really easy to use and build cool stuff. And all of these different pieces put together - if we jump to the next slide - what that really means is you can move from wherever you’re running Influx now into InfluxDB Cloud or the next generation of Influx products with almost zero downtime. Right? Because the Grafana plugin data source is a drop-in replacement, that means that if you are writing your metrics to any of those versions of InfluxDB and you upgrade Grafana and you upgrade the data source, the same dashboards will run against the different InfluxDB platforms with the same capabilities and so really makes it easy to go in and spin up your new instances.
Russ Savage: 00:28:49.720 So if we jump to the next slide. Jumping into InfluxDB Cloud is super straightforward. So you sign up for a free account, as I said. It’s a free-forever account, no credit card or anything. Sign up. Configure your data sources in Grafana, again leveraging the new Grafana data source - or the new InfluxDB data source in 7.1. You can configure your original data sources. You can configure them to dual write if you want to test out the capabilities between different versions of Influx. That’s fine. We encourage that. You connect your Grafana to our InfluxDB Cloud, and you’re off to the races. So really seamless experience to move between different versions of Influx and keep the same visualizations and keep the same observability, that’s really kind of what we’re excited about with this new Grafana release.
Russ Savage: 00:29:42.499 So again, I won’t take up too much more of your time so we can jump into Nigel’s presentation, but what we’re really interested - as I said at the beginning of the presentation, we’re insanely focused on building an amazing platform and working with developers to build that, and we want your feedback. Right? And so we want you guys to come join us. Our community Slack channels are incredibly active. We’ve got a great community manager, Michael Hall, who’s in there, and the community is growing every day. So influxdata.com/slack, sign up, and jump in, and ask questions, and see what other people are doing, really awesome place. Obviously, on GitHub, our InfluxData data organization with all of our projects in there, I think - we’ve got a large number of projects. But jump in, and open issues. Give us feedback. Tell us what you like and don’t like. Obviously, I’m the product manager. I’m reading through those issues all the time, so look forward to seeing you there. As Caitlin mentioned at the beginning, we’ve got some community office hours that we run on a regular basis where we highlight new capabilities to community members and give you the opportunity to interact and ask questions. We have virtual meetups, virtual summits, and I think there’ll be a bigger plug at the end for InfluxDays, but a lot of different ways as we’re all kind of adjusting to the world that we live in. We really want to make sure that we’re getting the feedback from the community on the new tools that we’re putting out there. And that’s really all I had. So I thank you for taking time to listen. As I said, super excited about the new connection between Grafana 7 and InfluxDB. And now I’m going to hand it over to Nigel to talk about how he leverages Grafana and InfluxDB at IBM.
Nigel Griffiths: 00:31:37.758 Oh, right, my turn. Thank you for those two. I’ve learnt more, and I’d already listened to those practice sessions last week. So here we have me, Nigel Griffiths. I work in advanced technology support. That’s sort of new product introduction. And I got to say out loud that these are my personal opinions. I’m not an IBM spokesman. There’s no legal ramifications of any outrageous things I’m going to say here. And I was asked to talk about how IBM uses InfluxDB and Grafana, particularly in large production servers and benchmark centers. And I thought, “Whoa. We’re not going to go there - are we? - because IBM has 350,000 people. I’m not going to poll round and find out what they’re all up to and give you a summary of that.” So I know the people in some of the big benchmark centers that look after our mainframes and our Power computers, and they were one of the people I asked, when I was getting into these sorts of tools, what do they use. And instantaneously they said, “InfluxDB and Grafana. Don’t even bother looking anywhere else.” But I did do that. I also know that, because of my nmon tool and my njmon tool, people in services get a lot of customers that say, “Can you help us set up both of these products, the InfluxDB and Grafana?” And so I get questions from them, and then I encourage that IBM is inside their company to get up to speed and use them. Then of course, I actually talk to a lot of customers that take the tools. My tools are open source, and these are too, so very low hurdle to actually get started and give them a go. And once you see the value of them, then they actually tend to purchase the Enterprise versions or go Cloud.
