How the Aquicore Solution Cuts Energy Waste and Improves Tenant Comfort Levels with InfluxDB Cloud
Session date: Apr 30, 2019 08:00am (Pacific Time)
Aquicore is revolutionizing “Asset Operations” for Commercial Real Estate owners & operators. In over 700 buildings and 150M SF, Aquicore is the backbone to IoT data enabled real estate decisions, paving the way for an autonomous building future. In this webinar, you will hear from Aquicore’s VP of Product, Mike Donovan, on how they use InfluxDB Cloud to collect and store the metric and event data from utility meters, submeters, building equipment, and other environmental conditions of the buildings to help their SaaS solution deliver real-time and actionable insights.
Watch the Webinar
Watch the webinar “How the Aquicore Solution Cuts Energy Waste and Improves Tenant Comfort Levels with InfluxDB Cloud” by filling out the form and clicking on the download button on the right. This will open the recording.
[et_pb_toggle _builder_version=”3.17.6” title=”Transcript” title_font_size=”26” border_width_all=”0px” border_width_bottom=”1px” module_class=”transcript-toggle” closed_toggle_background_color=”rgba(255,255,255,0)”]
Here is an unedited transcript of the webinar “How the Aquicore Solution Cuts Energy Waste and Improves Tenant Comfort Levels with InfluxDB Cloud”. This is provided for those who prefer to read than watch the webinar. Please note that the transcript is raw. We apologize for any transcribing errors.
Speakers:
- Chris Churilo: Director Product Marketing, InfluxData
- Mike Donovan: VP of Products, Aquicore
Chris Churilo: 00:00:01.227 All right. So with that, it’s three minutes after the hour and I’d like to get started at this point. And as a reminder to everybody, the session is being recorded so if you want to take another listen to it you can. You’re going to put your questions in the chat or the Q&A panel. You can find the icons at the bottom of the Zoom application. And, yeah, let’s sit back and relax and let’s listen to Mike from Aquicore tell us about how he was introduced to InfluxDB and what they use it for. So I’ll hand it over to you.
Mike Donovan: 00:00:35.038 Thanks, Chris. Hi, this is Mike from Aquicore. I’m the head of product at Aquicore. I’ve been here for about three and a half years. I started as really the first full stack engineer at Aquicore and just kind of took on more and more responsibility. And throughout that time went through a migration from Postgres as our time-series database to Influx as our time-series database. And, personally, I’ve been following Influx for a while and used it at previous jobs. But first, I just want to say thanks to Chris and Influx for giving me this opportunity to tell you guys about Aquicore and how we use Influx. Let’s see if I can - there it is.
Mike Donovan: 00:01:18.525 All right. So I want to go over today a little bit about Aquicore and what we do, the business that we have, and the platform that we have. I’ll go over our data collection architecture from inside of a building and then all the way to the cloud and then how we store that data. And then I have a list of challenges that we’ve come across throughout the three years of using Influx and how we’ve overcome most of them. And there’s a few that we’re still working on. And then, any questions, definitely here to answer.
Mike Donovan: 00:01:52.173 So I’ll start with an overview of Aquicore for those who are unfamiliar. We’re a platform for commercial real estate. For those of you who maybe don’t understand the commercial real estate world, this is really talking about office space, right? I’m sure the people who manage and own the real estate that you work in right now at your job, they actually have a lot of issues that need to be solved for. A lot of them have to do with outdated technology and inaccessible data, right? Most people are actually only able to receive one point of data about their energy usage, water usage, which is their utility bill, and that’s never enough data in order to make any smart decisions based off of it, right? And so what they end up doing is using the human tool to walk around the building and take readings that are erroneous and they just cost a lot of time. They have systems that are called building management systems, right? These are like fairly robust, controlled systems and sensors throughout the building but they’re very dated. They’re super old school. It’s essentially just a server that’s on-premise that has no historical capabilities and you really can’t do much with it and you always have to pay a contractor to come and do something. The equipment on these buildings - I mean, these buildings are decades old. So the equipment is really bad and outdated. And, again, they need more data in order to understand how their equipment’s being used.
Mike Donovan: 00:03:30.433 And then the fourth one, which is misaligned tenant expectations, it really impacts the bottom line of real estate because that’s how they obviously make money is by leasing out their spaces. And so I like to think about it like the real estate industry is kind of 5 or 10 years behind the curve of technology and that’s why kind of Aquicore exists. So there’s these three main people that we’re thinking about, these different personas, that are impacted by this lack of technology and data access, property managers, building engineers, and accounting. So the property managers are the ones who have to deal with the tenants and leasing the space, right? They’re the ones who probably talk to your office manager to get the office space that you’re in leased out. And then the building engineer is the one on site who’s actually spending most of his day responding to complaints, handling equipment, walking around the building making sure that everything is operating as it can be. Safety is a big deal for them as well as energy, right? All these people really do care about the energy usage of the building as well as how much it is costing them because imagine, everyone’s paying a lot for electricity.