Nigel Griffiths: 00:33:19.579 So very roughly, IBM, one-third software, one-third services, and one-third hardware. Yeah, I’m a bit blunt. They call it systems, and you think, “Well, what the heck’s a system? The planet is a system, so what do they actually mean by that?” Well, inside that, there are two different - well, three different groups, really. There’s the Power Systems which POWER9 chips, it’s the latest generation. They were our Unix machines. They run Linux, AIX, Unix, and a thing called IBM i which is like a cutdown mainframe sort of way of doing things. The operating system is enormous because it’s got a database inside it, but then you don’t have to purchase that. And these machines are quite big, so 192 CPU cores, lots of threads - we have 8 threads per core to run - 64 terabytes of memory, which is huge. You may not even have 64 terabytes of disk space. But we could suck in a 64-terabyte database into memory. If you do that, they tend to go about 10 times faster, so this is why people do it, and some applications just need a lot of memory anyway. Little bit of warning - maybe I shouldn’t say this, but I live in London in the UK. Houses are fairly expensive, but 64 terabytes of memory would cost more than all the houses in my street. I mean, it’s very big money, and the reason for that is the bitcoin people that buy up loads and loads of memory and keep the prices up. It’s nothing to do with IBM. We also have what’s now called Z or Z if you’re American. That’s the old mainframe that we’re famous for. Their latest chips are z15, and they run z/OS. They also have a variant of the same product that only runs Linux. It’s called LinuxONE. So they’re very big into Linux as a way of getting extra applications to run on all the hardware. And then there’s the storage division, but I’ll ignore them for now.
Nigel Griffiths: 00:35:18.566 Right. My claim to fame - and it’s 25 years ago; I’ve been at IBM 28 years, I think, and I have 10 years’ experience before that - is that I was in the benchmark center and I wrote this little tool called nmon, Nigel’s monitor. It put all the performance data onto the screen. And in those days, you had to go - this is a real history lesson here. We had dumb terminals, so we had 80 characters by 25 lines, and everything had to be squeezed onto that, so it was very condensed. And we also had a way that we could save the data into comma-separated files and had various tools to graph those tools. And for nmon for AIX, it was not open source. It’s a closed source. And it’s now part of AIX, and every time you install AIX, they install nmon. It’s really great. They actually have it running as well because it’s emergency fallback. If somebody says, “My machine’s going very slowly today,” they can look at the nmon data for yesterday and try and work out what the differences are to get an angle what went wrong. A couple of years after the AIX version, I created one for Linux. That is open source, and we got 690 downloads, so I reckon I got something right. And when you think a lot of IT departments might download one copy and then run it on 1,000 machines, I’ve no idea how many times it’s actually running at one time. That’s history. Right?
Nigel Griffiths: 00:36:37.248 Then I was thinking how things have changed since I was doing that 25 years ago, and it’s quite a shock how the computer industry has changed. So there’s the CPU 200,000 times faster. Well, the 200 is because we now have nearly 200 CPUs instead of the 1 that we started on 25 years ago. Memory’s a million times bigger. We’re now talking terabytes. Networks 10,000 times faster. I won’t tell you how fast we were going in those early days. It’s just so embarrassing. Your normal tablet will say the internet’s broken when it saw the data rate that we currently get in those days. Disks, they got a little bit faster, but they got much, much bigger. Didn’t they? And then that was a bit of a bottleneck. A lot of our big computers were running nearly 4,000 disks just to maintain the sort of I/O rates that we need for big databases, for example. But we’ve got coming in now the SSDs, solid-state drives, and NVMe attached so they’re not limited by SAS controllers. And so they’re quite a lot bigger, the SSDs, these days. We went to many terabytes and 10,000 times faster. We can get a million I/Os out of one of these, one little device that you can slip into your pocket where, in the good old days of brown spinning disks, 200 I/Os and you were flying, but it looks rather silly these days. The other thing is the nmon format. We had to do all sorts of ghastly things to the format because we used to crash, in the early days, Lotus 1-2-3. Half of you don’t even know what that means. Then it used to crash Excel as well. With the amount of data we could put into it, it’d just crash. Or it would slow down your machine’s [inaudible] paging, and it’d eventually just halt your PC, and you’d have to press the button to get it going again.
Nigel Griffiths: 00:38:23.463 So there are some of the opportunities. So I said to myself, “What would I do differently if I did it all again?” Well, instead of collecting a limited subset that I needed as a benchmark, I’d collect everything. There’s always some stats that you think, “I wish I’d collected that for the past year, and I could see how it’s been changing.” So that’s the sort of thing we want in there. A standard format rather than my quirky one. A central database. In those early days, we couldn’t send the data across the network because that would take 10% of the CPU running the network. These days, it’s noise level. You can’t even detect it’s happening. And then we want to do live graphing. With these comma-separated values, you had to wait to the end of the day or, at least, the end of the hour. Then you could draw some graphs and see what was happening. But we want live graphing. And so we sold all that. We’re collecting a lot more stats from our new tool. We use a standard format which is JSON. And LP is short for InfluxDB line protocol. And you can guess the other two tools that are fixing some major problems that we had with nmon.
Nigel Griffiths: 00:39:26.864 With the JSON format, we can push that into Elastic and Splunk, so it’s nice to cover the guys that are using the wrong databases. I think if I say that to this audience, maybe I can get away with that. And with the line protocol data, we can push that into Telegraf and get it to the Prometheus guys. Prometheus is a bit odd because it collects the data when it wants to rather than we push the data into the database when the data is ready. But Telegraf can join that up. An njmon user told me, “This is the config file for Telegraf,” and it was 10 lines long. I was shocked. First time I used Telegraf, I was just staggered about how clever that was in doing exactly what I wanted, and I thought it was going to take me months to try and work out how to solve that problem. So I actually have two tools. One’s called njmon because it’s JSON data, and the then imon because it’s generating directly to InfluxDB. If you go the JSON data, we send it off to a central daemon written in Python, and that uses the Python client library to push that into InfluxDB. And you can see the URL there to go have a look if you want to see some more.
Nigel Griffiths: 00:40:36.922 So let me talk for a few minutes about Grafana, and you have to say, “Wow.” Oh dear, if we could have this 30 years ago, it’d be - oh dear. But we don’t. You got it now. And I tend to think - every time I hear that thing, I look on Twitter and LinkedIn, they’re saying, “New version out. Go and get it immediately. Upgrade to it.” Then I look at the website. They have a page of samples of all the new graphs. And I go looking at all these new toys, these new graphs, and I’m thinking, “Ah, it’s really good. I’m going to have to give it a go, though.” So I thought I’d share some of my favorite things about the Grafana and the graphs. Number one is I could put my logo on the dashboard. I don’t think that’s documented. Maybe it is. Maybe it’s in there now. But I just found somebody else’s dashboard had a logo, and you open it up, and you can see what’s going on. You can learn from that. Number two, we got these nice pie charts, but we now have donut charts, and it just makes me smile when I think of a donut chart, especially when the wife is around. Anyway, number three, we have this dark background. And this is a little bit of humor in here. We have a lot of people as professional operators in the computer room sitting there all night watching what’s going on. And if they’re using the light background, the light is so bright off their big screens that they can’t get to sleep. So they switch it into dark mode, and then it’s all dark, and they can then snore all night with their nice dark backgrounds not waking them up, and then we can wake them up in the morning. There’s lots of jokes about the IQ level of operators. I’ll have to be careful there.
Nigel Griffiths: 00:42:10.910 Number four is this LED graphics equalizer. When I was a kid in university, we had lots of hi-fi systems with these LEDs that run up and down telling you whether your mega bass boost is kicking in and making the low-end noises louder. We’ve got a similar thing in here, but if you just look at that, your eye immediately goes to those two red quadrants up on the right-hand side. We can see one is the proc file system. Well, that’s not actually a file system. That’s a bunch of device drivers, so we discount that. But you can see, “Oh, my backup file system is nearly full,” and that’s something I really do want to know about very quickly. Number five is - I call these the button graphs. There’s probably a fancy name for single stats with background graphs or something. The nice thing about these is, on my screen, they’re about an inch wide, and I can put - I don’t know - 10 by 5, the 50 numbers and graphs on one screen. So I can get a lot of data, and we can get the backgrounds to change color if you go over an alert level, and it’ll draw your eye to it immediately. So you have one glance. You see everything here red or orange. “No. Okay. Let’s look at the next machine.” And I can get through a lot of data very quickly. Number six is this chart in here. They got a couple of examples. I just think of the Blue Ridge Mountains - is it Virginia or something? - in America. I’ve been there. I’ve walked those mountains. But this lovely, sort of peaceful-looking graph just sort of makes you feel all sort of romantic, and I don’t usually feel that way about my computers. And the last one is the carpet graph, and we’ll look at that in a minute.