Mike Donovan: 00:04:48.636 And I think the worst part about this setup in commercial real estate is just that they’re very siloed data sets, right? So the property manager has one reason to collect energy data while the building engineer has another reason and they’re completely siloed. And there’s no central platform that can give access to all this data. So what we do at a high level is we centralize the operations of real estate for these different personas, right? And we look at a few - we provide products in a few different areas of work for these people in real estate. The first is financial performance, right? So this is your utility budgeting, your tenant billing capabilities, billing back your tenants for their energy usage, and really just understanding how your utility spend is impacting the financial performance of your building. A big one that is super easy to understand is the utility and facility performance, right? So this is really understanding the energy consumption in the building, the water consumption, gas consumption, understanding the equipment usage. And this is where really that monthly utility bill just doesn’t provide enough information.
Mike Donovan: 00:06:08.162 And then people performance is last, right? So this is really thinking about the team of people in real estate that need to use this data to do their job, right? Again, whether it’s the property manager running the tenant billing cycle every month or running a yearly budgeting process, right, really thinking about that workflow that connects to the people in real estate. And it’s all based off of getting this real-time data and then exposing it and making it usable for these people. The main features that we have are bucketed into these four categories. So performance optimization is where we’re using this real-time data, equipment data, energy data, to find opportunities to improve the operations of a building. So this is where real-time monitoring of data comes in and you can actually see what’s going on in your building and be able to make changes, whether that’s changing your start time, when the building comes online in the morning to verifying that the equipment is running as you would expect or to make sure that the building doesn’t operate on a weekend. You have no idea how many times that actually happens.
Mike Donovan: 00:07:22.402 Utility budgets are another area that I kind of mentioned, right? So just centralizing the bills, the utility data, and all this to be able to track and predict what your spending will look like. Tenant billing is all about taking the real-time data and making it so that you can actually bill your tenants for that energy usage. And then, last but not least, which is kind of the reason why Influx is so important, is our building connectivity, right? So a lot of - we’re an Asset Operations platform but we’re also an IoT company. We will go in and install our devices, our edge devices that will web-enable the building and the meters and then send that minute-by-minute data to our cloud which is then ingested into Influx. And we can do that for - I’ve talked a lot about energy. But we’re also doing that for any other kind of sensor in a building, a humidity sensor, a temperature sensor. My mandate is to always go and find new sources of data to ingest.
Mike Donovan: 00:08:31.675 So here’s a look at some of what the platform does, right, just at a high level. We have a mobile app where you’re able to get access to all this real-time data on the go. So that’s just showing you your energy consumption with some predictive energy in there as well. So there’s predictive analytic capabilities and there’s a whole host of other things. We also have a web app that has all the dashboarding, VI, and just sort of broad data and workflow tools all housed within it. We’ve also got a fairly robust marketplace and integration platform where we partner with a lot of other companies in the commercial real estate landscape. And we like to consider ourselves, definitely, an open platform that makes our data accessible and likes to integrate with other platforms which is fairly a new concept in the commercial real estate industry. And then, finally, the IoT connectivity, right? So we actually build and deploy our own networking hardware. So the one difference is we’re not necessarily building the utility meter, right? We’re going to hook up one of these devices which is called a Hub, an Aquicore Hub, connected to one of the utility meters which then sends data to our cloud, right? These are normally what we call dumb meters that we will turn into smart meters.
Mike Donovan: 00:09:56.664 After you connect and you get all this data in the platform - just finish up with some of these other features, right? We have portfolio recording that we have to make all this data accessible to. Everything you see here is all real-time data that we’re ingesting and running calculations on through our Influx integration. So whether it’s understanding the energy usage, the actual dollars being spent, or the water usage, gas usage, all that stuff. We set budgets and targets for people. So this is, again, kind of our ability to make this data very usable for the people of real estate, right? Most people can know what their energy usage is but they don’t know how to make a behavior change happen with that data. So by setting these performance targets companies are able to reduce their energy usage and their utility spend. It’s kind of like a fitbit-style approach here as well as giving you real-time insights into your utility spend and where your money’s going between you and your utility bill.
Mike Donovan: 00:11:02.096 I mentioned tenant billing before, another aspect where real-time data is essentially, I mean, honestly, revolutionizing the industry here where we’re able to remove a completely manual process that exists in a whole host of buildings. And you just come in and you click a few buttons and with real-time data you can now bill your tenants for energy usage. Then there’s kind of our bread and butter which is just visualizing the energy use, the real-time data coming into the platform throughout your entire building broken down in multiple ways, right, whether it’s for the whole building, whether it’s for an individual piece of equipment, whether it’s for an individual piece of meter, all of that is accessible. And through an API you can export the data as well. The way that it all kind of works is that we have the ability in the platform to manage our fleet of devices that exist and those hubs that we showed. And so we have a devices and equipment module which essentially not only lets you manage our devices, our networking equipment that we’ll install in a site, but you can also inventory your entire building with the mobile app so that you know - which is actually not something that usually exists in a digital version, right? How many meters do you have, how many pieces of equipment do you have, is all something that you can collect in the Aquicore platform. And then just some more mobile here, getting access to weather predictive capabilities and then being able to track issues that are happening in your building in real-time. And then, finally, dashboards. Every tool that’s a real-time tool, right, has to have robust dashboarding capabilities and we’re no different, very configurable and a lot of flexibility here.