Nigel Griffiths: 00:43:49.882 So I presume you’ve all heard of the Dolly Parton curve. When we gave the practice run, the three other speakers never heard of this. Half of them didn’t even know who she was, so you have to look that up. This may give you a little clue. So this is a famous graph when you’re talking about big, big production servers. So we’ve got the morning, then the evening on the right-hand side. And then people come in between 8 and 9 o’clock, and the workload slowly builds up. Then we have a mid-morning peak. Then people start sliding off for lunch over sort of a time period, over two hours. Maybe they take their hour lunch break, so there’s the dip in the middle of the day. And then there’s another peak in the afternoon when people are trying to finish things off so they can go home, and then they slide off between 5:00 and 6:00 or 7:00, whenever they do that. Then the machines typically aren’t very busy at all. But then some early hour of the morning, they run the batch run. Look, it’s written properly. Then the machine gets 100% busy. Sometimes the problem is they can only make it go single threaded, and that’s another story. But they try and run the batch run as fast as they possibly can, and what’s critical there is how long the batch run takes.
Nigel Griffiths: 00:44:58.660 So we’ll go onto the next one. There’s sort of three crunch points in here. How high is the morning peak, the afternoon peak, and how long did the batch run take? They’re the three numbers that we’re really interested in. Now, the problem is if you take the average over the day, it’s going to say, “50%. There’s no problem here on this computer at all.” But one of these crunch points could be about to hit you next week or the week after, and you really want some sort of heads up about that. So not only is this a daily thing going on here, there’s a weekly trend as well. People tend to sell more or do more computing on Friday than they do on Monday. Maybe they’re just trying to get all the work done so they don’t have to work the weekend or something like that. Then there’s this period over a Monday. There’s end-of-month reporting and then maybe end of year. That could be Christmas problems or financial problems or whatever. So we got periods over periods over periods, and so we have to watch those, and we want to watch those batch run times as well.
Nigel Griffiths: 00:46:00.495 So this is an example of the carpet plugin, and this is fantastic in here. And it’s a heat diagram. Along the bottom, I’ve got the different days of the week for the past three, three and a half weeks. Then up and down the sides is from midnight till midnight the next day. So you can see very quickly. Your eye says, “Whoa. There’s a hotspot in here that’s in Thursday and Friday. That’s when this computer is particularly busy. That’s the one we have to monitor in those particular hours.” I put a little blue star in the middle of this chart in here because I’m a technical person. I don’t tell fibs. I’m not a salesperson. And so I got to Monday midday, and I was just looking at all the previous parts of the graph, and it’s just green on green on green. It’s really boring. That’s not like my production servers. So I put in a bunch of workloads, and you can see later that day and then the rest of the week I was generating workloads to generate a pattern that you might more typically see in a customer environment. So we want to monitor the peaks in, well, 8:00 till 10:00 and 2:00 PM in the afternoon, Tuesdays to Fridays. The busiest day is a Thursday in my example in here. Well, I hope you’re getting the idea even if I faked up a little bit of the data.
Nigel Griffiths: 00:47:33.659 So my things to do are to actually work on these sorts of graphs and see if we can get some trends coming out of this. And this batch overran. We can handle that with maybe some alerts, but we still could do with some trending analysis. And when we started, we started at 200. We’ve maybe got many more people logged in now. All our collective brainpower, if you could all work on this for the next half an hour, I’m sure we can get it solved, either on the Flux side or on the Grafana side, maybe both. So one way of working out, “What do you want to see -“ or what you need is, “What do you want to see?” So we’ve got three ideas here. At the top, we’ve got what I call removing the weeds. Remove all the data when it’s not busy, and then you can see the interesting bits. And funnily enough, machine learning or analytics can be very hard to do all the maths. I find it hard anyway. Your eye looks at that and says, “Whoa. Next Friday, we’re going to hit the top of this peak, and we got a real problem on the computer.” And so your eyeball and your brain can actually spot trends a lot, lot easier than some of the mathematics can. These days, I know there’s a lot of packages in libraries that allow you to do that. Another way is sort of just cutting out those two hours and then presenting them in different colors, perhaps, getting darker as it gets up to the more recent data. Or you could do the accumulated busy minutes for that hour, then draw those in a graph,
and again, your eye will tell you that you got a crunch point coming up in a week’s time. So I wanted to find out some ways of doing that automatically.