Mike Donovan: 00:12:55.343 So, yeah, so that’s - I think that all of the data that we just showed was very much related to - it all comes down to the real-time data that we’re able to collect about these buildings. And all that real-time data is going to flow through Influx and flow through our ingestions here. And so I wanted to get into how that kind of works. Some of the unique - starting off with, when I think about designing for this problem we have a few unique parts of the business that need to be satisfied, right? So support for various types of devices has always been something that we’ve had to do, right? And what I mean by this is we’ve iterated on the types of IoT devices that we will install in a building. It wasn’t always the case that we built our own hardware as a young company, right? You have to use off-the-shelf products. We were installed in over 800 buildings at this point. We’d run across so many different use cases. And so our platform has to kind of be agnostic to that fact, right? And we need to, again, just be focused on ingesting the data however we can. We also have to install and read from meters and sensors that exist in very hard to reach places. I mean, you can literally think about a 6-foot block of concrete with blocking any sort of Wi-Fi connection or any sort of wireless network connection between the meter and where you might have internet access or cellular access. So we’ve had to really solve that problem inside of the building. And then since our platform is a real-time energy monitoring platform, that’s one aspect of it, customers come to us on a daily, hourly, maybe a few times an hour, to get access to their real-time data. And a big differentiator is this access to very granular data, minute-by-minute data, so you can actually look at individual pieces of equipment and when they’re running and your building sequences. So this creates a need for data quality and just on-demand access.
Mike Donovan: 00:15:04.731 And so I’ll start with the hardware solution. It’s a critical component. If you think about the things that happen in a building, right, you have, I mentioned meters, right? So think of it like utility meters that might exist, that the utility company’s actually billing you off of in your house. It’s the same way. Then there’s the concept of sub-meters. And sub-meters, you can think about it like in your office space that your company occupies, there might be a meter that looks similar to a utility meter and it’s just collecting information about your energy usage, not the whole building’s. So there could be multiple sub-meters scattered throughout the building. Then there’s also IoT and smart devices. So there’s a lot of new developments coming out of the space management area around, I mentioned, humidity before, internal temperature sensors, noise, IAQ sensors, Indoor Air Quality sensors. All these things that tenants are actually demanding because they want a very clean and safe space to work in. And so we’ll also connect to that. Again, it’s just time-series data that we want to have access to. And so our devices can connect to that. And then, finally, a BMS system is still very prevalent. It’s in almost every building that we have access to. And where it’s applicable and where we can connect to it easily and fast, then we can also just speak to the BMS system and get access to a whole host of information.
Mike Donovan: 00:16:39.358 These level of devices all build what we refer to as a meshed network. It’s not Wi-Fi. It’s not Bluetooth. It’s actually a 900 megahertz proprietary network that can reach through the 6 feet big concrete slabs. And they all communicate eventually to an Aquicore Hub. And this is the cellular connected device that actually will send data to our cloud. When it goes to the cloud - I wanted to mention a little bit first about how we structure the data first. So when it’s in the cloud - and our platform is storing this sort of metadata about the building, the meters, and then the individual points. So for every building we get installed in there is a record of a building and its location, its size, the type of equipment that’s in there, the tenants that occupy it, any information we can collect about the building. That building is then - we will configure one to many meters or sensors within the building. Each meter gets a unique identifier. And it represents some type of utility. So there might be four electricity meters that we will configure in the platform, each with its own unique identifier, as well, as gas one, water one, and then maybe an IAQ sensor as well. And so all of that is configured and associated with the building. And each meter or sensor, then, gets one to many points. We call them points. They essentially represent a single input from this meter, right? There’s a lot of variability in the metering and sensor world. One specific meter might be able to send this data about multiple points of information, right, whether that’s amperage, voltage, electricity, like kW usage, the rate that water flows through the meter, the actual amount of gallons used. So there’s a whole host of metrics that can be sent to the platform from these points, from these meters. And we represent each of those different types as a single point.
Mike Donovan: 00:18:56.282 So with that data model in mind, now we can jump to how the data actually flows into the system. And I have a little diagram here. You can imagine our hub is sending data to our AWS cloud, which then routes it correctly. I mean, I think we have a pretty, par for the course, big data processing, data ingestion stream. It’s coming in through API gateway. And then we will send it - if I look at this last path here, I think it’s the easiest one. We’re going to send that to Kinesis so that we have our queuing mechanism. We’re immediately going to send it to - we’ve got a few consumers off that Kinesis stream. One of them is Firehose just for long-term storage in our data lake. It’s tremendous to have this in any sort of architecture because we’ve had to use it plenty of times for data re-planning and offline analysis. So having that structure there is great. And then we’ve got a Lambda that’s essentially reading off of the Kinesis and just doing the appropriate forwarding to our platform. And our platform is doing all the business logic around reading the point, the packet of data, which, essentially, each packet of data will represent one of those points like a kW value or a water gallons per minute value and associating it with the right metadata in our database which then stores it into Influx, right?