Nigel Griffiths: 00:49:10.908 Now, to finish off, I’ve got two little projects that I’ve been working on recently. It’s not easy to get the documentation for the measures and statistic names. Now, my two tools actually generate quite a lot of stats, and people are saying, “I can’t see the wood for the trees. I’m pretty sure you’re collecting this stat, but I can’t find it. How do you spell it, or which measure is it actually in?” And I’ve been trying to find out the similar thing sort of with Linux statd, trying to eye up the competition. How many stats do they gather compared to me? And also, for these capturing ad hoc stats on big servers, you want to add some extra ones to the njmon data. How would I actually do that? Well, I’ve got articles on my blog that was on my first sheet. I’ve just written a shell script. It’s not rocket science. It takes the Informix - sorry, the InfluxDB line protocol data and just uses it as a text file. So it outputs the tags. So you can see those there. The hostname is blue. It’s AIX. It could, coming up in here, be Red Hat, SUSE, or Ubuntu. So I tend to have different graphs for AIX and the Linuxes because the data’s slightly different. Then we can look, “What sort of process have we got in this serial number?” It lets you grab all the virtual machines that are on one particular machine. We can move them about dynamically, live, so they’re coming and going. So you want to track those.
Nigel Griffiths: 00:50:41.441 Then we got other things in here like the configuration information. A lot of this you’re not going to graph, of course. Then you got the CPUs, the disks, the memory. And on our Power machines, we have this thing called a rPerf, a relative performance measure. That’s very useful if you want to say, “Okay. I take all these logical partitions and put them onto a new machine. How big does it have to be to actually do that?” At the top corner in here, for AIX, we’ve got 1,400 or so metrics. And for Linux, we’ve got 800 metrics to actually look at and learn from. But now we got this very simple tool for documenting what we got in here. I strongly suspect that the Influx and Grafana guys are going to say, “Oh, yes, if you hit this button over here, this would already be generated.” But it’s useful to do. There’s a famous chart, isn’t there, about, “You want what?” and people laughing, and it’s programmers writing documentation. It’s like, “Whoa, no.”
Nigel Griffiths: 00:51:39.977 The other one is if you want to add data. Now, in big production servers, they can’t add tools. If you said, “Well, I want to get the data in Python,” oh, no, you cannot install Python on AIX in a production server. It would have to go into the test cell for six months. There’d be testing to approve that adding these packages won’t have an effect on the production workloads, and then it’ll be rolled out to those production machines over the next two or three years. But if you say you got one little command, you can stick it in a usr/local/bin, and if it goes wrong, you can just delete the one thing, then they’re usually happy to do that. And it’s part of their sort of toolbox of scripts that they run as systems administrators. So I’ve got a tool in here - it’s now called Measure - where you give it the measurement in Influx terms and then the statistics. This is in line protocol format. And it grabs the tags for you, puts those in, and sends it off to the InfluxDB. So this is something that a lot of customers want to add their own data. And I’ve got some examples there of databases, sales, what the users are up to, or some of the things that the IT guys are doing.
Nigel Griffiths: 00:52:51.373 Okay. Once you have a time series database, you suddenly realize, as was already said, there’s a lot of other things going on in the computer room which are just data that you could graph and learn a lot from. I’ve got friends that run the networks, and their prime interest in your network router is how hot it is. What? Because they know if anything goes wrong, it’ll either keep the adapters that are running the network go hot or the CPU goes hot or even the memory goes hot in there. And so that’s the first indication that something’s wrong. Maybe they got a packet storm going on that the temperature will rise as it tries to deal with that before any of the other measures will actually turn up. Here’s my little example. It’s a little pet project. I got a Raspberry Pi with a little SD card in it and five temperature probes across here. The dark green is going down the vent where the air conditioning comes out. Then the yellow one above is how hot the computer room is, and then I got three in the back in my servers. And you can see the temperature’s going up and down. I learnt the hard way earlier this year, the Raspberry Pi can do so much I/O to your micro SD card that they wear out. I asked my friends in IBM. They said, “Oh, yeah. We know about that.” And they tend to try to use SSDs instead to get around that.