Mike Donovan: 00:20:24.747 We’ve also got another route here for Legacy devices. And the Series 3 are the AQ-Hub box that I showed. Legacy devices are really anything that’s not our own hardware. And like I mentioned we’ve had to support this over the years here and there’s still cases where we have to support this. It still goes through our API gateway but because of the structure and the way we receive the data it just doesn’t go through Kinesis which sucks but it is what it is. It’s still working pretty good. So once the data comes into Influx we store all database off of the point ID, right? So I will store - and they’ll be multiple tables or measurements set up for each utility type, right? So there will be an electricity measurement. And we’ll say that for this point ID we’ll have the kW value, the voltage value, the power factor value, basically, any piece of information that we have we’ll create tags for each of those data values. And then we’re also putting in information about the time zone and I’ll get to why we’ve done that. But that’s pretty much the structure of these Influx tables. And, again, we’ll do that for each measurement whether it’s gas, water, steam, all kinds of things.
Mike Donovan: 00:21:49.994 Yeah. So I’ll touch on the time zone. You’ll see a time zone problem here in the historical column. I’ll touch on that in a follow-up challenge. But it’s part of what we’ve had to iterate towards. Yeah. So that’s the main - that’s sort of the overall structure for how data will flow and get sorted into Influx. And now I just kind of wanted to touch on some challenges that we’ve come across during this - I mean, it’s been about two and a half, three years now that we’ve implemented and been using Influx pretty heavily and relying on it for our production environment. So we’ve learned a few things along the way that I wanted to share. To give an overview of the timeline a little bit, in 2013 we built the platform just using Postgres as the data store for the time series database. So this is obviously a great way to get out in the running right away without a lot of internal - with just people who knew Postgres and their job application. And that lasted for a pretty good while. We had to build - one of the use cases that we have to do, right, is support various aggregation levels of data. So the data will come in every minute but then I need to show 15-minute data to a user because that’s maybe what they want to see or hourly data to a user. You sum up all the data points or you take an average depending on the metric that you’re showing or a daily value.
Mike Donovan: 00:23:23.134 So we had to build an application actually because there’s nothing internal in Postgres that could do this. So we built the Scala application that would run and would just all the time crank out these aggregations. It’s pretty meaty. Then in 2016, we switched to Influx. So I came on board and I had experience with Influx before. And the team was thinking about switching to it. They knew they needed to switch to a time-series database. So we really went all in with Influx and began that migration from Postgres to Influx. I think it maybe took three months throughout the whole time of actually getting it out there and making sure it was battle-tested before we switched over. Because we were essentially changing the tires on the car while it was moving. But it was a very successful switchover. And now, we’ve been using it pretty happily since then. And we really just are constantly looking for performance improvements. And we grow with our customer base. So as more and more devices come online and we start to collect more and more data we’re constantly finding different bottlenecks in our platform and improving them.
Mike Donovan: 00:24:40.761 So now I want to get into some of these challenges. The first one to talk through is this Postgres to Influx migration, right? This was obviously one of the first big challenges we had to tackle. And Postgres was totally not able to handle this load and the Scala application was also just too tough to maintain and it wasn’t the right solution to the problem of data aggregation. One of the first things that we ended up doing was we would have the Aquicore platform and once we had Influx up and running - and we’re using InfluxDB Cloud for production - we would essentially duplicate the data across, sending it to Influx and Postgres. And we did that for, oh, it must’ve been a few months, right, just to make sure that we could spot-check the data and it looked good. And that was happening for every single write that would go in. Once we had some - we were far enough along in the implementation and we felt comfortable flipping this on for a few customers - we have a feature-flipping mechanism in the platform that allowed us to say for certain customers that were going to try to just serve the platform using InfluxData. And that allowed us obviously to incrementally add more risks, and risks, and risks as we were turning it on for more customers. And eventually, we had turned it on for all customers. And then after maybe a few weeks of just waiting, double-checking, triple-checking everything, seeing what kind of bugs came in, we were able to completely remove the Postgres instance as well as the Scala application running it and just replace it with Influx.
Mike Donovan: 00:26:26.472 The next challenge that we had to do was this data aggregation challenge, right? So I mentioned it before, right? We’re collecting minute data but customers need to see data at various intervals. And our intervals are minutes, 15 minutes, hour, and day. And depending on the type of unit that you might be calculating, whether it’s the average energy usage or whether it’s the total water usage, you have different math that you have to run on each one of those. And this was the Scala application. Again, it was too much of a load for that system. One of the big reasons why we wanted to move to Influx was because of continuous queries, right? We could essentially have something internal to the database that was doing this to the real-time data that was coming in. So just an example, continuous query here, nothing, I think, too crazy. But you can see that we will actually start to create separate measurements per aggregation. And then our query logic will know, depending on what the user wants to see, what requests that come in for, it will query the right measurement to look at that. But this didn’t solve - unfortunately, continuous queries, at the time, did not - we implemented this, right, three years ago - two, two and a half years ago - did not solve everything for us because the daily aggregation actually had an issue and it was one with time zones.
Mike Donovan: 00:27:50.889 So our platform, like I said, in over 800 buildings, that’s across the US, now including Hawaii, which is cool, and even some international ones and so if you want to - and we wanted to provide a correct daily aggregate value for all our units. And at the time, Influx didn’t have enough support for time zones to allow us to use continuous queries to do this. And so what we had to do instead was essentially just create a job internal to our application that would run, that was time zone aware, that would run for all the buildings in the right time zone and then essentially execute the Influx query to do what a continuous query would do instead. At this point, time zone support, I think, is much better. The train never stops, right, when you have a platform out for production. So we haven’t gone back yet and refactored how that works. But it’s been successful so it hasn’t been really that big of an issue.
Mike Donovan: 00:28:58.888 The other problem that comes up for us is historical data, right? So I talked a lot about real-time data and that’s the majority of - when you come to our platform and you’re looking at real-time data that our devices are sending. But it’s the real world, right, and things happen with the on-site devices. There might be gaps of data. There might be periods where the customer wants to upload historical data to compare their new data with a baseline. So we’ve had all kinds of a host of needs for this historical data uploading. So what we had to do - this is where continuous queries also didn’t work so well, right, because continuous queries are great for new data coming in that’s up to date, right, that’s fresh data. If I’m going to go back a month from now and upload one hour’s worth of data running the continuous query doesn’t really handle it. So what, instead, we’ve done is build essentially a historic data background process that will work as customers upload it, right, or when our devices send us historical data from going offline after a period of time.
Mike Donovan: 00:30:09.130 So how it works is essentially that the historical data comes in. We will submit that initial data sample to Influx, right? And then we will also put the data sample, essentially, in a Redis which will then - then the platform, also, is listening for to know if there’s any batch of historic data that it needs to aggregate. And then it will - if it finds that, it will manually execute, essentially, the continuous query, again, for that time period, that window of time that the historic data was submitted for. I highlight this because I think that it’s definitely something that we’ve always had to do, right, ever since using - even when we had Postgres or using Influx. And it’s definitely been something we’ve iterated up to scale because this gets used so much more than you would think when you’ve got a real-time platform but their customers never want gaps in their data and so this always comes up.
Mike Donovan: 00:31:13.706 The other thing that we’ve - one of the other challenges that we hit was a write performance issue. And, again, as our initial implementation of the - our application’s a Java client, right? So the initial implementation, it uses the Java-Influx client. And for some reason, we didn’t actually use the recommended approach. I highlighted the recommended approach back in the day, right? And it was all using synchronous writing. And so as soon as we switched to using the recommended approach of asynchronous writing and the batch processing, then all our write problems went away. We stopped receiving error - we’d get a lot of errors that would happen on the write to InfluxDB Cloud. And it ended up being something inefficient with the way that we were trying to manage the synchronous writes. And so, I think this is a great real world example of, I think, where the robustness of the platform is all based on the people and decisions that every developer makes on a daily basis. And sometimes you don’t make the right call at the right time and you never know. Some of the things, you don’t always know why. But as soon as we implemented the asynchronous recommended approach it went away. It was great. So follow the recommendations for sure.
Mike Donovan: 00:32:44.846 Another challenge that we hit was when trying to create some predictive analytics. So we had a feature - when the platform first started, we didn’t really have any predictive analytics. And a feature request came up to add something, right? And so I’m just showing you a picture here of - the darker line is the real-time usage of energy in the building. And then that predictive envelope is essentially what we predict your building should use based on historical data as well as external factors to the building like the weather outside as well as occupancy, right, occupancy changes in the building. So people wanted this in order to improve their operations and know if they’re doing well or not. So we initially tried to do this in Kapacitor but we weren’t able to get over the hump because of the need to include external data sources, right? So in Influx, like I showed you those measurement tables, we only store enough - we store for a single point what’s the value that we’re collecting? What’s the consumption? What’s the kW? What’s the gallons per minute? We’re not storing weather, building context information, that’s all in a different data set. And so we wanted to use Kapacitor to do that. But once we needed to pull in all this other information about weather and building characteristics, it didn’t end up meeting our needs and so we moved into this Java application instead and it’s working pretty good. Kapacitor seemed, definitely, like a robust tool it just didn’t meet this one need because of that need to include external data sources.
Mike Donovan: 00:34:36.926 I think this is the - yeah. So the last challenge I just want to highlight is the production monitoring challenge that we faced. As a platform that’s been around for a while, we’ve had various go-rounds of different application monitoring tools. And we always are a platform that tries to utilize the best third-party services we can so that can stick to our own business needs, what we need to do for our customers. And so we’ve kind of always had an issue with diagnosing production performance issues that might happen as it relates to the Influx database, right? Three years ago when we first did this, I think InfluxDB Cloud was still developing their monitoring capabilities. It is much better now for sure. There’s Grafana updates and the charts and data that we have access to is great. I think that what we’re always looking for is, “How can I get this information all in one place?” Right? I’d love to be able to see it all in Datadog or New Relic. So right now when production issues are happening, we’re still looking at a few different data sources that we’d like to update to only be one. And that kind of covers - yeah. That kind of covers the challenges that we faced. Overall, I think we just really loved using Influx. It’s been great. I think it’s scaled with us and allowed us to reach all our goals as a company. And some of these remaining challenges, I think, we’re just going to continually try to make it a little better.