Nigel Griffiths: 00:54:13.267 So my Raspberry Pi was returning zeroes for everything, so I eventually got into the computer room. You can actually see how long it took me to actually get permission from the managers to let me in the building because of COVID problems. So it took three days to get permission. I restarted it, and it worked again. I thought, “Oh, fixed.” And then a couple of days later, you can see in here it fell off the network. It was responding nothing, and Grafana just joins the dots to cover the gap sort of thing in here. I had to go in. I let it all cool down, the SD card. Then I quickly copied it onto another SD card, and I actually got the data off it, and off we go again. And you can see this particular Friday is at the start of our heatwave here in London, and the computer room goes up because we’re trying to get rid of the temperature on the roof and the heat exchanges. And we can see we’re in another heatwave coming in here, and it’s getting too hot. Now, I think that’s it from me. If you’ve got any ideas that can help me with my particular project, do do that. I’m a big fan of Influx and Grafana. I’ve got 12 movies on my project, and that includes how to install them and get them working on the Power machines and using my data. No, wrong button. Back to Caitlin.
Caitlin Croft: 00:55:35.892 Perfect. Thank you. That was great, all three of you. Just another friendly reminder and shameless plug for InfluxDays. So InfluxDays is happening in November. The event is completely free, so I hope to see all of you there. About a week and a half prior to InfluxDays, part of the conference, we have our hands-on Flux training. So if you’re wanting to learn more about Flux, be sure to sign up. There is a fee attached to the Flux training, but that’s just mostly because we want to make sure that we have a really good student-to-instructor ratio. So it’s really fun. The instructors are data scientists based in Italy. They’re really fun guys, and because they’re Italian, the examples that they use throughout the training is all about pizza-making in an IoT sensor-enabled pizza oven. So it’s fun, and then you want pizza afterwards. So hope to see everyone at InfluxDays. It’ll be really fun. So we have a few questions here. So the first question is - someone’s just interested in knowing a little bit more about the differences between Grafana and Chronograf, especially when you’re connecting it to InfluxDB. So they’re interested in the different capabilities of the two products.
Russ Savage: 00:57:04.730 Yeah. I can touch a little bit on that, and then, obviously, Ronald can take it as well, but. So Chronograf specifically was built as the UI interface into the InfluxDB platform, so it’s really focused on only communicating and talking to InfluxDB. And in addition to some graphing capabilities, it also has some InfluxDB management capabilities and also the ability to edit TICKscripts in Kapacitor and things like that. And so it’s really hyperfocused on a very specific set of technologies as opposed to what - I’ll let Ronald describe the vision for Grafana.
Ronald McCollam: 00:57:47.736 Yeah. I think we’re on the same page. I think Grafana’s goal in life is not to be a better Chronograf than Chronograf. We’re never going to be able to do that. What Grafana wants to do is give you a common set of capabilities across multiple and different data sources. So if you have only InfluxData, Chronograf’s awesome. I like to think Grafana’s awesome there too, but there’s probably not a huge amount of differentiation. Where Grafana is going to be more useful is when you have, say, InfluxData and you want to combine that with Elastic data, and you want to be able to unify those together in a similar or in a consistent set of graphs and bring them together into one dashboard. So it’s not about individual features with Grafana and Influx, specifically. It’s more about connecting to multiple sources of data.
Caitlin Croft: 00:58:40.742 Perfect. Do you have any update on InfluxDB 2.0 release timeline? I think that’s probably a question for Russ.
Russ Savage: 00:58:49.439 Yeah. Yeah. Awesome. So you might have noticed yesterday we actually published in our community forums - our developer advocate, Michael Hall - or our community manager, Michael Hall put together an awesome document to describe the roadmap to release to get InfluxDB open source data out of Beta. And so we’re planning to do that in the next couple of months, and so we look forward to an early Q4 release for that. But for more details, check out the community post from Michael Hall.
Caitlin Croft: 00:59:26.771 And he did post it in Slack as well. So if you can’t find it, feel free to email me, and I can shoot it over to you. I think this next question is for Nigel. How many metanodes are in your cluster, and how do you deploy them in multiple data centers across regions? For example, how do you cope with network latency where metanodes have zero tolerance for communication between nodes?
Nigel Griffiths: 00:59:56.378 [laughter] Right. Basically, that’s not my problem. My problem is getting the data into InfluxDB. And how you do that is very well understood by the Influx database team because they run a massive Cloud infrastructure and they know how to do that. So over to Russ.
Russ Savage: 01:00:15.470 Yeah. So I guess when we get those type of detailed questions on webinar, it’s probably better to follow up on an email outside of this. But long story short, shouldn’t be a huge problem, and we can send documentation and explanation on why.