Chris Churilo: 00:36:25.917 Awesome. Thank you. So before I ask you all my questions, which I always have a million of, I’m going to let the audience know that they can either post their questions in the chat or in the Q&A. And we’ll be here for, definitely, at least, 10 or so more minutes. So please feel free to post your questions. And if you really want to speak then I can also unmute you so just raise your hand. Okay. So can we go back to the slide on the data that you’re pulling in? I just wanted to talk a little bit about how you structured that in InfluxDB. Yeah. There we go. So the point ID, and time zone and, I guess, historical, those are all tags? Is that how you brought this in?
Mike Donovan: 00:37:13.229 Yeah. So the point ID, time zone, and historical are all tags. And the point ID represents - if I go back here, right? It represents this object in our database. The reason why we do that is so that we kind of decouple the building metadata from the real-time data and that allows us - because a meter that was once representing electricity or was once representing a certain tenant will change over time but we don’t want our data in Influx to be dependent on that. We want to be able to adjust how we visualize the data.
Chris Churilo: 00:37:52.979 So then how do you - so when tenants do change over time or - so is that information stored in your Redis database or how do you make those connections?
Mike Donovan: 00:38:06.034 So this whole database here, this is essentially in the Postgres instance. And so that would be all within the metadata of the building database which is our Postgres instance, whether it’s tenants, whether it’s equipment, whether it’s information about the building.
Chris Churilo: 00:38:23.664 That makes sense. Okay. Cool. And then the other thing is you mentioned that the data’s coming in at minute intervals. Are they coming in at tinier intervals? I was thinking more like things like gas. Sometimes you can’t really see any changes in the data unless it’s in smaller intervals.
Mike Donovan: 00:38:47.515 Yeah. Right now, our lowest interval is minute. And this is really a question of customer need and what kind of value we can provide if we’re talking about going at a lower interval or more frequent interval rather. When you get into the equipment monitoring world, that’s when you really want to know about sub-minute because you want to know very minute changes in the equipment that you can run an analysis on. But, yeah, minute is just fine for all our customers. Now, I mean, some of them don’t even need minute data, right? They’re really just looking for 15-minute data so that they can - because that’s actually what’s related to how they are billed for energy, right? The utility company will only ever know about 15-minute data at the most. And so it’s all about relating this data to how much it’s actually costing you.
Chris Churilo: 00:39:45.394 That makes total sense. I loved how you guys had to overcome the challenge of concrete walls. I think so many of us don’t think about those kinds of things. You just assume that, “Oh, hey, Wi-Fi or - you’re assuming it’s just going to work but -
Mike Donovan: 00:40:00.463 Yeah. It’s a big deal in the commercial real estate world. And I think we - I mentioned the partnership and marketplace. We talked to a lot of commercial real estate companies. There’s a lot to learn from our solution here because we’ve had to try it all and really kind of settled on this approach because it’s the fastest and can handle all the variability of the buildings, right? No one building is the same.
Chris Churilo: 00:40:24.994 And how much energy do your sensors require? Because you also mentioned they’re in really hard-to-reach places so I imagine that must be a concern too?
Mike Donovan: 00:40:37.247 Yeah. So it runs off a normal 12-volt plug, right? So a lot of times you just need to plug it into a nearby outlet. But it can also be powered off of the meter itself, right? So we actually will sometimes just connect to the actual meter that we’re - we’ll connect our bridge to the actual sub-meter and then pull off a little bit of electricity just to power it.
Chris Churilo: 00:41:02.651 Okay. Excellent. We have a question that just came in. Are your predictive analytic savings in Influx or generated on demand?
Mike Donovan: 00:41:14.793 Those are actually stored in Influx. We actually will calculate. So how it ended up working - this is why we wanted to do it in Kapacitor at first was because, yeah, just keeping it all in Influx would’ve been great. We, actually, in the Java application, we’ll query Influx for all the data, query all the building context information about weather and occupancy. And then it will store it in a separate measurement in Influx just for predictive analytics.
Chris Churilo: 00:41:50.990 Let’s see. Another question just popped in. Is cellular the only connection medium supported or can the AQ hub be hardwired to an IP network for transferring data to the cloud?
Mike Donovan: 00:42:03.093 We prefer to always use cellular, not to say that it never happened where we’ve had to use the building internet. But I will just say that when data doesn’t show up in the platform nobody wants to hear that it’s a networking problem. They blame you. They blame us, right? Because I can’t say, “Well, go talk to your IT department.” And so we really like to be in control of the full solution so that we can - it’s obviously more responsibility but it actually works out to be better because the building internet is usually not very reliable.
Chris Churilo: 00:42:41.687 John, hopefully, that helps you. So we have another question. The question is, can you tell us what was involved in the decision-making process for choosing InfluxDB?