Caitlin Croft: 01:00:33.365 Okay. Perfect. I will follow up with the person and cc you, Russ, on it. The next question is asking if this session is recorded. Yes, it is being recorded and will be available for replay later tonight. What are the advantages of a dedicated time series database versus a plain old relational database?
Russ Savage: 01:00:55.564 Sure. So I’ll take that one. So there’s nothing saying that you can’t put time series data into a relational database system. I think the key advantages of using a dedicated time series database is, out of the box, it comes with a ton of capabilities and features that are focused around analyzing time series data that you would normally have to build yourself and manage yourself. If you’re taking a general relational database and turning it into a time series database, you have to write a lot of extra software to make working with that data much easier. A time series database has all of those capabilities built in. The other thing is when you’re dealing with time series data, it has a particular shape that’s different than a relational data shape, and the data comes in in much higher frequency, much higher volume than you might be used to in a relational database. And because we know that it’s time series data, we can make a lot of assumptions and really tune the performance for that high ingestion rate and that high speed. So again, nothing stopping you from putting time series data into a relational database system, but using the right tool for the job gives you faster development and better performance.
Nigel Griffiths: 01:02:26.799 I’ve got quite a lot of experience with relational databases. Oracle are my big servers, for example. And I like the flexibility. So if I want to add a new measure - we’ve used GPFS, for example; it’s a distributed file system - I can just throw that in along with 28 different counters, and Influx still says, “Yeah, sure. We’ll manage that.” If you’re trying to do into an SQL database, you have to define tables and indexes and all sorts of other things before you get them in. And if, tomorrow, I say, “Oh, actually, there’s a 29th stat, and I’ve wrecked my SQL database. I have to start managing the tables and adding extra columns,” it’s a real nightmare. So the flexibility of a times series database like InfluxDB is enormous advantage to just keep you going and doing what you really want to rather than spending time managing an SQL database.
Ronald McCollam: 01:03:17.737 And at the risk of piling on, sorry, I’d just say that - I’d echo what Russ says about performance. I know personally, in the past, I’ve done things in my more naive days of like, “Oh, I’ll just throw a bunch of sensor readings into my SQL, and I’ll capture those readings every 10 seconds.” And that’s great until I decide to go back and look at 30 days’ worth of data and I realize I didn’t index any of that. I didn’t build out any of the things that are necessary to give the database the ability to pull that data back in a reasonable amount of time, and now my queries are timing out. So those things are just taken care of for you by a time series database.
Caitlin Croft: 01:03:55.181 Did you turn on the anti-entropy service in production to monitor the IBM servers? Were there any data inconsistencies you experienced so far in the cluster? And if so, how did you resolve it?
Nigel Griffiths: 01:04:14.502 [laughter] I’ve no idea what you’re talking about.
Russ Savage: 01:04:16.978 I’ll take that one. So -
Nigel Griffiths: 01:04:18.964 Thank you.
Russ Savage: 01:04:19.805 So anti-entropy is a service that helps sync shards across clustered InfluxDB. And so if you’re having questions or concerns about the anti-entropy service, please connect with us and reach out, and we’ll figure out to help you. But yeah, it’s a capability of clustered InfluxDB.
Caitlin Croft: 01:04:39.581 So yeah. They had another follow-up question here. How big is your cluster, how many data nodes, and what is the replication factor? Are these data nodes all running on physical servers or VMs? So I think that should -
Nigel Griffiths: 01:04:54.774 Not my problem. I would say that we’re talking about big servers here. So I can throw a machine with 200 CPUs and 64 terabytes of memory at it if I’ve got problems with scaling, where if you’re running it on your more usual AMD64 machines, then you have to start scaling at a lower level. But I’ve got some, well, well-known worldwide banks that are using my tools, but I’m supplying the data gathering tools. Initially, when they’re smaller scale, they just bung it all into one database, and then when they scale up, then they want to talk about the Cloud offering so that’s all done for them if they don’t want to get involved in the details of them again, perhaps the services from InfluxDB to setting up bigger clusters. So I haven’t been involved at that level.
Russ Savage: 01:05:44.252 Yeah. And check out our - so in our documentation, we’ve got some hardware sizing guides for Enterprise. But yeah, in general, Influx will use everything that you give it. And so if you need to analyze more data, you need to run larger queries, throw more resources at it, and Influx will happily leverage them. And obviously, in a clustered Influx, the nodes that are running the actual data stores and actually doing the processing of the data nodes, so those tend to be much beefier and heavier servers. The meta nodes are relatively light. They’re acting as routers for requests back and forth and some metadata, so. But yeah, check out our docs for more details or connect with us.