Mike Donovan: 00:42:54.955 Yeah. Well, so maybe if I go back now three years ago, I think that we definitely had to get into a time-series database. That was, I think, pretty clear to us. And I had been tracking InfluxDB for a long time and using it at previous companies. I think the thing, when I look at InfluxDB versus some of the other ones, I’m always looking for - and whenever we choose these tools I’m looking for something that’s been around for a little while and has a really good community behind it because I’m choosing a technology for the long haul. The feature sets are sometimes often similar for our needs, right? We don’t necessarily need all the rest of the Tick Stack. So I was really just impressed by the community and the consistent updates to InfluxDB Cloud. All those things kind of allowed us to choose Influx.
Chris Churilo: 00:43:50.074 And then, I think you use the hosted version too, right?
Mike Donovan: 00:43:54.625 Yeah. So in our InfluxDB Cloud is what we run our production environment in. And then in our QA and lower environments, we will run our own managed version on EC2 instance. It’s probably more for our own learning than anything else.
Chris Churilo: 00:44:15.192 Yeah. No. I mean, you should. And everyone on this call, you should definitely do that. We have a lot of people that will use either enterprise or cloud for their production environments and then for their QA environments, various test environments, they’ll use the open source version. All right. We have a couple more questions. Arn asks: How do you handle metadata? Do you have a separate database for that?
Mike Donovan: 00:44:43.190 Yes. So Influx will only store data that looks like this, right? So it’ll just have a point identifier. Where was that? And then all of this data is stored in Postgres. So there’s a table for buildings. There’s a table for all the meters. There’s a table for all the points. And there’s relationships built between them all. And so when I go to query like, “What’s the usage on this meter,” well, I go and get all the points under that meter and I basically build a query in Influx that selects all the right points and then aggregates the data.
Chris Churilo: 00:45:25.496 Arn, let us know if that helped with your question. We have another question. Can you expand more on your data quality control? Is all that data saved in Influx and tagged as bad or just left out?
Mike Donovan: 00:45:38.185 So we don’t necessarily tag it in Influx. The only thing I actually didn’t mention in here is another tag is whether or not the data is interpolated. So that’s maybe the only measure of quality that will be stored in Influx. And that’s essentially like - like I said, we’ve seen it all. So there are definitely times where a meter will report for a few minutes but we still want to give - we don’t want the user to have a bad experience so we’ll interpolate the missing data, only up to so much time, right? It’s not [inaudible] crazy but we will put that in there. As far as other data quality efforts, there happening external to Influx and to this overall data processing. And that’s a lot of where our data science comes in and sort of doing that analysis outside and then coming in because there’s a whole support process around that, right? I mean, we literally - like I said, 800 buildings. I don’t know. Last I counted it was 6,000 devices reporting to us. Maybe it’s at like 10,000 now, as it was a little while ago. So there’s a whole support process around doing that analysis and fixing it. Fixing it usually results in using that historical data mechanism that I referred to.
Chris Churilo: 00:46:58.411 Let’s see, another question. In terms of your outcomes, do you feel InfluxDB Cloud has enhanced your competitive advantage in any way?
Mike Donovan: 00:47:09.590 Well, for sure, right? I mean, I think that the name of the game is all about how fast can we build high-quality software in production? And I need to not be worried about - I don’t want to be worried about building my own time-series database. I don’t want to even be worried about managing my own time-series database. I just want to be worried about predictive analytics and the right craft and mobile app and those types of things. So I think that Influx - and once we moved to Influx and got really good at it, it’s allowed us to scale the amount of data and varying types of data we are able to collect much faster as well as by using InfluxDB Cloud we’ve just got that whole responsibility lifted off our shoulders about just managing the ever-growing database that we have.
Chris Churilo: 00:48:00.714 So you showed us a couple screenshots of a smartphone app and then also a web app. So I’m assuming that you’re querying InfluxDB to populate those graphs?
Mike Donovan: 00:48:18.339 Correct. So-
Chris Churilo: 00:48:19.269 And maybe you can share with the audience what frameworks you used to build those dashboards?
Mike Donovan: 00:48:28.595 Sure. So the web application is all built on - our main backend is a Java application running in Heroku right now. We’re slowly sort of breaking apart that application into various microservices that actually run in Lambda. We’re big proponents of serverless. And, yeah, I just haven’t had a problem that a [inaudible] script application in Lambda can’t fix currently when I’m building our APIs. So the backend’s all doing that. And that’s the backend, the thing that’s integrating with Influx and bringing the databases on API calls. The frontend application on the web app is an angular application. And then the mobile app is actually a React Native application. And that’s across iOS and Android, obviously.
Chris Churilo: 00:49:16.621 Well, they both look really good. They’re very clear. I mean, even with this small screenshot, I know exactly what’s going on. So I have one last question and then I’ll let everyone else ask some questions. So when you talked about the predictive analytics, I thought it was interesting that weather can seem like an obvious thing to add to the mix. The occupancy numbers that changes, that was a surprise to me. I imagine there must be lots of other data sources that you can also consider. And how do you go about determining what other data sources you might want to bring into the mix to try to determine the predictions?
Mike Donovan: 00:50:00.948 Yeah. I think this is where industry expertise comes in. So at Aquicore, we’re very much a technology company with a large product organization. But we’ve also got in-house experts in building systems and for all the different - I mean, there’s a whole industry around that building engineer persona, right? We want to work with the best of those people so that we understand how equipment runs, what impacts the building so that we can determine what to include, right? So whether that’s internal expertise or working with some of the best of the best out there to know what to factor in. So knowing that we need to factor in occupancy and how variable it is, is known because of being able to work with the best building engineers out there. Other things that always come to mind are the seasonal variabilities, right, knowing that there’s a big difference between winter and summer and the operations of a building and what causes that change, right? That’s all from understanding how HVAC systems work in these large commercial real estate offices as well as things like knowing that the building is running a completely new system. They just ran through a capital project to install a completely new HVAC system, which completely changes the operations of the building. Keeping up to date with that information and knowing how to factor that in is super important and [inaudible] based on [inaudible].
Chris Churilo: 00:51:36.792 So do these building engineers - I mean, they must be pretty excited to have all this data at their fingertips. Do they get inspired and start asking you guys for more features or more data sources coming in?
Mike Donovan: 00:51:50.747 Yeah. I’ve got a backlog of feature requests that seems to constantly grow. At Aquicore, I think there’s no shortage of vision and no shortage of [inaudible] that we’re trying to solve for the industry. One thing that we track a lot, as a company, is the engagement that we have on our platform. We’re an enterprise application, right? People buy us for their jobs and it’s a whole company purchasing us across all their buildings. And it’s kind of rare, I think, to see an enterprise application tracking engagement. We’re upwards of 70% MAU. And we’re very proud of that number because we think it relates to the building engineers, the property managers, all those people really getting value out of this data and the ways that we’re able to bring the insights to the market. And it also results in lots of feature requests, lots of different data that we want to collect. Just recently we implemented a humidity sensor and a temperature sensor in a data center, right? This is actually a new trend in real estate that more people are renting out data center space. And so monitoring the indoor of - these property management companies want to monitor the indoor temperature and humidity of these data centers to ensure optimal operations. So working with customers to solve those needs is always something we’re doing.
Chris Churilo: 00:53:11.157 Of course. Of course. Every time I hear about these use cases I start to realize how little I know about what is actually happening in the world around me. This was really cool. I learned a ton. I’m sure our audience did as well. And to the audience, if you have any other questions after this webinar feel free to shoot me an email and I’ll forward your questions to Mike. This happens all the time. Sometimes we walk away and we start to realize, “Oh
, I should’ve asked him about this or that.” And don’t worry. We can always still get you in contact with him and hopefully help you build your applications as well. Mike, any last words about - maybe some advice about building a SaaS like you did and pulling in all those disparate data sources?
Mike Donovan: 00:54:05.319 I think the best thing is to always think about the right iteration path when you’re doing these types of undertakings, right? So choosing and just trying to stick to what your core business is trying to do, right? I see a lot of teams and a lot of platforms where it might be a little more over- engineered and they’re not trusting of third-party tools out there enough and they’re sort of rebuilding the wheel. So you always want to be mindful to not reinvent the wheel, I think, and to always align what you’re doing in your architecture with the actual business use case and business value you’re trying to deliver. And that’s, I think, how you’ll always make the right decision along the way and not over-engineer your solution and then essentially you take away from building other features that your company needs.
Chris Churilo: 00:54:56.820 Very good. I’m sure you are also hiring? So where are most of your engineers based out of?
Mike Donovan: 00:55:05.491 So we’re all based out of - the main headquarters is in Washington, DC. We also have an office in Florida, near Miami. And then we’ve got a few remote employees as well. So we have an actually open position. I don’t know if it’s up on the website yet. But we have an open position for a full stack engineer. I’m always on the hunt for great engineers who really care about the user, the technology, and the business value that they’re trying to create. And so if you’re totally someone who wants to move quickly and be in this sort of innovative industry right now shoot me an email or just go to careersataquicore.com.
Chris Churilo: 00:55:49.377 Excellent. Well, thank you so much for your time today, Mike. This was absolutely excellent. And we will chat again soon. Thanks, everybody.
Mike Donovan: 00:55:58.539 Thank you.
[/et_pb_toggle]
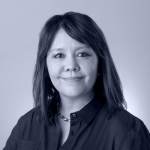
Chris Churilo
VP of Marketing, InfluxData
Chris Churilo helps businesses build passionate teams that market to the developer audience by building content and programs that are engaging, and drives demand for the product that results in revenue. As the Vice President of Marketing at InfluxData, she has oversight of day-to-day marketing operations to ensure that growth marketing, comms, digital, partner and customer marketing as well as demand gen all function as a tight cohesive unit aligned with the company’s goals. For Chris, sharing the cool projects that developers build with InfluxDB is the most rewarding part of the role. Outside of the office, Chris enjoys singing to her dog, kick-starting her nonprofit, and downwinding on her paddleboard across the bay.