Caitlin Croft: 01:06:37.854 Perfect. How would you compare Apache Spark plus InfluxDB plus Grafana combination for data analytics?
Russ Savage: 01:06:49.617 Yeah. I can see that in the chat. So Apache Spark, I used to work at a small Hadoop company. Apache Spark, a really awesome processing framework for Hadoop, and I think you can probably use it outside of Hadoop these days, but really built around custom application as much as I understand it. The combination of InfluxDB and Grafana is meant to be a really easy way, almost a no-code - basically, a no-code solution for setting up data processing and analytics for your metrics, which is slightly different than Apache Spark, more of a build-scripts-and-coding solution. Does anybody else have any different understanding?
Caitlin Croft: 01:07:44.483 There seems to be consensus across the panelists, so.
Russ Savage: 01:07:48.984 Yeah. I mean, they’re different technologies for different tools, and using one does not exclude you from using the other. And so if you have the data that you need the power of Hadoop and large-scale processing, you can then leverage Grafana or InfluxDB after those jobs run to visualize the results. And you can leverage our technology to monitor the JMX Java services that those things are running on, so.
Caitlin Croft: 01:08:21.454 Perfect. There was another question asking if the webinar would be available for offline viewing. It will be uploaded for viewing, but you’ll need internet access. But it will be available for replay and the slides will be available to review later today. We’ll just keep it open a little bit longer, just another minute, see if anyone has any last-minute questions for our awesome speakers. It was really fun hearing -
Nigel Griffiths: 01:08:48.847 Some of those [crosstalk] mentioned may make an excellent other session for somebody else to answer, some hints and tips when you go to the next stage when you run out of power on one box. I’ll certainly attend.
Caitlin Croft: 01:09:01.741 [laughter] I love it. Well, thank you, everyone, for joining today’s webinar. Appreciate you guys staying on. I know we went over a little bit. Once again, this will be available for replay later today. Thank you to our panelists for presenting. And if you have any more follow-up questions, you all should have my email address. You’ll also get an automated email from Zoom after the fact with my email address, and I’m happy to connect you with our speakers. So happy to connect you with our speakers, and thank you very much, everyone, for joining today’s webinar.
Russ Savage: 01:09:47.273 Thanks, everybody. Have a great day.
Ronald McCollam: 01:09:48.405 Thank you.
Nigel Griffiths: 01:09:48.982 Bye, all.
[/et_pb_toggle]

Nigel Griffiths
Advanced Technology Specialist, IBM Power Systems
Nigel Griffiths has 40 years experience in the computer industry in many roles. Starting as a program in C, porting the UNIX Kernel to new hardware, UNIX system admin, RDBMS DBA, Admin and performance tuning, Benchmarker, new server testing, product launch, IBM Technical conference speaker and evangelist for Power Systems and AIX. 7000 followers between Twitter and LinkedIn and 200 technical topic YouTube videos. When relaxing Nigel codes and support open source performance monitoring tools for AIX and Linux. Warning: don't ask him about njmon, unless you have 4 hours to spare!
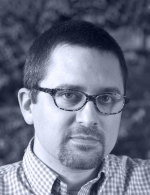
Ronald McCollam
Solutions Engineer, Grafana Labs
Ronald McCollam is a "geek of all trades" with experience ranging from full stack development to IT operations to management. He has a strong background in open source software, starting when a stack of 3.5" Slackware floppies was the *easy* way to install Linux. He has architected and managed everything from "Big Data" systems at Canonical and Hortonworks down to embedded IoT devices at balena.io, but the common theme with all systems has always been monitoring. So Grafana Labs is a perfect fit! When not on the road, Ronald resides on his back porch in Somerville, MA with a frosty beverage in hand.
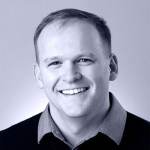
Russ Savage
Director of Product Management, InfluxData
Russ Savage is the Director, Product Management at InfluxData where he focuses on enabling DevOps for teams using InfluxDB and the TICK Stack. He has a background in computer engineering and has been focused on various aspects of enterprise data for the past 10 years. Russ has previously worked at Cask Data, Elastic, Box, and Amazon. When Russ is not working at InfluxData, he can be seen speeding down the slopes on a pair of skis.